2018-2019 Long-Term Future Fund Grantees: How did they do?
By NunoSempere @ 2021-06-16T17:31 (+194)
Introduction
At the suggestion of Ozzie Gooen, I looked at publicly available information around past LTF grantees. We've been investigating the potential to have more evaluations of EA projects, and the LTFF grantees seemed to represent some of the best examples, as they passed a fairly high bar and were cleanly delimited.
For this project, I personally investigated each proposal without consulting many others. This work was clearly limited by not reaching out to others directly, but requesting external involvement would have increased costs significantly. We were also partially interested in finding how much we could figure out with this limitation.
Background
During its first two rounds (round 1, round 2) of the LTF fund, under the leadership of Nick Beckstead, grants went mostly to established organizations, and didn’t have informative write-ups.
The next few rounds, under the leadership of Habryka et. al., have more informative write-ups, and a higher volume of grants, which are generally more speculative. At the time, some of the grants were scathingly criticised in the comments. The LTF at this point feels like a different, more active beast than under Nick Beckstead. I evaluated its grants from the November 2018 and April 2019 rounds, meaning that the grantees have had at least two years to produce some legible output. Commenters pointed out that the 2018 LTFF is pretty different from the 2021 LTFF, so it’s not clear how much to generalize from the projects reviewed in this post.
Despite the trend towards longer writeups, the reasoning for some of these grants is sometimes opaque to me, or the grant makers sometimes have more information than I do, and choose not to publish it.
Summary
By outcome
Flag | Number of grants | Funding ($) |
More successful than expected ![]() | 6 (26%) | $ 178,500 (22%) |
As successful as expected ![]()
| 5 (22%) | $ 147,250 (18%) |
Not as successful as hoped for ![]() | 3 (13%) | $ 80,000 (10%) |
Not successful ![]() | 3 (13%) | $ 110,000 (13%) |
Very little information ![]() | 6 (26%) | $ 287,900 (36%) |
Total | 23 | $ 803,650 |
Not included in the totals or in the percentages are 5 grants worth a total of $195,000 which I tagged didn’t evaluate because of a perceived conflict of interest.
Method
I conducted a brief Google, LessWrong and EA forum search of each grantee, and attempted to draw conclusions from the search. However, quite a large fraction of grantees don't have much of an internet presence, so it is difficult to see whether the fact that nothing is findable under a quick search is because nothing was produced, or because nothing was posted online. Overall, one could spend a lot of time with an evaluation. I decided to not do that, and go for an “80% of value in 20% of the time”-type evaluation.
Grantee evaluation examples
A private version of this document goes by grantees one by one, and outlines what public or semi-public information there is about each grant, what my assessment of the grant’s success is, and why. I did not evaluate the grants where I had personal information which people gave me in a context in which the possibility of future evaluation wasn't at play. I shared it with some current LTFF fund members, and some reported finding it at least somewhat useful.
However, I don’t intend to make that version public, because I imagine that some people will perceive evaluations as unwelcome, unfair, stressful, an infringement of their desire to be left alone, etc. Researchers who didn’t produce an output despite getting a grant might feel bad about it, and a public negative review might make them feel worse, or have other people treat them poorly. This seems undesirable because I imagine that most grantees were taking risky bets with a high expected value, even if they failed in the end, as opposed to being malicious in some way. Additionally, my evaluations are fairly speculative, and a wrong evaluation might be disproportionately harmful to the person the mistake is about.
Nonetheless, it’s possible that sharing this publicly would produce positive externalities (e.g., the broader EA community gets better models of the LTFF in 2018/2019). I've created a question on the EA Forum here to ask about people's perspectives on this tradeoff.
Still, below are two examples of the type of evaluation I did. The first one states fairly uncontroversial figures which are easy to find about a fairly public figure. The second is about a private individual whom I’m explicitly asking for permission, and includes some judgment calls.
Robert Miles ($39,000)
Short description: Producing video content on AI alignment
Publicly available information:
- At the time of the grant, "the videos on his Youtube channel picked up an average of ~20k views." This has increased much more in recent times; the 9 videos made in the two years since the grant have an average of 107k views. Assuming a grant a year, this comes to 7cts/view, which seems very, very cheap.
- Robert Miles gets ~$12k/year on Patreon, and I imagine some ad revenue, so the Shapley value of the LTF is somewhat lower than one might naïvely imagine. I don't think this is much of a concern, though, something like $30cts/view is still pretty cheap.
Shape of the update:
- Video impressions increased a lot, but the defining factor is probably whether watching Robert Miles's videos produces researchers, donors, or has some broader societal change. This is still uncertain, and difficult to measure.
- Overall result: More successful than expected.
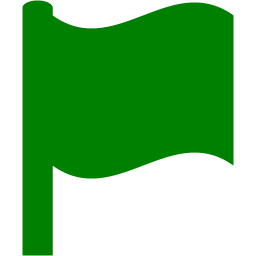
Vyacheslav Matyuhin ($50,000)
Short description: An offline community hub for rationalists and EAs in Moscow. "There’s a gap between the “monthly meetup” EA communities and the larger (and significantly more productive/important) communities. That gap is hard to close for many reasons."
Publicly available information:
- Rationalist Community Hub in Moscow: 3 Years Retrospective
- LW user: berekuk
- I reached out to Vyacheslav, and he answered here in some detail.
- Kocherga continues to exist, but its webpage is in Russian.
Shape of the update:
- This is difficult to evaluate for a non-Russian. Per Vyacheslav Matyuhin’s self report, the grant kept Kocherga alive until 2020, and it then attained financial independence and continued activities online throughout 2020/2021 (“Kocherga is still going — we moved everything to Zoom a year ago, we have 8-12 events and ~100-120 registrations per week, and we did some paid rationality trainings online too.”)
- The main organizer was feeling somewhat burnt out, but is now feeling more optimistic about Kocherga’s impact from an EA perspective.
- Overall, the case for Kocherga’s impact seems pretty similar to the one three years ago.
- Overall result: As successful as expected
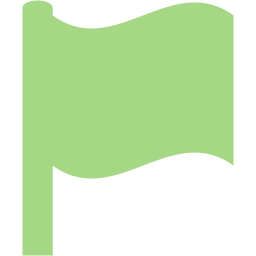
Observations
I don’t really have any grand conclusions, so here are some atomic observations instead:
- There is a part of me which finds the outcome (a 30 to 40% success rate) intuitively disappointing. However, it may suggest that the LTF was taking the right amount of risk per a hits-based-giving approach.
- Perhaps as expected, grantees with past experience doing the thing they applied to seem to have done significantly better. This suggests a model where people pursue some direction for free, show they can do it, and later get paid for it. However, that model has tradeoffs.
- I find it surprising that many grantees have little information about them on the internet. Initially, I was inclined to believe that their projects were thus not successful, and to update negatively on not funding information of success. However, after following up on some of the grants, it seems that some produced illegible, unpublished or merely hard to find outputs, as opposed to no outputs.
- I was surprised that a similar project hadn’t already been carried out (for instance, by a large donor to the LTFF, or by the LTFF itself.)
- I can imagine setups where this kind of evaluation is streamlined and automatized, for instance using the reviews which grantees send to CEA.
- I know that I am missing inside information, which makes me more uncertain about conclusions.
Larks @ 2021-06-16T18:56 (+54)
Thanks very much for doing this useful work! This seems like the sort of project that should definitely exist, but basically inexplicably fails to come about until some random person decides to do it.
I hate to give you more work after you have perhaps already put in more time on this than everyone else combined, but I can think of two things that might make this even more useful:
- Conclusions about the types of grants that performed well or badly, e.g.
- Did they tend to be larger organisations or individuals?
- Were they more speculative or have a concrete roadmap?
- Were they research based, skill acquiring or community organising?
- Comparisons to other granters.
- A 30-40% success ratio isn't that informative if readers don't have a strong sense of what success means to you (because readers can't see what you've classified as a failure), so we don't know how good or bad this is for the LTFF. But if we could compare it to the other EA Funds, or to OpenPhil or GiveWell or the SFF, that could give useful context and help people decide which one to donate to.
NunoSempere @ 2021-06-17T16:52 (+21)
Thanks! To answer the questions under the first bullet point:
- Individuals performed better than organizations, but there weren't that many organizations.
- Individuals pursuing research directions mostly did legibly well, and the ones who didn't do legibly well seem like they had less of a well-defined plan, as one might expect.
- But some people with less defined directions also seem like they did well.
- Also note that maybe I'm rating research directions which didn't succeeded as less well defined.
- I don't actually have access to the applications, just to the grant blurbs and rationales
- Grants to organize conferences and workshops generally delivered, and I imagine that they generally had more concrete roadmaps
- There was only one upskilling grant.
In general, I think that the algorithm of looking at past similar grants and see if they succeed might be decently predictive for new grants, but that maybe isn't captured by the distinctions above.
Ozzie Gooen @ 2021-06-17T00:25 (+12)
Some quick thoughts:
- This work was meant to be built on. Hopefully there will be more similar work going forward (by both us and others), so much of the purpose here is to lay some foundation and help dip our toes into this sort of evaluation. (It can be controversial or harmful, so we're going slowly). As such, ideas for improvement are most welcome!
- I've read the larger review. I'd note that there were few groups that really surprised me. If you go through the list of grantees, and think about what you know of each candidate, I'd bet you can get a roughly similar sense. (This is true for those who read LW/EA Forum frequently). One of the main purposes of this sort of work is to either find or try and fail to find big surprises. From my perspective, I think that groups/individuals who had previously provided value (different from seeming prestigious, to be clear), went on to provide more value, and those that hadn't didn't do as well.
- This work wasn't done with the particular intention of helping to decide between EA Funds. We have been doing some other investigation here, somewhat accidentally (I've been assisting a donor lottery winner to decide). It's a good thing to keep in mind going forward.
- It would be great to later have measures of total impact for longtermism. We don't have strong measures now, but would love to help develop these (or further encourage others to).
Jonas Vollmer @ 2021-06-18T16:38 (+46)
Thanks a lot for doing this evaluation! I haven't read it in full yet, but I would like to encourage more people to review and critique EA Funds. As the EA Funds ED, I really appreciate it if others take the time to engage with our work and help us improve.
velutvulpes @ 2021-06-19T21:40 (+16)
Is there a useful way to financially incentivise these sort of independent evaluations? Seems like a potential good use of fund money
Jonas Vollmer @ 2021-06-21T08:07 (+13)
I'd be pretty excited about financially incentivizing people to do more such evaluations. Not sure how to set the incentives optimally, though – I really want to avoid any incentives that make it more likely that people say what we want to hear (or that lead others to think that this is what happened, even when didn't), but I also care a lot about such evaluations being high-quality and and having sufficient depth, so don't want to hand out money for any kind of evaluation.
Perhaps one way is to pay $2,000 for any evaluation or review that receives >120 Karma on the EA Forum (periodically adjusted for Karma inflation), regardless of what it finds? Of course, this is somewhat gameable, but perhaps it's good enough.
Max_Daniel @ 2021-06-17T00:02 (+43)
- There is a part of me which finds the outcome (a 30 to 40% success rate) intuitively disappointing. However, it may suggest that the LTF was taking the right amount of risk per a hits-based-giving approach.
FWIW, my immediate reaction had been exactly the opposite: "wow, the fact that this skews so positive means the LTFF isn't risk-seeking enough". But I don't know if I'd stand by that assessment after thinking about it for another hour.
NunoSempere @ 2021-06-17T09:01 (+21)
Yes, for me updating upwards on total success on a lower percentage success rate seems intuitively fairly weird. I'm not saying it's wrong, it's that I have to stop and think about it/use my system 2.
In particular, you have to have a prior distribution such that more valuable opportunities have a lower success rate. But then you have to have a bag of opportunities such that the worse they do, the more you get excited.
Now, I think this happens if you have a bag with "golden tickets", "sure things", and "duds". Then not doing well would make you more excited if "sure things" were much less valuable than the weighted average of "duds" and "golden tickets".
But to get that, I think you'd have to have "golden tickets" be a binary thing. But in practice, take something like GovAI. It seems like its theory of impact is robust enough that I would expect to see a long tail of impact or impact proxies, rather than a binary success/not success, instead of a lottery ticket shaped impact. Say that I'd expect their impact distribution to be a power law: In that case, I would not get more excited if I saw them fail again and again. Conversely, if I do see them getting some successes, I would update upwards on the mean and the standard deviation of the power law distribution from which their impact is drawn.
Max_Daniel @ 2021-06-17T13:25 (+10)
Thanks, that makes sense.
- I agree with everything you say about the GovAI example (and more broadly your last paragraph).
- I do think my system 1 seems to work a bit differently since I can imagine some situations in which I would find it intuitive to update upwards on total success based on a lower 'success rate' - though it would depend on the definition of the success rate. I can also tell some system-2 stories, but I don't think they are conclusive.
- E.g., I worry that a large fraction of outcomes with "impact at least x" might reflect a selection process that is too biased toward things that look typical or like sufficiently safe bets - thereby effectively sampling from a truncated range of a heavy-tailed distribution. The so selected grants might then have an expected value of n times the median of the full distribution, with depending on what share of outliers you systematically miss and how good your selection power within the truncated distribution is - and if the distribution is very heavy-tailed this can easily be less than the mean of the full distribution, i.e., might fail to even beat the benchmark of grant decisions by lottery.
- (Tbc, in fact I think it's implausible that LTFF or EAIF decisions are worse than decisions by lottery, at least if we imagine a lottery across all applications including desk rejects.)
- Similarly, suppose I have a prior impact distribution that makes me expect that (made-up number) 80% of the total ex-post impact will be from 20% of all grants. Suppose further I then do an ex-post evaluation that makes me think that, actually the top 20% of grants only account for 50% of the total value. There are then different updates I can make (and how much I should make which of these depends on other context and the exact parameters):
- The ex-post impact distribution is less heavy-tailed than I thought.
- The grant selection process is systematically missing outliers.
- The outcome was simply bad luck (which in a sense wouldn't be that surprising since the empirical average is such an unstable estimate of the true mean of a highly heavy-tailed distribution). This could suggest that it would be valuable to find ways to increase the sample size, e.g., by spending less time on evaluating marginal grants and instead spending time on increasing the number of good applications.
- E.g., I worry that a large fraction of outcomes with "impact at least x" might reflect a selection process that is too biased toward things that look typical or like sufficiently safe bets - thereby effectively sampling from a truncated range of a heavy-tailed distribution. The so selected grants might then have an expected value of n times the median of the full distribution, with depending on what share of outliers you systematically miss and how good your selection power within the truncated distribution is - and if the distribution is very heavy-tailed this can easily be less than the mean of the full distribution, i.e., might fail to even beat the benchmark of grant decisions by lottery.
- However, I think that in this case my sys 1 probably "misfired" because the fraction of grants that performed better or worse than expected doesn't seem to have a straightforward implication within the kind of models mentioned in this or your comment.
NunoSempere @ 2021-06-19T07:49 (+4)
Makes sense. In particular, noticing that grants are all particularly legible might lead you update in the direction of a truncated distribution like you consider. So far, the LTFF seems like it has maybe moved a bit in the direction of more legibility, but not that much.
Linch @ 2021-06-17T18:40 (+4)
Conversely, if I do see them getting some successes, I would update upwards on the mean and the standard deviation of the power law distribution from which their impact is drawn.
It makes sense to update upwards on the mean, but why would you update on the standard deviation from n of 1? (I might be missing something obvious)
NunoSempere @ 2021-06-17T21:03 (+5)
Well, because a success can be caused by a process who has a high mean, but also by a process which has a lower mean and a higher standard deviation. So for example, if you learn that someone has beaten Magnus Carlsen, it could be someone in the top 10, like Caruana, or it could be someone like Ivanchuk, who has a reputation as an "unreliable genius" and is currently number 56, but who, when he has good days, has extremely good days.
NunoSempere @ 2021-06-17T21:13 (+11)
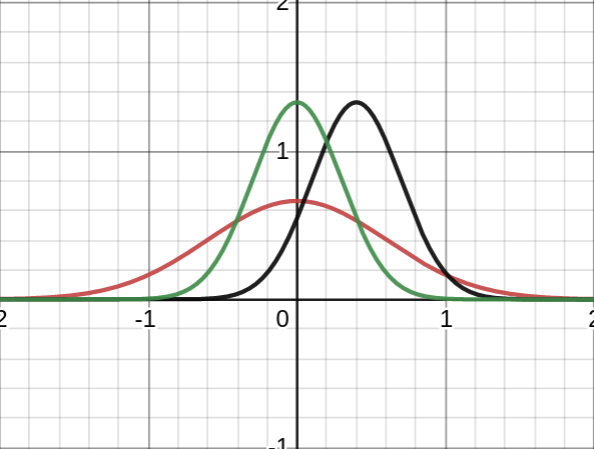
Suppose you give initial probability to all three normals. Then you sample an event, and its value is 1. Then you update against the green distribution, and in favor of the red and black distributions. The black distribution has a higher mean, but the red one has a higher standard deviation.
Linch @ 2021-06-17T23:49 (+2)
Thanks, I understand what you mean now!
jacobjacob @ 2021-06-17T23:49 (+13)
To really make this update, I'd want some more bins than the ones Nuno provide. That is, there could be an "extremely more successful than expected" bin; and all that matters is whether you manage to get any grant in that bin.
(For example, I think Roam got a grant in 2018-2019, and they might fall in that bin, though I haven't thought a lot about it.)
NunoSempere @ 2021-06-19T07:51 (+4)
I would also want more bins than the ones I provide, i.e., not considering the total value is probably one of the parts I like less about this post.
Max_Daniel @ 2021-06-18T08:50 (+4)
Yeah I agree that info on how much absolute impact each grant seems to have had would be more relevant for making such updates. (Though of course absolute impact is very hard to estimate.)
Strictly speaking the info in the OP is consistent with "99% of all impact came from one grant", and it could even be one of the "Not as successful as hoped for". (Though taking into account all context/info I would guess that the highest-impact grants would be in the bucket "More successful than expected".) And if that was the case one shouldn't make any updates that would be motivated by "this looks less heavy-tailed than I expected".
Daniel_Eth @ 2021-06-21T04:12 (+23)
"There is a part of me which finds the outcome (a 30 to 40% success rate) intuitively disappointing"
Not only do I somewhat disagree with this conclusion, but I don't think this is the right way to frame it. If we discard the "Very little information" group, then there's basically a three-way tie between "surprisingly successful", "unsurprisingly successful", and "surprisingly unsuccessful". If a similar amount of grants are surprisingly successful and surprisingly unsuccessful, the main takeaway to me is good calibration about how successful funded grants are likely to be.
MichaelA @ 2021-06-16T20:05 (+16)
(In case any readers are unfamiliar with Shapley values - mentioned in the Robert Miles section of this post - Nuno previously wrote an interesting post on the concept.)
MichaelA @ 2021-06-16T20:03 (+12)
Thanks, I found this interesting.
But I also found the outcomes hard to interpret - when you say "than expected", are you referring to what it seems the LTFF expected when they made the grant, what you think you would've expected at the time the grant was made, what you expect from EA/longtermist donations in general, or something else?
NunoSempere @ 2021-06-17T17:02 (+2)
are you referring to what it seems the LTFF expected when they made the grant, what you think you would've expected at the time the grant was made, what you expect from EA/longtermist donations in general, or something else
Yes, that's tricky. The problem I have here is that different grants are in different domains and take different amounts. Ideally I'd have something like "utilons per dollar/other resources" but that's impractical. Instead, I judge a grant in its own terms: Did it achieve the purpose in the grants rationale? or something similarly valuable in case there was a change of plan?
jacobjacob @ 2021-06-17T23:51 (+5)
I was a grantee in 2019 and consent to having my evaluation shared publicly, if Nuno wants to post it.
Linch @ 2021-06-18T00:15 (+4)
I assumed that you'd be one of the people in the
5 grants worth a total of $195,000 which I [NunoSempere] tagged didn’t evaluate because of a perceived conflict of interest
bucket, fwiw.