Exploratory Careers Landscape Survey: Community Members
By Vaidehi Agarwalla đ¸ @ 2022-12-16T18:54 (+16)
This is a Draft Amnesty Day draft. This means that this post may have some errors, or be missing some anlaysis. If it weren't for this initiative, we may not have published this post for some time. |
Thank you to Amber Dawn for editing and getting this post over the finish line, Neha Georgie, Arjun Khandelwal and Sarrin Chethik for reviewing drafts of this post, and to all the group organisers who took the time to complete the survey. Mistakes are ours.
In 2019-2020, a few group organisers founded & ran a project called the Local Career Advice Network, a voluntary network of EAs seeking to improve the quality and availability of localised career advice (particularly outside the US and UK). This survey was conducted by the LCAN in February 2020. We used the results from this survey to prioritise our Fall 2020 activities.
Key Takeaways
- From a survey of 27 group organisers from around the world, we found that the biggest career bottlenecks for community members were: freedom of movement (situational), information resources (situational), motivation (internal) and personal uncertainty (internal). (Read more about the discussion of these factors)
- Survey design is challenging: It was difficult to gather the information efficiently and without biassing survey respondents. Weâve included a detailed write-up of our methodology that we hope others will find useful if doing similar work. (Read More about our methodology, and our evaluation of the design).
- As a byproduct of this research, we created a Career Factors Framework which systematically maps out specific factors which can expand or limit viable career paths. Survey participants found the framework useful for thinking about different potential bottlenecks and how they might address them. (Read more about the development of the framework)
- We outline steps we considered (and actually took) to address the identified bottlenecks. The main outcome of this survey was to run a career 1-1 training workshop for group organisers which had positive results. (Read more)
Goals
Because of our own uncertainties and feedback from stakeholders, the Local Career Advice Network team decided to evaluate our initial assumptions about the major career-related bottlenecks facing EA group members at the end of 2020. So, we decided to survey EA group organisers and ask them explicitly about their membersâ bottlenecks.
As we designed & analysed the survey, we tried to be careful to:
1) avoid biassing group organisers' responses
2) account for group organisers' biases
3) accurately judge the representativeness of different groupsâ responses
Initial Hypothesis
Before conducting the survey, our hypothesis was that the main career bottlenecks for EAs in underrepresented countries were:
- A lack of career opportunities such as promising jobs, internships, and volunteer opportunities to make progress on EA cause areas in those regions. This doesnât just mean a lack of opportunities at EA organisations, but rather a lack of knowledge about other high-impact opportunities that may be worth pursuing (e.g. roles in local government, think tanks, academia, etc.).
- A lack of freedom of movement, i.e., the inability to move geographic regions for a job
This was based on group discussions and informal conversations with ~20 group organisers, mainly from outside of the US/UK. One community builder at a meta organisation at the time thought it was possible that the main bottleneck was instead that members in those groups weren't as talented or promising as those in other groups.
Based on this feedback, we wanted to develop a way to evaluate any and all bottlenecks EA community members might face, and survey organisers in a way that didnât influence their responses.
Methodology
Deciding on an approach
We first deliberated about whether we should take a structured, semi-structured or unstructured approach to surveying respondents about their group membersâ bottlenecks. We outlined some advantages and disadvantages (summarised in the table below) of each approach.
Table 1: Attributes of different survey approaches
Approach | |||
Attribute | Structured | Semi-structured | Unstructured |
Methodology | Quantitative Survey | Qualitative Survey | Case Studies, Interviews |
Sample Size | Large | Medium | Small |
Data Accuracy (excluding influencing respondents) | Low | Medium | High |
Time-Cost (respondents) | Low-Medium | Medium | High |
Time-Cost (surveyors) | Low | Medium-High | High
|
We decided on the semi-structured survey approach. Our reasoning was as follows:
- Both we and our respondents had limited time. For us, LCAN is a volunteer project and both the survey designers had full-time jobs. Many of our respondents had their own responsibilities with their groups or full-time jobs. At this point we had been working on LCAN for 4 months and wanted to start testing out different ideas. We were also wary of organisersâ survey fatigue. This ruled out an unstructured approach.
- We wanted to get a representative sample of groups from around the world. This was another reason why the unstructured approach could not be used: this would mean that we would only have capacity to interview a small number of organisers.
- We were very uncertain about the results we might get, and didnât want to compromise data accuracy in order to make the survey quicker to fill out. We didnât want to bias and limit the answers from respondents with the career factors framework, so we let them brainstorm bottlenecks first, before introducing them to our framework. This ruled out a purely structured approach.
Thus we decided on the semi-structured approach. This consisted of both structured and open-ended questions, which will be described in detail below. We were ultimately able to get 27 respondents, notably 11 who were national group organisers and could speak about general trends in their countries. We think that we werenât able to get more responses because the survey did take a reasonable time to complete (around 30+ minutes). To account for this, we asked a number of demographic questions to help us weight responses, such as how much time the organisers spent on community building and careers advice, the size of the group, and so on.
Developing the semi-structured approach
In the semi-structured approach, we wanted to investigate the different factors that could affect (positively and negatively) a personâs career transition into a career with high(er) impact. At first, we experimented with trying to identify different âtypesâ of members who had a similar cluster of career factors that enabled or limited them which group organisers may be able to discuss (e.g. someone who has the relevant career capital for a path, but perhaps lacks the resources or work authorization to pursue it). As we started discussing these different âtypesâ we realised that 1) we didnât have sufficient data to know whether these types were accurate and 2) we could instead try to simply identify the different factors that affect someoneâs career transition, and ask questions about these to the respondents.
We then developed the career factor framework (see below). Using this framework, we still needed to figure out how we wanted to ask respondents about these factors. At first we considered asking group organisers to identify the âtypesâ themselves. The rationale behind this was to see if there were some very talented people who faced the same bottlenecks. But we realised that this might be too complicated and could make the data quite messy. It would also probably take too much time, as organisers would need to think about individual group members and (particularly in larger groups) they may not know most members personally, or if they did it would take too long. We didnât want the survey to be too complicated or cumbersome and put respondents off. For that reason, we also couldnât ask precise quantitative questions such as, for example, the numbers of members who faced a particular bottleneck.
In the end, we decided to ask respondents to freely brainstorm the different bottlenecks faced by their group members, and then ask them to isolate and categorise individual bottlenecks, with the understanding that many factors could correlate and overlap.
The downside of this approach is that we lacked the nuance of individual cases. We wanted to use the data we gathered to help us identify the big picture issues, and discover areas that needed more focus or more targeted research.. We try not to make too many inferences from the data.
Developing the Career Factors Framework
We started with 80,000 Hoursâ formula for a perfect job. However, we found it didnât break down the possible factors into enough depth for the purposes of our study. Importantly, it didnât account for the situational factors that weâd observed from the group organisers weâd spoken to before. In particular, we wanted to take another look at the concept of âpersonal fitâ, which is defined by 80,000 Hours as: âYour degree of personal fit in a job depends on your chances of excelling in the job, if you work at it.â
We grouped the individual factors identified into three high-level categories:
1. Internal Factors
2. Situational Factors
3. Career Capital Factors.
Throughout the process of developing this framework we sought out feedback from group organisers, especially those with significant experience running career 1-1s.
Table 2: Career Factors Version 1.0
The definitions of each category and factors are listed in the table below.
Category | Definition | Factors |
Internal Factors | Factors internal to the person which may include motivational, personality or other traits. | Innate abilities Knowledge of EA Path uncertainty Personal Uncertainty |
Situational Factors | Factors external to the person, which can be linked but are not limited to socioeconomic status, nationality, social connections and health. | Economic resources Social and Health Resources Freedom of Movement Flexibility of career capital |
Career Capital Factors | Factors that are directly related to a given career path and your technical ability to excel in it. Unlike the 80K definition, we have defined this to be more closely associated with career-specific factors. | Skills Experience Credentials Connections |
The above is a summary of the first version of the Career Factors Framework . After conducting the survey, we updated the framework in accordance with the responses. We will describe this process in the data cleaning & code section below.
Questions
We asked respondents:
âPlease take a few minutes to write the most important or salient bottlenecks that come to mind when thinking about the members of your local group. Treat this as a brainstorm session and write as many things as you can think of, in the next section we will ask you to categorise these.â
We then briefly explained our framework and provided a brief overview of the categories and framework, as it was presented in the previous section.
We then asked respondents to identify individual bottlenecks from their brainstorm, and for each brainstorm asked them to:
- Categorise the bottleneck into none, one, or multiple of the factors from our framework. If they couldn't categorise the bottleneck, we asked them to explain why.
- How widespread is this bottleneck? (Extremely prevalent, Moderately prevalent, Somewhat prevalent, Not at all prevalent)
- How difficult is it to overcome this bottleneck? (Extremely difficult to overcome, Moderately difficult to overcome, Somewhat difficult to overcome, Not at all difficult to overcome)
- Do you have any additional thoughts on this bottleneck?
Steps to reduce bias
Once we'd chosen the semi-structured survey approach, we then tried to design it to mitigate its weaknesses, and to make sure that we didn't bias respondents' answers. We did this by:
- Asking respondents to freely brainstorm bottlenecks before introducing them to our framework.
- Trying to make our framework as comprehensive as possible and including all possible bottlenecks.
We tried to ensure data accuracy by:
- Priming respondents to think about concrete situations by asking them to evaluate their career events first, and explicitly asking them to think about concrete situations
- After respondents categorised the bottlenecks, we gave them room to add more thoughts in case they wanted to clarify any points. Many respondents added more details and we found this clarification useful in helping improve the categorisation to be more consistent.
- Respondents could categorise their observed bottlenecks by selecting one, many, or none of the career factors. They could also suggest their own factors if they wanted to (none did).
- We also allowed respondents to add additional bottlenecks that weren't in their original brainstorm after they'd used our framework. We tried to mitigate the influence of the framework by taking into account the reported prevalence of different bottlenecks.
- Since many factors overlap with each other, we did two comparisons of factor co-occurrence to make sure we could capture the interactions between factors.
- We created a heat map of the co-occurrence of factors across groups to see whether there were clusters of factors that appeared together.
- We created a chart of factors within bottlenecks based on respondents' original coding since many respondents picked at least 2 or more factors for each bottleneck, but in our recoding we picked the one dominant factor that we think matched that bottleneck the best. This served as a check on how well we re-coded the bottlenecks.
Data Cleaning and Coding
We expected that we would need to re-categorise the constraints given respondents' limited time with our framework and how interrelated constraints can be. We re-coded the factors by:
- Categorising uncategorised bottlenecks
- Recategorizing bottlenecks into one dominant factor
- Splitting up 1 bottleneck into two if there appeared to be 2 equally dominant factors
- Creating new factors based on responses
We used context from the respondentsâ initial brainstorm, their categorization, description of the bottleneck identified and the additional thoughts to make sure we were categorising the information accurately. For most bottlenecks, we ended up choosing a factor that was among the factors the respondent had originally chosen. Where we couldnât make a good judgement call we excluded these responses from the analysis. We also decided to only list a factor once per group, to avoid over-counting. It didnât change the data by much, but weâve decided to just go with the 1 factor.
Coding Career Factors
This chart shows the comparison between the original coding respondents gave to the bottlenecks they identified, as compared with our final re-coding. Each column breaks down our final coding of the factor into the originally assigned factors. So, for example, for the âSkillsâ factor, only 4 respondents originally coded this factor as a âSkillsâ, while the remaining respondents coded it as something else.
For ease of comparison, weâve also colour-coded the career categories: dark green for career capital, blue for internal factors and light green for situational factors.
As a test of the relevance of our framework, the 2020 EA Survey asks participants what bottlenecks their impact. Here are their responses:
Respondent bias aside, many of these reasons fit quite neatly into our framework categories, as shown in the table below for the top 10 (excluding ânothingâ):
Finances | Economic Resources | 89 |
Network | Connections | 49 |
Productivity | Economic Resources | 36 |
Uncertainty | (less certain) Personal Uncertainty or Path Uncertainty | 34 |
Mental Health | Social and Health Resources | 28 |
Job opportunities | Opportunities | 27 |
Direction | (less certain) Motivation or Path Uncertainty | 21 |
Time | Economic Resources | 21 |
Career change | (less certain) Flexibility of career capital? | 20 |
Education | Credentials | 20 |
Interestingly, the limit on impact is dwarfed by finances, with over 50% of the economic resources factors. This may be because participants are measuring their impact more by ability to donate than ability to use their careers, unlike our respondents who were focused on the latter.
Limitations of the Data
Despite these efforts, we believe there are still biases in our data. We have outlined many of our main concerns in the first post of this survey writeup. Here are a few other limitations:
Respondentâs lack of information: Group organisers don't know everything about their members, even those who know their members quite well.
- Only a few group organisers mentioned social and health resources as a problem amongst their group members. This may be because members are not open with group organisers about these kinds of challenges. Based on general discourse in the community, we believe that this may be more of a barrier than suggested by the results here. In the cases that health is a concern, it could be a major constraint. (In general, we encourage group organisers to consider the prevalence of each bottleneck for their own group rather than look at the average results).
- Respondents may not have been exhaustive in their listing of bottlenecks to save time when filling out the survey.
Results & Discussion
Overall, there was no one standout bottleneck or category of career bottlenecks. Situational factors and internal factors were almost equal at 38% and 35%, followed by career capital factors (24%) and other factors that we were unable to categorise (5%) (see pie chart). The top 5 factors cross all three categories, and are ranked closely together.[1]
Although we don't discuss this data in detail below, this data seems useful to contextualise how organisers see their members' actions overall:
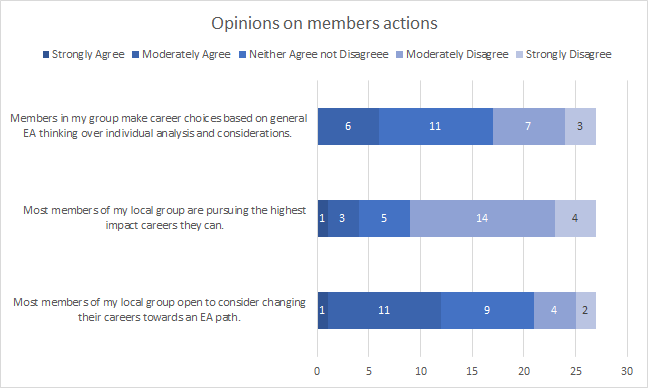
The top bottlenecks
Opportunities and freedom of movement
We expected freedom of movement to be a top bottleneck for non-US and non-UK organisers. Issues included difficulties getting work visas, financial or difficulty communicating with their parents about EA jobs, and separation from social support networks of friends and family. The main co-listed factor is Economic Resources, suggesting this could contribute (this seems more plausible than the opposite connection). However, our respondents also specifically cited an overall lack of jobs (not just location-specific opportunities), so we coded these separately and added Opportunities as a new factor. Opportunities are also related to the Connections factor (3 respondents tagged these bottlenecks as Connections).
We had 8 total mentions of a lack of EA jobs, with 6 unique respondents citing a lack of local EA-aligned jobs as a bottleneck for their members, and an additional 2 repeated respondents citing a general lack of opportunities. Some respondents cited a lack of EA jobs in particular, other barriers were: language, country/location, and a lack of remote jobs. Others cited limited work opportunities at effective organisations (not necessarily EA) or even any organisations focused on relevant causes. Some respondents cited a lack of opportunities to test fit for undergrads, or on short time scales (both university groups), and one of them cited a lack of suitable academic supervisors for AI safety work in their country.
Motivation
We added âmotivationâ as a factor because several respondents cited it. We had hypothesised that motivation would be a complex issue, and the results suggest that this is correct. Respondents cited numerous reasons why members lacked motivation including people feeling like they canât contribute because they lack a scientific background, challenges due to being later in their career, lack of ambition or initiative, lack of interest or commitment to EA. Respondents who did mention motivation explicitly in their free brainstorms, categorised it as personal uncertainty, path uncertainty and knowledge (however, motivation was not an option in the default, and people have strong preferences towards defaults in general).
We thus still think it could be more actionable to address the most prominent causes of low motivation, or one of the other prominent bottlenecks, but itâs possible that interventions that tackle motivation holistically may be worth investigating further.
These reasons may have deeper causes such as: a lack of self-efficacy exacerbated by a lack of knowledge, or needing a nudge to apply to impactful positions. Further, lack of motivation can itself be a root cause of other issues. For example, 3 respondents said that people who were unwilling to move lacked motivation.
Personal uncertainty
Personal uncertainty (internal factors) was a top bottleneck. The most common reason for personal uncertainty (cited by 4 respondents) was low confidence or self-esteem. Reasons for low confidence were: decision paralysis because of the pressure to do the âmostâ good, feeling that you canât have an effective career if you donât fit into one of 80,000 Hoursâ jobs, and imposter syndrome. Thus personal uncertainty could be resolved by improving the communication of EA career advice and ideas. Other points:
- One respondent noted that this was especially the case for women.
- One respondent suggested that it could be effective to offer mentorship and to change EA messaging to celebrate a wider range of paths and emphasise that the movement will adjust to individualsâ plans.
- One respondent mentioned uncertainty regarding what to do in their country.
Respondents originally coded personal uncertainty under knowledge, path uncertainty and credentials. These are not surprising co-listings.
Skills
Overall, there was acknowledgement that solving the issue of skills (career capital) was fairly difficult. One person suggested headhunting to fill high impact career paths in their country. Another mentioned the confusion between skills and talent, and finding it hard to know what is innate and what is learnable. In particular, this organiser mentioned that their members are âhighly educated and skilled people, but I do think getting high-impact roles is very hard to do. Internships or volunteer positions are hard for some people to get, and that is to be expected. I believe personal confidence/charisma/social skills might be something that limits some of my group members (as well as more credentialed things).â
One organiser mentioned not knowing how to support people when they arenât well-suited to 80Kâs priority paths, which is likely to relate to skills, but may also relate to innate talent or other career capital factors.
One person mentioned challenges because most of their active members are fresh graduates or college students lacking skills and credentials (because they attended the local universities) and two mentioned a lack of fluent English as a barrier, one stating âThe current world language isn't English, but bad Englishâ.
Updating our career factors framework
We updated our career factors framework as a result of the survey.
- We added motivation to the âinternal factorsâ category. We had initially brainstormed but then excluded this because we had hypothesised that motivation was a very complicated factor, and it would be more useful to know the underlying issues. Since it was explicitly mentioned several times, we added it back in.
- We added Opportunities to the situational factors as a new category, in addition to freedom of movement. The difference is that lack of opportunities was a lack of jobs or opportunities overall (agnostic to the region) rather than the inability or unwillingness to get existing opportunities due to limited mobility.
- We added Information resources to situational factors. Respondents mentioned a lack of sufficient information about high impact jobs and about different jobs' salaries and other policies. Some mentioned that it would be useful to have more tailored advice, more people specialise in giving EA career advice, and better material for workshops.
Evaluation of the survey design
We didnât ask our organisers how they define a successful career change, and used the term âpriority pathâ throughout our v1 of our career factors framework, which refers to 80,000 Hoursâ priority paths. We think this is a significant flaw in our design.
This question seemed less pressing at the time the survey was conducted in early 2020. The de facto assumption was that 80,000 Hoursâ priority paths (and some other prominent EA paths) were highly valued (~50% of group organisers in our survey cited use 80K as a resource âall the timeâ), and we assumed (we think mostly rightly) that most organisers, especially those on community building grants (who were evaluated on the basis of these metrics) saw these as the highest impact paths. Additionally, many group organisers discussed paths in animal welfare and global health and development in their responses, which werenât explicitly listed as 80K priority paths.
We now believe that getting a sense of organisersâ strategy and perspective would have been valuable and would ask both how respondents define a successful career change, use the term âhigh impact pathsâ and make it clear that this doesnât imply that 80,000 Hoursâ priority paths were all/most of possible high impact paths.
Next Steps Considered & Taken
We could address the freedom of movement bottleneck by helping members identify promising local and remote opportunities, and improve information resources by generating more knowledge about promising paths, fields, and organisations on a locally-relevant level.
- LCAN ran a pilot group of 3-4 organisers conducting local priorities research in summer of 2020 with mixed results.
Both motivation and personal uncertainty could potentially be alleviated by addressing the situational factors discussed above.
Improving 1-1 career advice could also address these issues. Career 1-1s were the top-rated event in the career activities evaluation, and there appears to generally be high demand for them in the community. We believe that personal uncertainty in particular could be addressed by career 1-1s. They are highly personalised and targeted, meaning that if they are done well an organiser can help identify the root cause of an uncertainty and potentially help address it. Additionally, organisers can provide support and encouragement which could improve motivation or uncertainty.
- LCAN conducted a pilot career 1-1 training workshop with positive initial results.
- ^
Notes: 6 factors were categorised as âOtherâ because they were either too general and were applicable to almost all or all factors, or they were not directly relevant to the question. We have excluded these from our analysis, apart from acknowledging them in this summary chart.
Factors with a high percentage (40-75%) of NAs (Connections, Information Resources, Knowledge of EA and Innate Talent) are because we discarded previous prevalence scores when recoding and/or because the respondents left this field blank. These bottlenecks are lower in frequency, but we included it to show the range of responses, and how complex this issue can be.