Update to Samotsvety AGI timelines
By Misha_Yagudin, JonathanMann, NunoSempere @ 2023-01-24T04:27 (+120)
Previously: Samotsvety's AI risk forecasts.
Our colleagues at Epoch recently asked us to update our AI timelines estimate for their upcoming literature review on TAI timelines. We met on 2023-01-21 to discuss our predictions about when advanced AI systems will arrive.
Forecasts:
Definition of AGI
We used the following definition to determine the “moment at which AGI is considered to have arrived,” building on this Metaculus question:
The moment that a system capable of passing the adversarial Turing test against a top-5%[1] human who has access to experts on various topics is developed.
More concretely:
A Turing test is said to be “adversarial” if the human judges make a good-faith attempt to unmask the AI as an impostor, and the human confederates make a good-faith attempt to demonstrate that they are humans.
An AI is said to “pass” a Turing test if at least half of judges rated the AI as more human than at least third of the human confederates.
This definition of AGI is not unproblematic, e.g., it’s possible that AGI could be unmasked long after its economic value and capabilities are very high. We chose to use an imperfect definition and indicated to forecasters that they should interpret the definition not “as is” but “in spirit” to avoid annoying edge cases.
Individual forecasts
P(AGI by 2030) | P(AGI by 2050) | P(AGI by 2100) | P(AGI by this year) = 10% | P(AGI by this year) = 50% | P(AGI by this year) = 90% | |
F1 | 0.39 | 0.75 | 0.78 | 2028 | 2034 | N/A[2] |
F3 | 0.28 | 0.7 | 0.87 | 2027 | 2039 | 2120 |
F4 | 0.26 | 0.58 | 0.93 | 2025 | 2039 | 2088 |
F5 | 0.35 | 0.73 | 0.91 | 2025 | 2037 | 2075 |
F6 | 0.4 | 0.65 | 0.8 | 2025 | 2035 | N/A[3] |
F7 | 0.33 | 0.65 | 0.8 | 2026 | 2037 | 2250 |
F8 | 0.2 | 0.5 | 0.7 | 2026 | 2050 | 2200 |
F9 | 0.23 | 0.44 | 0.67 | 2026 | 2060 | 2250 |
Aggregate
P(AGI by 2030)[4] | P(AGI by 2050) | P(AGI by 2100) | P(AGI by this year) = 10% | P(AGI by this year) = 50% | P(AGI by this year) = 90% | |
mean: | 0.31 | 0.63 | 0.81 | 2026 | 2041 | 2164 |
stdev: | 0.07 | 0.11 | 0.09 | 1.07 | 8.99 | 79.65 |
50% CI: | [0.26, 0.35] | [0.55, 0.70] | [0.74, 0.87] | [2025.3, 2026.7] | [2035, 2047] | [2110, 2218] |
80% CI: | [0.21, 0.40] | [0.48, 0.77] | [0.69, 0.93] | [2024.6, 2027.4] | [2030, 2053] | [2062, 2266] |
95% CI[5]: | [0.16, 0.45] | [0.41, 0.84] | [0.62, 0.99] | [2023.9, 2028.1] | [2024, 2059] | [2008, 2320] |
geomean: | 0.30 | 0.62 | 0.80 | 2026.00 | 2041 | 2163 |
geo odds[6]: | 0.30 | 0.63 | 0.82 |
Epistemic status:
- For Samotsvety track-record see: https://samotsvety.org/track-record/
- Note that this track record comes mostly from questions about geopolitics and technology that resolve within 12 months.
- Most forecasters have at least read Joe Carlsmith’s report on AI x-risk, Is “Power-Seeking AI an Existential Risk?”. Those who are short on time may have just skimmed the report and/or watched the presentation. We discussed the report section by section over the course of a few weekly meetings.
- Note also that there might be selection effects at the level of which forecasters chose to participate in this exercise; for example, Samotsvety forecasters who view AI as an important/interesting/etc. topic could have self-selected into the discussion.
- (Though, the set of forecasters who participated this time and participated last time is very similar.)
Update from our previous estimate
The last time we publicly elicited a similar probability from our forecasters, we were at 32% that AGI would be developed in the next 20 years (so by late 2042); and at 73% that it would be developed by 2100. These are a bit lower than our current forecasts. The changes since then can be attributed to
- We have gotten more time to think about the topic, and work through considerations and counter-considerations, e.g., the extent to which we should fear selection effects in the types of arguments to which we are exposed.
- Some of our forecasters still give substantial weight to more skeptical probabilities coming from semi-informative priors, from Laplace’s law, etc. But overall, we have also moved into being guided by more direct considerations: thinking about how AI progress will look year-by-year, what is the probability of a slowdown, what is the probability of a China/Taiwan war, etc.
- We have also spent significant time interacting with state of the art systems. We have seen other people producing new and exciting applications on top of these models. Just recently, Microsoft was in talks with OpenAI to invest renewed billions. This all gives us another round of information.
Acknowledgments
Thanks to Aaron Ho, Nuño Sempere, Greg Justice, Pablo Stafforini, Vidur Kapur, Misha Yagudin, Jared Leibowich, Tolga Bilge, Jonathan Mann, and Eli Lifland. for contributing to the discussion and/or submitting forecasts.
- ^
A person who answers a range of general and specific knowledge questions better than 95% of the general population.
- ^
P(AGI by ∞) is between 80% and 90% due to non-recoverable catastrophic risks, though this is very uncertain.
- ^
P(AGI by ∞) is between 80% and 90% due to x-risks other than AI misalignment. They note that P(x-risks) goes up with more advanced AI systems being available and used (e.g., used in nuclear command & control).
- ^
This depends significantly on whether China will blockade or invade Taiwan in a relevant timeframe. We think probability conditional on no Cross-Strait troubles is roughly 20% more likely, but this is highly uncertain. See also http://metaforecast.org/?query=taiwan.
- ^
Just mean ± 1.96 stdev.
- ^
EdoArad @ 2023-01-24T11:22 (+38)
Plotting the estimates, we get:
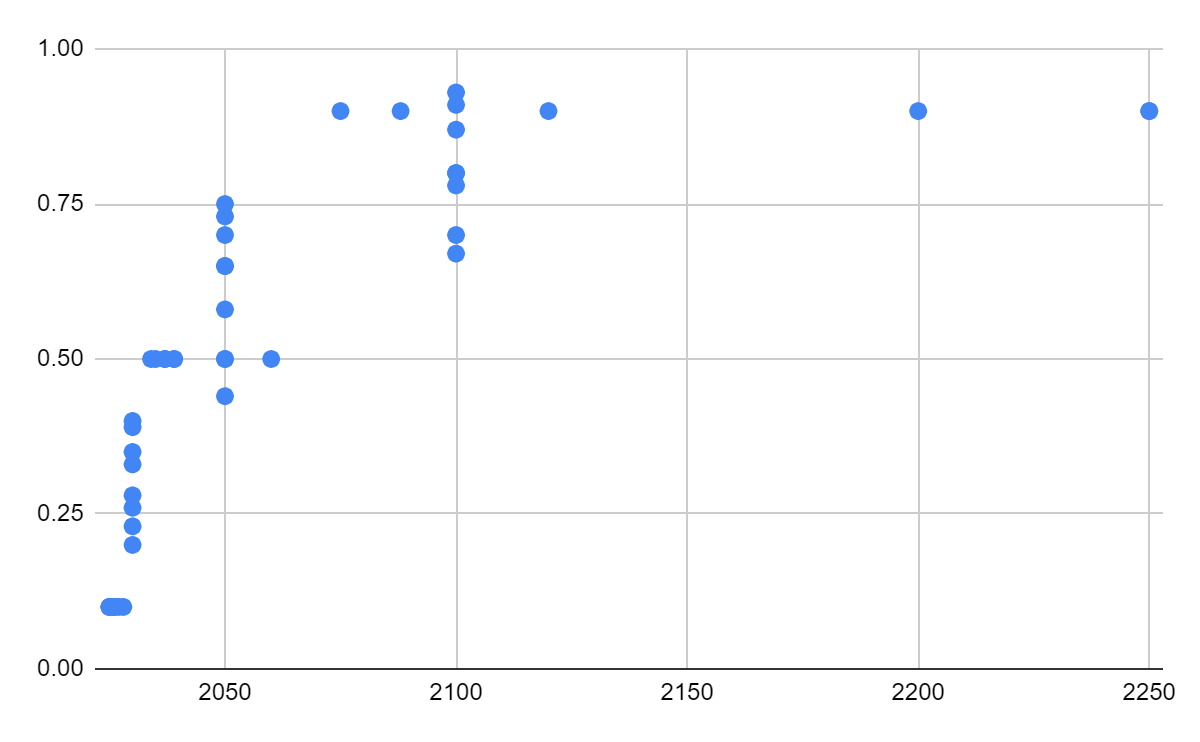
This looks logarithmic. Plotting the probability over Log(Year - 2022) does look linear (although clearly it is't, as it is bounded to [0,1], so a better fit would probably be something "arctan"y):
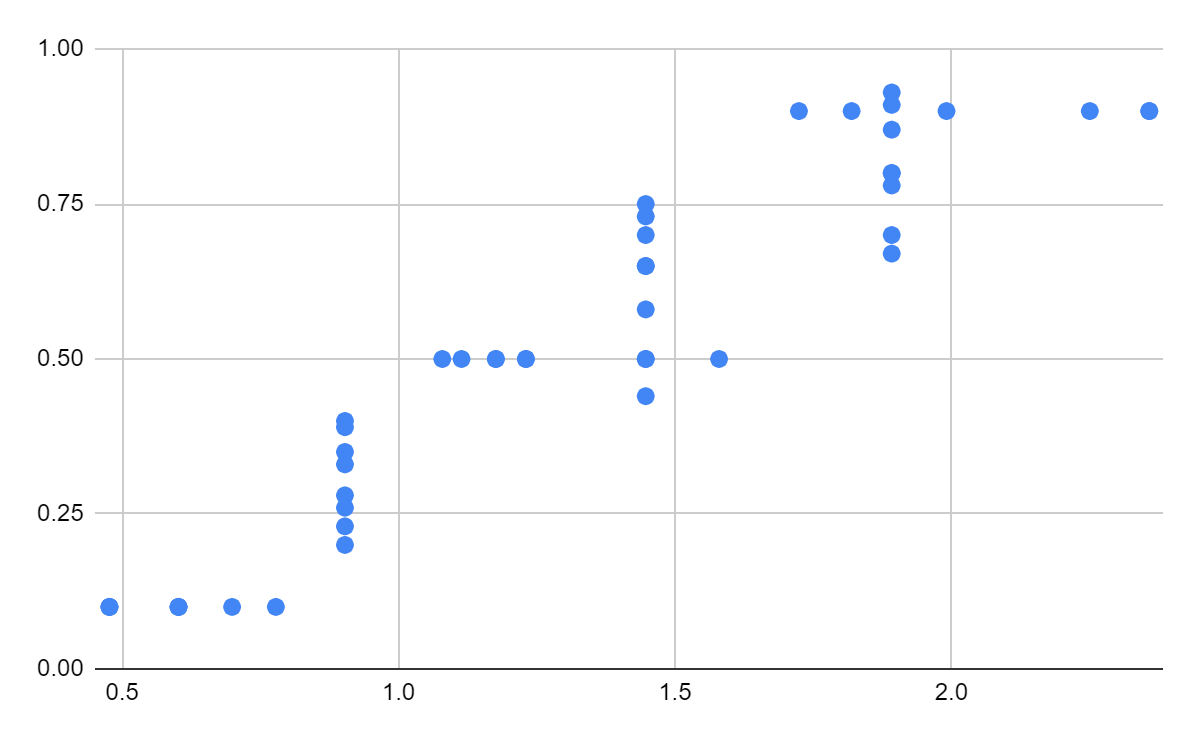
Also, it makes sense to me that uncertainty over "time until E" would behave more like a log-normal distribution (when the probability is fixed). That is, I'd expect that a forecaster's estimate for years-until-AGI in particular probability p would itself be a lognormal distribution over the years (as I imagine the forecaster would be equally likely to be wrong by twice as many years or half as many years).
This justifies taking the geometric mean over the years (as it corresponds to an average over the log of the years), but not when looking at the probabilities.
Fitting the curve with a linear function (excluding the N/As), we get
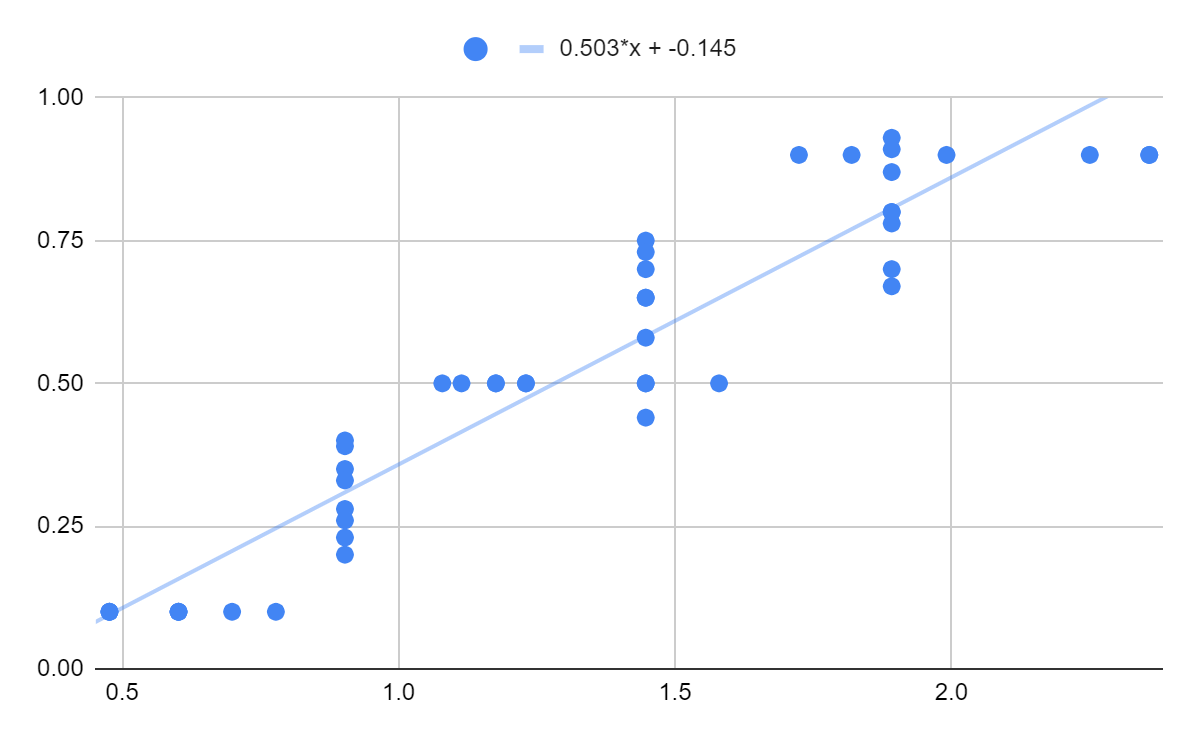
For , we'd get
For , we'd get
For , we'd get
Or, for a probability , we'd get the year .
For , we'd get
For , we'd get
For , we'd get
Overall, I got rather similar numbers 😊
NunoSempere @ 2023-01-24T11:30 (+5)
Nice, thanks for the analysis.
aogara @ 2023-01-24T18:49 (+24)
The adversarial Turing test seems like an odd definition to forecast on. Nuno's linked blogpost makes one side of the argument well: There could be ways to identify an AI as different from a human long after AI becomes economically transformative or capable of taking over the world. On the other side, AI that passes an adversarial Turing test could still fail to have economic impact (perhaps because of regulation, or maybe it's too expensive to replace human labor) or pose a meaningful existential risk (because it's not goal directed, misaligned, or capable of overpowering humanity).
I'd be more interested in your forecasts on a few other operationalizations of AI timelines:
- Economic impact, as measured by GDP growth rate or AI as % of inputs to GDP, seems like an important aggregate to track and forecast. It has the important quality of being easily verifiable and continuous over time, making forecasts easy to validate with each passing year. On the other hand, economic impact will likely lag cutting edge capabilities, which might pose the most x-risk.
- X-Risk is what I actually care about. With all the debate over whether AI x-risk is disjunctive or conjunctive, I wouldn't want to use a model split into "Will we get AGI, and if so, will x-risks be realized?" that has clear cases where x-risk could occur without first meeting the AGI definition. A tougher question is whether to forecast the exact date of human disempowerment, or a preceding "point of no return", or another set of ideas. But all of these seem more directly aimed at the most important question of x-risk.
- A particularly clean decomposition is "In what year will world energy consumption first exceed 130% of every prior year?" from Matthew Barnett's Metaculus question. This is designed to forecast transformative AI while accounting for the possibility that AI will overpower humanity, causing GDP to collapse as AI seizes all available resources for its own goal. Forecasting both this question and the economic impact question might show your x-risk estimate in the difference, unless you think that AI could overpower humanity without transformative industrial capacity.
Your thinking on these questions has been pretty persuasive to me, especially Nuno's recent blog and Eli Lifland's writeup of thinking through the full case. It's nice to get a perspective that's just a bit outside of the constant AI hype bubble. But these forecasts just felt a bit less informative than they could otherwise be, driven by edge cases around the definition. Curious if you would disagree with the importance of those edge cases, or think other forecasting targets have important flaws.
Misha_Yagudin @ 2023-01-24T18:58 (+9)
Thank you! We agree and [...], so hopefully, it's more informative and is not about edge cases of Turing Test passing.
We chose to use an imperfect definition and indicated to forecasters that they should interpret the definition not “as is” but “in spirit” to avoid annoying edge cases.
aogara @ 2023-01-31T23:25 (+2)
Fair enough. I think people conceive of AGI too monolithically, and don't sufficiently distinguish between the risk profiles of different trajectories. The difference between economic impact and x-risk is the most important, but I think it's also worth forecasting domain-specific capabilities (natural language, robotics, computer vision, etc). Gesturing towards "the concept we all agree exists but can't define" is totally fair, but I think the concept you're gesturing towards breaks down in important ways.
NunoSempere @ 2023-01-24T19:19 (+5)
as measured by GDP growth rate
Note that GDP is a bad metric here because it doesn't captura value created (e.g., Wikipedia doesn't particularly increase GDP in proportion to its value), and e.g., we might also expect GPT-3 and descendants to fall prey to Jevons paradox.
David Atkinson @ 2023-01-26T21:02 (+16)
In case people are interested, while updating updating Epoch's Literature Review of AI Timelines with this new forecast, I created a small notebook which uses a slightly different approach to aggregating the individual forecasts. Briefly, I fit a Gamma distribution to each forecast, which produces a CDF. Then, I combine the fitted CDFs using the geometric mean of odds. (This general approach is inspired by what AI Impacts has done in their expert surveys, although they use a different method of aggregating the fitted CDFs.) The final results are pretty similar, although a little more aggressive in the short- and longer-term, and a little less aggressive in the medium-term:
P(AGI by ___):
- 2030: 28%
- 2050: 59%
- 2100: 89%
Year when P(AGI) reaches ___:
- 10%: 2024
- 50%: 2043
- 90%: 2104
The notebook also includes some simple plots of the forecasts which you can play with.
MichaelStJules @ 2023-01-24T06:05 (+11)
Are you making short term (e.g. 1 year) AI-related forecasts, too? It would be helpful to figure out how much weight to give to your forecasts in this domain (and between individual forecasters), and may also give useful feedback for your longer range forecasts.
Misha_Yagudin @ 2023-01-24T06:14 (+14)
I've preregistered a bunch of soft expectations about the next generation of LLMs and encouraged others in the group to do the same. But I don't intend to share mine on the Forum. I haven't written down my year-by-year expectations with a reasonable amount of detail yet.