A Landscape Analysis of Institutional Improvement Opportunities
By IanDavidMoss @ 2022-03-21T00:15 (+97)
Summary
The Effective Institutions Project is a global working group that seeks out and incubates high-impact strategies to improve institutional decision-making around the world. Over the past several months, we conducted a landscape analysis to identify which of the world’s institutions (e.g., prominent government agencies, corporations, NGOs, etc.) offer the highest-leverage opportunities for philanthropists and professionals seeking to improve world outcomes over the next 10-100 years.
Methodology
To build a short list of important institutions to prioritize for deeper analysis, we combined existing lists of the world’s top private foundations, employers, universities, etc; assessed which institutional affiliations appeared most often among the members of global commissions convened over the past five years; and solicited nominations of important institutions from 30 global experts representing a range of fields, geographies, and professional networks.
Next, we conducted research and developed 3-5-page profiles on 41 institutions. Each profile covered the institution’s organizational structure, expected or hypothetical impact on people’s lives in both typical and extreme scenarios, future trajectory, and capacity for change.
Finally, we built a quantitative model to gauge the potential benefits of engaging with each of these 41 organizations by way of a hypothetical large, well-designed philanthropic investment. The model provided a rough estimate for the impact that an investment of this nature might have on two outcomes of interest: 1) global wellbeing-adjusted life-years over the next 10 years and 2) reducing the probability of existential risks over the next 100 years. The analysis takes into account the feasibility of influencing each institution and the likelihood that equivalent improvements would be implemented in the time frame anyway.
Key Findings
Our analysis suggests that opportunities to drive better world outcomes by improving institutional decision-making are relatively concentrated among a small number of organizations. This finding holds across both of our outcomes of interest, and the top-ranked institutions turned out to be largely the same in each analysis.
Our list of top institutions features heavy representation from tech companies and US government bodies, six of which ranked among the top ten in both our 10-year and 100-year analyses:
- Amazon
- DeepMind
- Meta (formerly Facebook)
- OpenAI
- The Executive Office of the US President
- The US National Security Council.
Additional top-ranked institutions from a near-term perspective included Google and the World Health Organization. Additional top-ranked institutions from a long-term perspective included Alphabet, the European Union, and the US Congress. Finally, we assessed that Chinese citizens and organizations may have opportunities to improve world outcomes via engagement with the Politburo of the Chinese Communist Party and China’s State Council on certain non-politicized issues.
Our extremely rough, please-don’t-take-these-numbers-literally estimate is that an investment of $100 million a year focused on well-designed strategies in this arena would be worth the equivalent of saving 4-30 million lives (20-200x as cost-effective as GiveWell’s top charities) over the course of a decade, or eliminate as much as one-tenth of total existential risk from all sources over the course of a century.
Conclusions and Next Steps
This article is a first attempt to answer a research question of vast complexity conducted with very limited resources (about 250-300 hours of effort in total across all parties). All conclusions put forward here should therefore be regarded as tentative and subject to revision as we learn more.
Throughout 2022, Effective Institutions Project will undertake deeper dives into selected institutions above in an effort to identify promising opportunities and strategies to make a difference. We will also continue adding to the breadth and robustness of this landscape analysis.
Introduction
The Effective Institutions Project is a global working group that seeks out and incubates high-impact strategies to improve institutional decision-making around the world. We analyze how and in what ways institutions’ decisions influence people's lives, study how key institutions currently make decisions, identify interventions that might cause those institutions to take actions that will lead to better global outcomes, and mobilize funding and talent to execute on the most promising interventions. Alongside all of this, we are building an interdisciplinary network of reformers to share insights, coordinate efforts, and steadily increase the odds of success over time.
In summer 2021, EIP published a framework for prioritizing among strategies to improve decision-making practices at specific institutions. The framework offered definitions for several key terms (“institution,” “decision-making,” “improving”) and included two case studies demonstrating the method. It identified two potential pathways for improving institutional decision-making: 1) bringing an institution’s mission or stated goals into better alignment with the interests and wellbeing of everyone affected by its decisions, not only its most direct or powerful or visible stakeholders; and 2) increasing the likelihood that a well-intentioned institution will achieve its desired outcomes by, for example, considering better options and promoting more accurate team judgments.
At the time, we indicated that the next step was to apply the framework to as many institutions as we could to try to identify the biggest opportunities to make a difference. In particular, we wanted to uncover answers to the following three questions:
- Among all the world’s institutions, which represent the greatest opportunities to improve life on Earth, both today and far into the future?
- To what degree are opportunities to make an outsized difference concentrated among just a few institutions vs. spread far and wide?
- How do these conclusions differ across neartermist vs. longtermist worldviews?
This article represents the continuation and initial conclusions of that work.
Our Process
At the outset of this exercise, we faced a major logistical challenge, which is that there are millions of organizational entities that could qualify as “institutions” by our definition. We knew that applying our prioritization framework separately to each of these would not be feasible, so we needed a not-completely-arbitrary way to whittle this massive set of potential contenders down to a list short enough to fit our bandwidth constraints. We approached this challenge from three different directions. First, we constructed a “long list” of key institution candidates by combining lists of the world’s top private foundations, employers, universities, etc. Second, we analyzed a recently completed inventory of global commissions to assess which institutional affiliations appeared most often among the commissions’ members. Finally, we asked for and received nominations of important institutions from 30 global experts representing a range of fields, geographies, and professional networks. (We had also intended to conduct a fourth analysis, synthesizing existing lists of the world’s most important institutions, but were unable to find any that approached the question through a comparably holistic lens.) Synthesizing the above information inputs, we curated a “short list” of 77 institutions to prioritize for further research.
Long list
We began this work by combining lists of top institution candidates from existing, mostly category-specific sources including the 80,000 Hours and UNJobs lists of notable employers, the largest private foundations, top-ranked global universities, the Fortune 100, the largest corporate R&D spenders, and so forth. After deleting duplicates and clearly irrelevant entries, we then supplemented the list with relevant national government agencies and political parties, making sure that the top countries by population and/or GDP as well as nuclear powers were represented. We additionally asked contacts in our network for suggestions of powerful institutions in Latin America, Africa, and Asia since those regions were at risk of underrepresentation.
The final list encompassed 1207 institutions. Many judgment calls were necessary as we worked through criteria and edge cases, and there is assuredly still room for improvement. Nevertheless, it provided a helpful starting point and ongoing reference for constructing a broad view of important institutions around the world.
Inventory of commissions
We next consulted an inventory of commissions that was assembled for the Global Commission on Evidence to Address Societal Challenges, whose co-leader, John Lavis, is an advisor to the Effective Institutions Project. On behalf of the Evidence Commission, Kartik Sharma and Hannah Gillis catalogued 55 previous global commissions addressing societal challenges from 2016 to 2021 on subjects ranging from post-pandemic policy to land use to media freedom. As part of the analysis, Sharma and Gillis recorded the institutional affiliations of commission participants, which could then be used as a proxy for institutional importance or centrality.
The most common institutional affiliations in the dataset were the UN, World Bank, Oxfam International, African Union, Bill and Melinda Gates Foundation, and World Economic Forum. The complete ranking of institutional affiliations can be viewed here.
Expert survey
Finally, we drafted a brief survey and sent it to a curated list of 92 experts including noted institutional scholars, retired politicians and diplomats, foundation executives, NGO leaders, senior consultants, and journalists. We asked respondents to nominate at least five institutions (i.e., government agencies or legislative bodies, corporations, NGOs, foundations, media companies, etc.) that they identified as “among the most powerful or influential in the world,” to justify why they chose those specific institutions, and to explain the logic undergirding their selections. We also offered them the opportunity to name additional institutions and to suggest additional survey respondents.
Overall, we received 30 responses. The following respondents agreed to be named publicly:
- Ṣẹ̀yẹ Abímbọ́lá, Lecturer, Public Health at the University of Sydney; editor-in-chief, BMJ Global Health
- Joslyn Barnhart, Assistant Professor of Political Science at the University of California, Santa Barbara; Senior Research Affiliate, Future of Humanity Institute at Oxford University
- Norman Bradburn, Tiffany and Margaret Blake Distinguished Service Professor Emeritus at the University of Chicago, former chair of the Department of Behavioral Sciences and provost of the university
- Frances Brown, Senior Fellow and Co-Director of the Democracy, Conflict, and Governance Program, Carnegie Endowment for International Peace
- Jacob Harold, co-founder and former Executive Vice President, Candid
- Marek Havrda, Deputy Minister of European Affairs, Office of the Government of the Czech Republic
- Charles Kenny, Director of Technology and Development and Senior Fellow, Center for Global Development
- Bruce Jones, Senior Fellow, Brookings Institution
- Ruth Levine, CEO, IDinsight; former director of the Global Development Program at the William and Flora Hewlett Foundation
- Nancy Lindborg, CEO, the David and Lucile Packard Foundation
- David Manheim, lead researcher for 1Day Sooner and Superforecaster for Good Judgment Inc.
- Dylan Matthews, editor, Future Perfect at Vox
- Sarah Mendelson, Distinguished Service Professor of Public Policy at Carnegie-Mellon University; former Senate-confirmed US Representative to the UN Economic and Social Council
- Clara Miller, President Emerita, the FB Heron Foundation
- Luke Muehlhauser, Program Officer, AI Governance and Policy at Open Philanthropy
- Eric Nee, editor-in-chief, Stanford Social Innovation Review
- Poorva Pandya, CEO, Empowering Farmers Foundation
- Dana Priest, longtime national security reporter for the Washington Post, John S. and James L. Knight Chair in Public Affairs Journalism at the University of Maryland
- Roya Rahmani, former Afghani Ambassador to the United States
- Suyash Rai, Deputy Director and Fellow, Carnegie India
- John-Arne Røttingen, Ambassador for Global Health, Norwegian Ministry of Foreign Affairs
- Fabiola Salmán, Partner and head of Latin American practice, Dalberg Advisors
- Rumtin Sepasspour, Research Affiliate, Centre for the Study of Existential Risk
- Carl Shulman, Research Associate, Future of Humanity Institute at Oxford University; Advisor, Open Philanthropy
- Camilo Umaña, urban economist, IDOM Consulting
In addition, we had five other respondents who preferred to remain anonymous, including experts at several think tanks and universities and a senior partner at a major global consulting firm.
The survey results indicated a surprising degree of convergence across respondent backgrounds and perspectives. The US Presidency/executive branch, US Congress, the Chinese Communist Party or the Chinese government more generally, and Meta/Facebook were each nominated by more than a third of respondents. Other institutions named at least five times include Alphabet (particularly the Google and DeepMind divisions), the Bill and Melinda Gates Foundation, the United Nations generally, and the UN Security Council.[1]
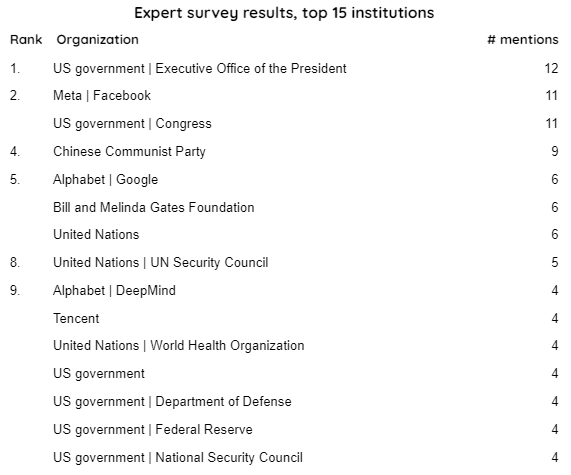
Short list
The inputs above, combined with a dash of editorial judgment from the project team, yielded a “short list” of 77 institutions divided into three priority tiers.[2] We were ultimately able to create individual profiles for 41 of these 77 institutions, including 100% of those in the top tier and 77% of those in the second tier. We established three levels of robustness indicating our level of confidence in each profile as follows:
- Basic: profile is based on desk research / author has no special knowledge of the topic
- Enhanced: author is knowledgeable about the topic and/or the profile has been reviewed by an outside expert
- Robust: profile has been reviewed by multiple outside experts
Of the 41 profiles, 24 (59%) met the criteria for Basic and 17 (41%) met the criteria for Enhanced, as follows:
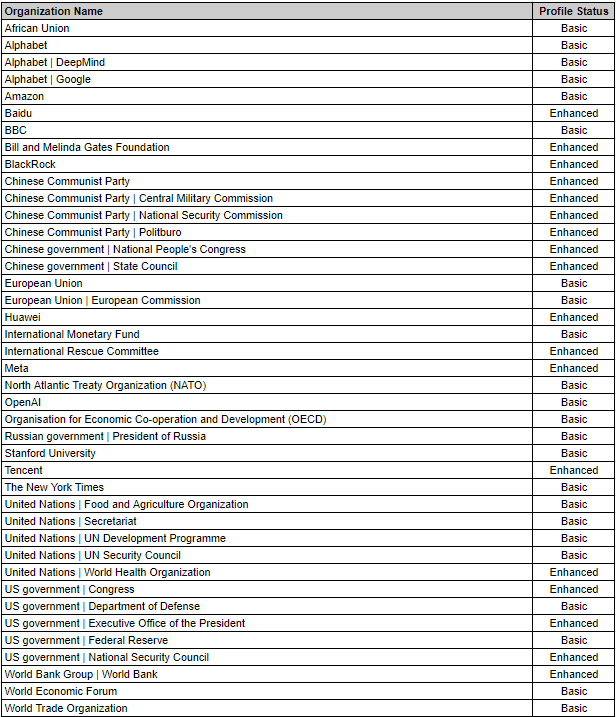
Quantitative model
Finally, we built a quantitative model to gauge the potential benefits of engaging with each of these 41 organizations by way of a hypothetical large, well-designed philanthropic investment.[3] The model, which was based on our previously-published prioritization framework, provided a rough estimate for the impact that an investment of this nature might have on two outcomes of interest: 1) global wellbeing-adjusted life-years over the next 10 years and 2) reducing the probability of existential risks over the next 100 years. The model features more than 1700 variables and takes into account the feasibility of influencing each institution and the likelihood that equivalent improvements would be implemented in the time frame anyway.[4] Our estimates were probabilistic in nature and the confidence intervals were very large for reasons detailed in the “Caveats and Limitations” section below, so the numerical outputs from the model should be treated as rough guidance rather than precise estimates. Nevertheless, the analysis yielded some fairly clear and surprising conclusions.
Top Institutions
Our findings suggest that there are substantial opportunities to drive better world outcomes by improving institutional decision-making, and moreover that (at least for now) these opportunities are highly concentrated in a fairly small number of organizations. Even more strikingly, and unexpectedly from our standpoint, this finding held across both outcome metrics we used – improvement of global health and wellbeing over the next ten years, and reduction of existential risks to humanity over the next hundred years – and the top-ranked institutions were largely the same in each analysis.
Based on an eyeball synthesis of Michael Aird’s database of existential risk estimates, we judged there to be about an ~8% probability in the median scenario of human civilization experiencing a disaster from which it never recovers over the next century. We estimate that smart and well-resourced institutional improvement efforts among the 41 organizations we profiled could eliminate up to ~1.2 percentage points, or 15%, of that risk.[5] Furthermore, over 70% of the total opportunity for x-risk reduction was concentrated in the top ten of those 41 institutions, and many of those in the next tier are subdivisions, competitors, or otherwise closely related to the top-ranked entities.
While we don’t have a similar global estimate of the total wellbeing-adjusted life-years at stake in any given year, we similarly observed a level of concentration among the top ten scorers in that category of about 70%. For these ten institutions alone, we estimate that successful institutional improvement efforts could conceivably be worth up to ~7 billion WELLBYs (equivalent to saving roughly 30 million lives) over the next ten years in the median scenario.[6]
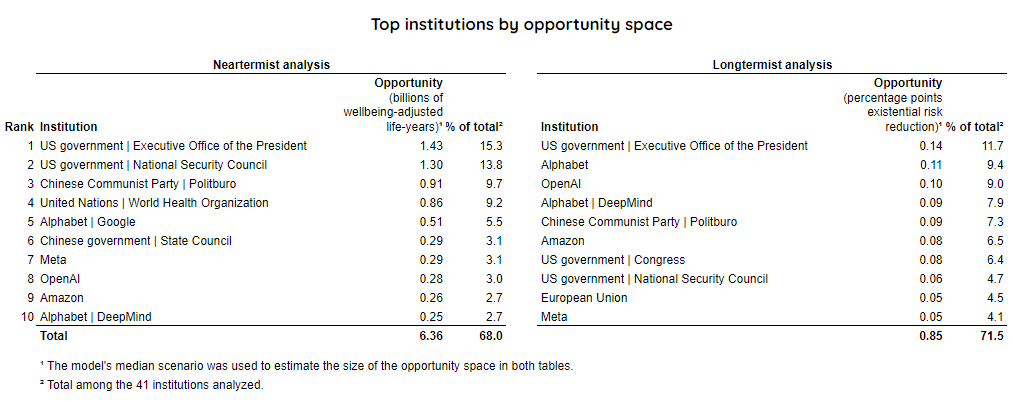
Overall top institutions
Seven institutions achieved top-10 rankings in both analyses: Amazon, the Chinese Communist Party’s Politburo, DeepMind, Meta, OpenAI, the Executive Office of the US President, and the US National Security Council. The synopses below have been condensed from the full profiles we developed for each institution.
Amazon
Amazon is the world’s largest online retailer, with a market cap of $1.6 trillion and more than $450 billion in annual revenue. In addition to its flagship store, it also produces and sells products such as the Alexa personal assistant and ecosystem, Kindle e-reader, Audible platform, Fire TV, Whole Foods Markets, and movies and television shows through its Amazon Prime Video platform. Amazon is also the world’s leading cloud computing provider through Amazon Web Services.
As Amazon has grown, it has become increasingly woven into the fabric of daily life, with millions of users relying on the company for fast deliveries and access to hard-to-find products. The company and its workers were a lifeline for many during the heights of the COVID-19 pandemic when other retail options were shut down, and the company’s revenues boomed accordingly. Similarly, Amazon’s dominance in the cloud computing market means that sustained or ill-timed disruptions to its infrastructure could have widespread impacts on the economy and possibly interfere with the provision of essential services; it also gives Amazon the power to “deplatform” actors it considers to be violating its terms of service. Meanwhile, Amazon’s relentless focus on building and leveraging economies of scale have had deep impacts on the structure and business practices of the industries in which it operates, both for better and for worse.
Amazon has long embraced new technologies melding the digital and analog worlds such as drone delivery, and there are good reasons to believe it will be among the major players in AI development and business applications. Despite that, the effective altruism community and other tech-forward institutional reformers do not seem to have as much of a presence in the Amazon ecosystem as other large tech companies, making Amazon perhaps a prime growth market.
The Politburo of the Chinese Communist Party
The Chinese Communist Party is unquestionably one of the most consequential institutions in the world, exercising near-total control over both internal policy and foreign affairs for a country of 1.4 billion people with increasing ambitions for global leadership. The CCP’s Politburo, and in particular the seven-member Politburo Standing Committee, is arguably the most important of several centers of gravity for the Chinese state and is the principal entity crafting high-level policy and strategy for the country. The Politburo represents the different power bases and factions within the CCP. Its members derive their power from their senior positions in the Party, security apparatus, military, and important regional governments. In addition, the Politburo formally controls the operations of the CCP and makes promotion decisions for middle and senior managers across all state- and party-controlled institutions, giving it enormous influence. Xi Jinping, as General Secretary of the CCP, is a member of the Politburo Standing Committee and is functionally the ultimate authority in all government decision-making.
Because the Politburo has limited staff capacity available in some policy areas to manage such a broad remit, it often seeks advice and input from think tanks (which are usually state-operated) and academics within China. This avenue of information flow provides an extremely narrow but potentially viable pathway for engaging with the Chinese party-state. The most promising arenas for progress are likely to be those such as climate change, biorisk, and AI safety where the welfare of Chinese citizens is clearly at stake from the perspective of their government. In general, we expect that domestic institutions and citizens in China would be in a better position to pursue institutional improvements within the country.
DeepMind
DeepMind is one of the world’s leading artificial intelligence research laboratories. Originally founded in 2010, it was acquired by Google in 2014 and is now a direct subsidiary of Google’s parent company, Alphabet. DeepMind is best known for developing AlphaZero, a neural network that taught itself the complex game of Go from scratch and eventually became the first computer program to beat a Go world champion. More recently, DeepMind solved a 50-year-old protein folding challenge, an advance expected to accelerate progress in biological research. Breakthroughs like these are one reason why DeepMind ranks highly even in our 10-year time horizon; the pace of new discoveries is such that it is not hard to imagine DeepMind technology underpinning hugely consequential and as-yet-unforeseen applications as soon as 2030.
DeepMind is one of two major AI research labs (the other being OpenAI, below) explicitly working towards “artificial general intelligence” – an AI system that can perform intellectual tasks at least as well as human beings across multiple domains. This phenomenon would be the most powerful technology ever created and could easily outpace humans’ ability to control it if not properly designed. DeepMind has therefore, in collaboration with a small field of researchers working in the field of AI safety, been working to incorporate ethical and practical safeguards into its products. Many people involved with the effective altruism community (which has long treated AI safety as a top priority) work at or are involved with DeepMind, most notably Allan Dafoe who leads DeepMind’s Long-Term Strategy and Governance team and was until recently the head of the Centre for the Governance of AI.
DeepMind has largely been allowed to maintain its decision-making independence since the Google acquisition. In 2018, however, Google took direct control of DeepMind’s health division, and in May 2021 reports suggested that ethical oversight of DeepMind’s current and future AI research will now be the responsibility of Google’s Advanced Technology Review Council, as opposed to DeepMind’s own board of ethics. The accepted narrative is that Google wants DeepMind to focus more on commercial applications of its research, and broaden its research focus beyond deep learning neural networks to other forms of AI. Productive influence at DeepMind, therefore, likely looks like ensuring that the attention it pays to ethics and safety continue to influence product decisions at a high level of fidelity and do not become simply a public relations fig leaf.
Meta
Meta (until recently known as Facebook, Inc.) is the world’s largest social network. Across its various platforms, including WhatsApp, Instagram, and Messenger in addition to Facebook, Meta had 3.5 billion active users as of the third quarter of 2021. The company has also invested heavily in virtual and augmented reality technologies, most notably the Oculus VR headset, and recently rebranded in a play to dominate the so-called “metaverse.”
Meta’s impact on the world runs primarily through the network effects it has created by amassing such a huge user base. Its users leverage the company’s platforms to connect, share information, and express themselves in a kind of digital public square, which in turn enables all sorts of offline ripple effects ranging from organizing protests to catalyzing distributed emergency response to spreading propaganda. Meta’s (usually automated) choices about what content to promote, ignore, and censor, especially from high-profile figures, thus can have significant social and geopolitical consequences. In addition, Meta has a highly competitive AI division called Facebook AI Research (FAIR).
More than some of the other tech companies on the list, Meta/Facebook has attracted intense scrutiny from regulators and the public alike in response to perceived failings and social harms of its current suite of products. Its employees have organized internally to try to force change and some have taken their concerns to the media, but for the most part company leaders have sought to absorb the pressure as a cost of doing business rather than chart a radically different course. Thus, while there is no dispute that Meta’s choices can carry enormous weight, it remains to be seen how much more can be done to influence how it does business that hasn’t already been tried.
OpenAI
Along with DeepMind, OpenAI is one of two major AI research labs committed explicitly to building artificial general intelligence. Founded in 2015 and with only around 250 employees, OpenAI is the youngest and smallest institution on our list. It is best known for the GPT series of language models, including GPT-3, Codex, and InstructGPT.
The case for impact at OpenAI is very similar to the case for DeepMind. While OpenAI LP is structured as a for-profit entity, OpenAI was originally launched as a nonprofit and the company is still owned (and ostensibly governed) by the nonprofit entity OpenAI Inc. Open Philanthropy, the EA-affiliated philanthropic vehicle of Dustin Moskovitz and Cari Tuna, supported OpenAI with an at-the-time-unprecedented grant of $30 million that led to Open Philanthropy CEO Holden Karnofsky serving on OpenAI’s board of directors. (Karnofsky has since left the board, replaced by former Open Phil staffer Helen Toner who is now director of strategy for Georgetown University’s Center for Security and Emerging Technology.)
More recently, however, the trajectory of the company has become less clear and more controversial. In the past couple of years, OpenAI has focused increasingly on AI capabilities research as opposed to AI safety research. Producing elite capabilities research could be helpful for achieving the primary goal of developing aligned AGI, but there are concerns among the AI safety community that the focus has shifted too far in favor of capabilities, which is reflected in the fact that a number of OpenAI’s leading AI safety researchers left the organization in 2020-21 to form the new entity Anthropic.[7] If sector-wide capabilities research proceeds at a faster rate than safety research, the risk of misaligned AGI may increase.
As with DeepMind, OpenAI perhaps counterintuitively appears on our neartermist top institution list as well due to expectations that its products will be widely deployed in impactful settings within ten years’ time, as well as the perception that its small size and altruistic roots will make it relatively more open to feedback and necessary course adjustments than many of the more established institutions on our list.
The Executive Office of the President of the United States
In the words of one of our survey respondents, “US executive authority is awe inspiring.” The president of the United States is commander-in-chief of the world’s most powerful military and has the ability to deploy armed forces unilaterally in emergency situations. Domestically, while it’s the role of Congress to pass major legislation, the president can issue executive orders covering a wide range of policy issues, including immigration, civil rights, and the structure of the federal government. The president nominates executive branch leaders and judges to be confirmed by Congress, and leaders of executive branch agencies report to the president. The president also negotiates treaties and other agreements with other countries and sets the agenda for US foreign policy. In a crisis scenario, the president can invoke emergency powers with potentially breathtaking scope.
The Executive Office of the President is a rather large segment of the executive branch that focuses on advising the president and overseeing the vast machinery of the federal bureaucracy. It includes the White House Office, the Office of Management and Budget, the National Security Council, the Council of Economic Advisers, the Office of Science and Technology Policy, and several other agencies.
For such a powerful institution, the Executive Office of the President is capable of shifting both its structure and priorities with unusual ease. Every US President comes into the office with wide discretion over how to set their agenda and select their closest advisors. Since these appointments are typically network-driven, positioning oneself to be either selected as or asked to recommend a senior advisor in the administration can be a very high-impact career track. An even more straightforward pathway to influencing the Executive Office of the President, at least for American citizens, is via the electoral process – the last two presidential contests were each decided by fewer than 80,000 votes spread across several key states.
The United States National Security Council
The United States spends well over $1 trillion every year on national security and foreign policy, projecting power and shaping world affairs in every corner of the globe via nearly 750 military bases and more than 250 embassies and consulates worldwide. Arguably, the single most consequential institution shaping and directing these activities is the National Security Council. Originally devised as a coordinating body bringing together perspectives from the Departments of Defense, State, Treasury, Homeland Security, and various intelligence agencies across government, the NSC has evolved into an independent power base led by the National Security Advisor. It is technically a subdivision of the Executive Office of the President, but thanks to its touchpoints with other Cabinet agencies, National Security Council meetings provide the primary decision-making venue for the President’s national security and foreign policy agenda. It is the only forum where all relevant actors in the national security space meet and the President is presented with a range of policy options.
The NSC is a key crisis response actor, and not just in arenas that fall under traditional definitions of “national security” – for example, the agency has an increasing biosecurity portfolio and led the US response to the Ebola outbreak in 2014-16 as well as COVID-19 after the Vice President’s pandemic response team was disbanded in 2020. The staff of the NSC is relatively small – in the low hundreds of people – but it meets virtually continuously, shaping and directing the work of the massive US federal national security apparatus. Indeed, one of the reasons the NSC scores highly on our metrics is that it seems to combine immense access to authority and resources with a degree of flexibility that is unusual among major institutions. It’s not hard to imagine that the NSC might add portfolios for AI risk, nanotechnologies, and other existential threats in the near future; already, a special envoy for climate change has joined the mix. And because of the NSC’s positioning as a forum for interagency coordination and mandate for threat horizon scanning, planning and risk mitigation work that it engages in during “quiet” years could yield vast dividends during future crises.
Additional top institutions from the perspective of global health and wellbeing
Institutions that were relatively more important from a neartermist vs. a longtermist lens included Google, the State Council of China, and the World Health Organization.
From its humble roots as an upstart search engine in 1998, Google has grown to become one of the world’s largest technology companies, with a market share of over 91% in online search and a kaleidoscope of integrated apps, products, and services including GSuite, Google Maps, Google Chrome, YouTube, Android, various hardware products, cloud computing, and its AI division Google Brain.
Multiple Google products have billions of active users, giving the company immense sway and embeddedness into daily life and work around the world. As with other large social networks, YouTube is particularly a double-edged sword, making possible an unprecedented degree of collaboration, education, and digital documentation in the video format while also facilitating the spread of misinformation and polarizing content. Changes to Google’s search ranking and recommendation algorithms across YouTube and other products could plausibly increase or decrease quality of life for millions of people, as can the creation of new products that improve or interfere with people’s lives. Google did not rank quite as highly on our longtermist analysis because its internal AI division is not driving as explicitly toward the development of artificial general intelligence as its counterparts at some other companies, reducing (though not eliminating) the company’s relevance to x-risk scenarios. However, this could easily change, and it’s also possible that Google’s existing suite of products could indirectly impact the long-term future by helping to shape public opinion about important issues.
The State Council of the People’s Republic of China
China’s State Council is the cabinet of the People’s Republic of China and is tasked with implementing the objectives of the CCP. Led by China’s vice premier, Li Keqiang, the State Council’s importance comes both from its role in driving economic policy for the world’s second-largest economy and its position as the chief regulatory body for AI and other new technologies in China. For example, the State Council and its Ministry for Industry and Information Technology was the driving force behind Made in China 2025, which calls for increased investment in domestic semiconductor manufacturing capacity among other objectives. In an AI “crunch time” scenario or great power conflict, however, its role will likely be limited to implementing the directives of the Politburo and Secretary-General.
While the State Council made the list of top institutions primarily on the strength of its role in shaping the Chinese economy and the resulting wellbeing implications of its decision-making for Chinese residents, it is also potentially the key actor in China for preventing existential risks that are dependent on regulatory actions before they become major policy issues. More so than other Chinese institutions, the State Council actively seeks out expert input, sometimes convening “national teams” around the pursuit of its stated goals that can include representatives from universities and think tanks. On some issues such as climate change, agencies under the purview of the State Council have even worked with Western development consultants. This is another narrow pathway to impact that is worth investigating further, though at this point we expect that domestic institutions and citizens within China would be best positioned to pursue institutional improvements in their own country.
World Health Organization
The World Health Organization is a United Nations agency that connects nations, countries, partners, and people to promote health worldwide and serve the vulnerable. It leads global efforts to expand universal health coverage as well as direct and coordinate the world’s response to health emergencies. Its work encompasses both communicable and non-communicable diseases, including neglected tropical diseases such as malaria, and WHO also works on environmental health hazards like polluted air and chronic exposure to noxious substances.
Key to WHO’s importance is its direct relationships with national health officials in more than 150 countries, for whom it helps set medical guidelines and provides resources to monitor diseases and health systems. While WHO would be a crucial player in preventing and/or fighting a future pandemic, because overall estimates of existential risk from pandemics in Aird’s database were quite a bit lower in magnitude than those from other risks, its importance in that domain was not enough to elevate it to the top ranks of institutions for reducing x-risks overall. There is plenty of scope for mitigating catastrophic pandemics and other health challenges that fall short of extinction, however, and the importance of those do show up in our near-term rankings.
At the moment, WHO relies heavily on both government and private donors such as the Bill and Melinda Gates Foundation for its funding, with much of the budget restricted for specific causes and activities. With the COVID-19 pandemic having raised the salience of international efforts to coordinate pandemic preparedness and response, the next few years could offer a favorable window in which to try to pursue management improvements at the organization and bolster its capacity for the future.
Additional top institutions from the perspective of reducing existential risk
Institutions that were substantially more important from a longtermist vs. a neartermist lens included Alphabet (Google and DeepMind’s parent organization), the European Union, and the US Congress.
Alphabet
Alphabet is a holding company that contains about 400 direct and indirect subsidiaries, most of the latter of which are direct subsidiaries of Google LLC. Alphabet is currently the world’s third largest public corporation by market capitalization. Besides Google and DeepMind, other notable Alphabet companies include Calico (aging research), Loon (rural internet access), Waymo (autonomous driving technology), Wing (drone delivery) and X Development (a “moonshot factory” working on “solving the world’s hardest problems using technology.”)
Alphabet was spun out of Google as part of a business restructuring in 2015, and its roots in its child company are still very much in evidence. Sundar Pichai, the CEO of Google, is also the CEO of Alphabet, and Google co-founders Sergei Brin and Larry Page still hold a controlling interest in the company. Right now, there is not a lot of evidence that important decisions are made at the level of Alphabet itself beyond mergers and acquisitions. However, as AI and potentially other technologies involving Alphabet companies (DeepMind foremost among them) move closer to assuming genuine geopolitical importance, Alphabet’s formal governance structures could become increasingly pivotal.
The European Union
The European Union is a unique economic and political intergovernmental body with 27 European member nations. Governed by a set of treaties, the EU has most of the elements of a typical government – an executive, a parliament, a court system – and has steadily increased its scope and powers since its founding in 1951.
One reason for the EU’s high ranking is that it already places more emphasis on resilience in the face of catastrophic risks (along with global health and wellbeing) than most large government entities, which perhaps stems from its roots as a confederation of many countries. The EU is known for piloting innovative policies which then have ripple effects around the world, a phenomenon known as the Brussels effect; the global rollout of the GDPR data privacy regulations has been a particularly dramatic example. The EU has taken a leadership role in international climate negotiations and adopted the ambitious European Green Deal seeking to make the bloc climate-neutral by 2050.
There has already been quite a bit of writing about the EU’s relevance to global AI governance, and there is legislation in active development now that would be the first government policy to address general purpose AI systems. Because of the Brussels effect and other ways in which EU policies can influence state and corporate actors, the EU could be influential on the world stage even though it is not a major military power or home to any leading research labs pursuing AGI. The same logic applies to other existential risks that would benefit from global coordinated action, such as pandemic preparedness and threats from nanotechnology.
United States Congress
As the legislative branch of the US government, Congress has enormous potential to shape lives and circumstances not only in the US but around the world. On a routine basis, it sets the annual budget allocations for the federal government, including defense authorizations, aid budgets, research budgets, and more. The Senate confirms appointees to lead all executive branch agencies and serve as judges in the nation’s federal courts. In addition, Congress has the ability to pass, modify, and repeal legislation affecting nearly every aspect of human and animal life.
As jaw-dropping as Congress’s powers are in theory, however, it rarely gets an opportunity to fully exercise them. Because of numerous veto points in the US federal system along with procedural bottlenecks and political polarization, Congress these days often struggles to accomplish basic tasks in a given session such as funding the federal government for more than a few months at a time and preventing the country from defaulting on its debt. Because issues relating to existential risks are less politicized than many others, however, we still believe there may be opportunity for productive engagement on that front. For example, forming a “future generations” caucus in Congress focused on preventing catastrophic and existential risks, modeled after similar work in the UK, could be a productive pathway toward fostering cross-partisan consensus on critically important issues. We are also starting to see candidates running for office with these issues on their platforms.
Insights and Takeaways
Several intriguing patterns are apparent from the analysis that help to address or provide more context for our research questions.
What’s more important: an institution’s impact or its ability to be influenced?
Our analysis considered the intersection between an institution’s scope of impact and its potential for change (as well as how much of that change would be counterfactual). By contrast, the survey simply asked which institutions were “among the most powerful or influential in the world.” Of the 13 institutions that ranked highest in either version of our analysis, eight were also among those that were mentioned most often by survey responses, and several of the others were close cousins to those that did make the list or were in the next tier below. The high degree of overlap between the two lists suggests that it makes sense to center conversations about improving institutional decision-making around the most powerful institutions more so than those that seem the most readily accessible, at least initially. In practice, we do expect that robust and well-designed initiatives focused on second- or third-tier institutions will sometimes yield higher expected impact than a more difficult strategy targeting one of the top institutions on our list. But at this stage, a deeper investigation of opportunities to make positive shifts in specific, high-impact institutional contexts is likely to be a very worthwhile use of time and resources.
Neartermist vs. longtermist perspectives on improving institutional decision-making
A surprising feature of our analysis is the degree of convergence among top institutions yielded by the so-called “neartermist” perspective, which is to say the impact of wellbeing-adjusted life years over the next decade, and the “longermist” perspective, which looks at existential threats to humanity over the next century. Methodological choices account for at least some of this effect: for example, we incorporated estimates of each institution’s importance in an “extreme” scenario, which for some institutions did involve x-risks or so-called global catastrophic risks (i.e., events that cause widespread and severe but ultimately temporary devastation). In addition, we gave institutions credit on an annual basis for planning actions they could take to improve their performance in a relevant “extreme” scenario, again raising the salience of x-risks. However, it’s striking that even our lower-bound estimates gave top rankings to many of the same institutions, including the US presidency, Google, Meta, and the CCP Politburo.
One institution that is relevant specifically from an “everyday” perspective (i.e., not accounting for extreme scenarios at all) is the African Union. Under these constraints, the AU achieves a high score on the strength of its large scope (1.3 billion people live in Africa, which is also the fastest-growing continent) and the fact that the institution is still in the process of being built out.
It’s also worth keeping in mind that many of the institutions on our list are quite large and multifaceted, and interventions to improve their decision-making might look different through a neartermist vs. longtermist lens. For example, a neartermist might be particularly interested in influencing Meta’s policies around content moderation and recommendation algorithms to minimize their negative impacts on mental health, while a longtermist would likely be more interested in the activities of its AI research team.
The near-term relevance of tech and AI
Of the seven institutions that achieved top-tier status on both lists, nearly all would be expected to play a central role in the development and governance of transformative AI. This was the dominant factor in shaping the longtermist rankings, but AI powerhouses were well represented on the neartermist list as well. It’s important not to draw too simplistic a conclusion from this, however, because the large, diversified tech companies that appeared in our neartermist rankings scored highly as much because of their current product suites and logistics capabilities as their investment in advanced AI technology.
A related observation is that many of the top institutions are quite young: six of them, all tech companies, are fewer than 30 years old, and OpenAI was founded just over six years ago. Ostensibly, our framework considers the amount by which conscious efforts to improve these institutions could contribute to existential risk reduction over the next 100 years, but it’s worth keeping in mind that any or all of them could be long gone or greatly diminished in importance by the end of that period. Our hope is that future iterations of this work will more fully explore the dynamics of how institutions, including new institutions, rapidly gain or lose importance on the world stage.
A new theory of change for institutional reform
Most existing institutional improvement strategies can be classified into a few categories based on their theory of change:
- “Issue-centered” theories of change specialize in a particular cause area such as climate change or nuclear security, and seek to engage institutions selectively in order to make progress on that issue. Practitioners of this model possess a robust command of policy details related to their specialty, but because they are seen as representing a narrow interest, they sometimes struggle to develop trusted relationships with decision-makers whose authority is broader in scope than the particular issue in question.
- “Audience-centered” theories of change work across issue areas but specialize in a target audience, e.g. policymakers in one country. In the best-case scenario, practitioners of this model can exert tremendous cross-cutting influence in their chosen context, but their relevance is greatly diminished outside of it. The uncoordinated nature of the distribution of institutional reformers with audience-centered theories of change thus leaves critical gaps among some audiences whose decisions carry enormous influence.
- “Product-centered” theories of change have identified a tool, service, or strategy that practitioners believe will make all institutions more effective, and focus on promulgating adoption of that intervention as widely as possible. Forecasting and prediction markets fall into this category alongside various voting reforms, anti-corruption efforts, transparency initiatives, and more. The interventions in these theories of change are often genuinely good ideas that make a reliably positive impact when adopted. However, the pursuit of product-centered theories of change is often strongly shaped by market dynamics, with adoption driven as much by the strength of client interest as the potential for impact. In addition, they are not always the best solution for complex institutions that may have a wide range of dysfunctions to contend with.
While not necessarily challenging the case for the above approaches, the key findings of our analysis – a high concentration of impact opportunities among a small number of largely unrelated institutions, along with significant convergence between neartermist and longtermist perspectives on impact – strongly supports our hypothesis that a fourth, “system-centered” theory of change would be a valuable addition to the portfolio. This approach, which we believe has few if any precedents, seeks out and prioritizes the highest-impact opportunities without artificial boundaries on scope related to issues, audiences, or interventions. It works best in combination with the theories of change above, as it benefits from their respective specializations but is well positioned to address each of their weaknesses. EIP will be further exploring the potential of this new model in the coming year as we continue our work to establish a global observatory for institutional improvement opportunities.
Caveats and Limitations
This was a first attempt to answer a research question of vast complexity, conducted out of necessity with very limited resources. As a result, it is virtually certain that there are errors and gaps in our analysis, some of which may turn out to be quite important. All conclusions put forward here should therefore be regarded as tentative and subject to revision as we learn more. We expect to have more confidence in the robustness of future iterations of this work, although there will always be a significant degree of uncertainty.
The impact metrics discussed in the “Top Institutions” section represent something like an upper bound on the size of the opportunity space associated with those institutions; we should expect that any specific interventions or campaigns would only be able to capture some of this upside in practice. While the analysis does consider the expected counterfactual efforts to improve these institutions, both externally and from within, it does not take into account the detailed contours of specific campaigns or the risks of accidental harm from those campaigns. In our previous article, we developed a “specific-strategy” version of our prioritization framework that can take these elements into account when considering a concrete opportunity to influence a particular institution. Accordingly, our listing of top institutions should not be treated as a recommendation to immediately start work on improving them. Instead, our immediate recommendation is to invest in deepening knowledge about these institutions in order to determine what, if any, strategies might yield robustly positive outcomes, and at what cost.
There are certain methodological quirks and limitations to be aware of when interpreting our findings. First, the practice of translating institutional decisions into our chosen impact metrics required many judgment calls, including what heuristics to use when making judgments about the nature and degree of an institution’s impact on people’s lives, how to apportion responsibility for world events across institutions that might be subsets of one another or make decisions jointly, and how much credit to give institutions that primarily serve as convening bodies for more-powerful actors. We also faced difficult questions like what baseline expectation we should have about how much a typical large institution’s decision-making can be influenced by a well-designed and -executed strategy with altruistic aims. Since it wasn’t feasible for us to fully resolve competing priors about these questions in the time available, we focused less on getting the numbers “right” in an absolute sense and more on ensuring that the relative rankings across different criteria made sense and were defensible given what we knew about each institution.
Finally, because the estimates of risks from artificial intelligence in Aird’s database of existential risk estimates were generally much higher than those for other x-risks, organizations involved in AI safety and governance ended up dominating our longtermist rankings. If the risk estimate database oversampled from researchers who are unusually pessimistic about AI safety, that would have in turn affected our results.
Next Steps
The findings of this pilot analysis will serve as a roadmap for Effective Institutions Project’s continued landscape analysis work in 2022. We plan to start deepening our understanding of a subset of the institutions highlighted above, driving toward concrete funding strategies and career tracks that are likely to present significant opportunities to make the world a better place. We will work in close collaboration with researchers, advisors, and others knowledgeable about the relevant institutions to seek actionable intelligence on both existing aligned partners whose efforts could use additional support and new initiatives that could be transformative if brought into being. For example, some speculative strategies/outcomes that could be both feasible and plausibly high-impact based on our research might include:
- Creation of a cross-partisan "future generations caucus" in the US Congress focused on existential risks, modeled after similar work in the UK Parliament
- Ensuring that Alphabet's corporate board of directors is well-educated about AI safety issues
- Helping the World Health Organization implement the recommendations for strengthening global preparedness for future pandemics from the WHO’s independent evaluation of its response to COVID-19
- Facilitating the creation of a unit focused on existential risks from novel technologies within the US National Security Council
We also intend to expand the number of institutions captured by our analysis and the quality of that analysis, increasing the number of institutional profiles that merit “Robust” and “Enhanced” designations and drafting new profiles of previously uninvestigated institutions. Doing so will help us avoid accidental harms from misjudging the practical and political contexts of our target institutions and ensure that we are not accidentally focusing on too narrow a set of pathways to make a difference.
Finally, we plan to conduct a second (and more robust) iteration of this analysis in winter 2022-23. Next time around, we will not only incorporate new information gained throughout the year, but also apply a number of methodological improvements aimed at improving the precision and confidence of our judgments. Similarly to GiveWell’s top charities list or 80,000 Hours’s top career tracks, we see our top institutions list as a living document that will get more useful and trustworthy over time as we are able to invest more effort into it.
If you would like to support future iterations of this project, we gladly welcome both donations and volunteers; contact info@effectiveinstitutionsproject.org to learn more.
Acknowledgements
This research project has benefited from the contributions of many individuals. Ian David Moss served as overall project director and lead author of the final report. Rassin Lababidi made major contributions to the long list and helped administer and analyze the expert survey. Angela María Aristizábal, Jan-Willem van Putten, and Abeba Taddese made additional contributions to the long list. Ṣẹ̀yẹ Abímbọ́lá, Daniel Ajudeonu, Norman Bradburn, Frances Brown, Barry Eye, Jakob Grabaak, Laura Green, Bruce Jones, Ruth Levine, Nancy Lindborg, Dylan Matthews, Clara Miller, Luke Muelhauser, Dave Orr, Dana Priest, Roya Rahmani, John-Arne Røttingen, and Rumtin Sepasspour provided names of experts to contact about the survey. Ahmed Yusuf led the survey analysis. Joseph Levine analyzed the list of commissions, which had been provided to us by John Lavis. Daniel Ajudeonu, Nick Anyos, Nathan Barnard, Merlin Herrick, Deborah Abramson Kroll, Rassin Lababidi, Joseph Levine, Arran McCutcheon, Ian David Moss, Aman Patel, Jonathan Schmidt, Ana-Denisse Sevastian, and Ahmed Yusuf researched and drafted profiles of individual institutions on our short list, while Jacob Arbeid, Katriel Friedman, Dan Spokojny, and Jenny Xiao served as expert reviewers. Emil Iftekhar, Matt Lerner, Ian David Moss, and Sam Nolan built quantitative estimates of the impact potential of engaging with each institution based on the completed profiles and our prioritization framework. Michael Aird, Shahar Avin, Max Daniel, Gaia Dempsey, Ozzie Gooen, Fynn Heide, Emil Iftekhar, Deborah Abramson Kroll, Luke Muehlhauser, Dan Spokojny, Peter Wildeford, and Jenny Xiao provided feedback on various drafts of this document. The Effective Altruism Infrastructure Fund, David and Lucile Packard Foundation, and private donors contributed financial support.
- ^
There are some instances of subdivisions of institutions coexisting on the list alongside their parent bodies. Whenever this happened, we tried our best to avoid double-counting by only giving the parent organization credit for its direct ability to influence the subdivision, e.g., by choosing its leader.
- ^
All institutions nominated at least twice by survey respondents or that were associated with at least four global commissions were included in the short list. We supplemented these with a few that did not meet either criterion but nevertheless seemed important to include.
- ^
For benchmarking purposes, we imagined a $100 million budget allocation for each institution with a deeply researched strategy tailored to the institution’s specific context.
- ^
For an example calculation, see the case studies included in the previously-published framework document.
- ^
Please see the “Caveats and Limitations” section for important context on these numbers and reasons not to take them too literally.
- ^
For reference, our lower-bound estimate of the opportunity space for these institutions is about 900 million WELLBYs over 10 years. At $100M per institution, this would work out to be about 20x more cost-effective than GiveWell’s current top charities and 200x more cost-effective than cash transfers.
- ^
OpenAI does still have its own AI safety team, which is currently led by Jan Leike.
MathiasKB @ 2022-03-21T09:32 (+32)
Can you comment on why you chose not to release the quantitative model and calculations that you used to derive these conclusions? As detailed as this work is, I don't feel comfortable updating my views based on calculations I can't see for myself.
As you point out in the post, I imagine there is huge variability based on various guesstimates (as there should be!). To me at least, the most valuable part of this work lies in the model and the better understanding we get from attempting to make models, rather than the conclusions of the model.
IanDavidMoss @ 2022-03-21T12:35 (+16)
Mathias, I'm happy to share the full spreadsheet with you or anyone else on request -- just PM me for the link. In addition, anyone can see the basic structure of the model as well as two examples of working through it in our previous piece introducing the framework we used.
Making the model public opens up the possibility of it being shared without the context offered in this article, and I'm hesitant to do that before we have an opportunity to document it much more fully. My hope is that we'll be able to do that for the next iteration.
EDIT: I've decided to just let people see it for themselves since the interest in this is stronger than I thought it would be.
I'd emphasize that there's a lot of information in the article that doesn't rely on the model at all -- e.g., the expert survey results, the details about specific institutions, etc. So I don't think whether you trust the numbers or not is all that big of a crux.
Pablo @ 2022-03-21T12:46 (+30)
Since the model can't yet be shared publicly, it would be valuable, I think, to have an external person from the community with experience evaluating projects (e.g. Nuño Sempere) take a closer look at it and share their impressions.
Nathan Young @ 2022-03-21T15:19 (+3)
Is there any way you would have felt more comfortable releasing the model? Say if the EAIF supported it, or if it was visible to logged in users only?
Charlie Dougherty @ 2022-03-21T09:14 (+21)
It is an interesting analysis, but how do you propose to have any influence over these institutions? For example, how would you go about,"Ensuring that Alphabet's corporate board of directors is well-educated about AI safety issues"? How would you influence Amazon? What would be the intention of the intervention? How would you influence the office of the President of the USA? The importance of these "institutions" seems self-evident, but what you would actually do to change things, and what exactly you would want to change, seem to be more salient questions.
Another question is if you comparing apples to apples when comparing Amazon to Congress. What makes them both institutions, and what is useful about creating a category that includes both of them? Would you use the same interventions?
howdoyousay? @ 2022-03-21T14:33 (+8)
I'm replying quickly to this as my questions closely align with the above to save the authors two responses; but admittedly I haven't read this in full yet.
Next, we conducted research and developed 3-5-page profiles on 41 institutions. Each profile covered the institution’s organizational structure, expected or hypothetical impact on people’s lives in both typical and extreme scenarios, future trajectory, and capacity for change.
Can you explain more about 'capacity for change' and what exactly that entailed in the write-ups? I ask because looking at the final top institutions and reading their descriptions, it feels like the main leverage is driven by 'expected of hypothetical impact on people's lives in both typical and extreme scenarios', and less by 'capacity for change'.
It seems to be a given that EAs working in one of these institutions (e.g. DeepMind) or concrete channels to influence (e.g. thinktanks to the CCP Politburo) constitute 'capacity for change' within the organisation, but I would argue that that in fact is driven by a plethora of internal and external factors to the organisation. External might be market forces driving an organisations dominance and threatening its decline (e.g. Amazon), and internal forces like culture and systems (e.g. Facebook / Meta's resistance to employee action). In fact, the latter example really challenges why this organisation would be in the top institutions if 'capacity for change' has been well developed.
For such a powerful institution, the Executive Office of the President is capable of shifting both its structure and priorities with unusual ease. Every US President comes into the office with wide discretion over how to set their agenda and select their closest advisors. Since these appointments are typically network-driven, positioning oneself to be either selected as or asked to recommend a senior advisor in the administration can be a very high-impact career track.
Equally, when it comes to capacity for change this is both a point in favour and against, as such structure and priorities are by definition not robust / easily changed by the next administration.
Basically, it's really hard to get a sense of whether the analysis captured these broader concerns from the write-up above. If it didn't, I would hope this would be a next step in the analysis as it would be hugely useful and also add a huge deal more novel insights both from a research perspective and in terms of taking action.
Also curious about how heavy this is weighted towards AI institutions - and I work in the field of AI governance so I'm not a sceptic. Does this potentially tell us anything about the methodology chosen, or experts enlisted?
EDIT: additional point around Executive Office of the President of US
IanDavidMoss @ 2022-03-21T16:39 (+5)
Yep, I agree, this is complex stuff. The specifics on what interventions might be most promising to support were largely out of scope for this preliminary analysis -- this is all part of a larger arc of work and those elements will come later this year (see diagram below, with apologies for the janky graphics).
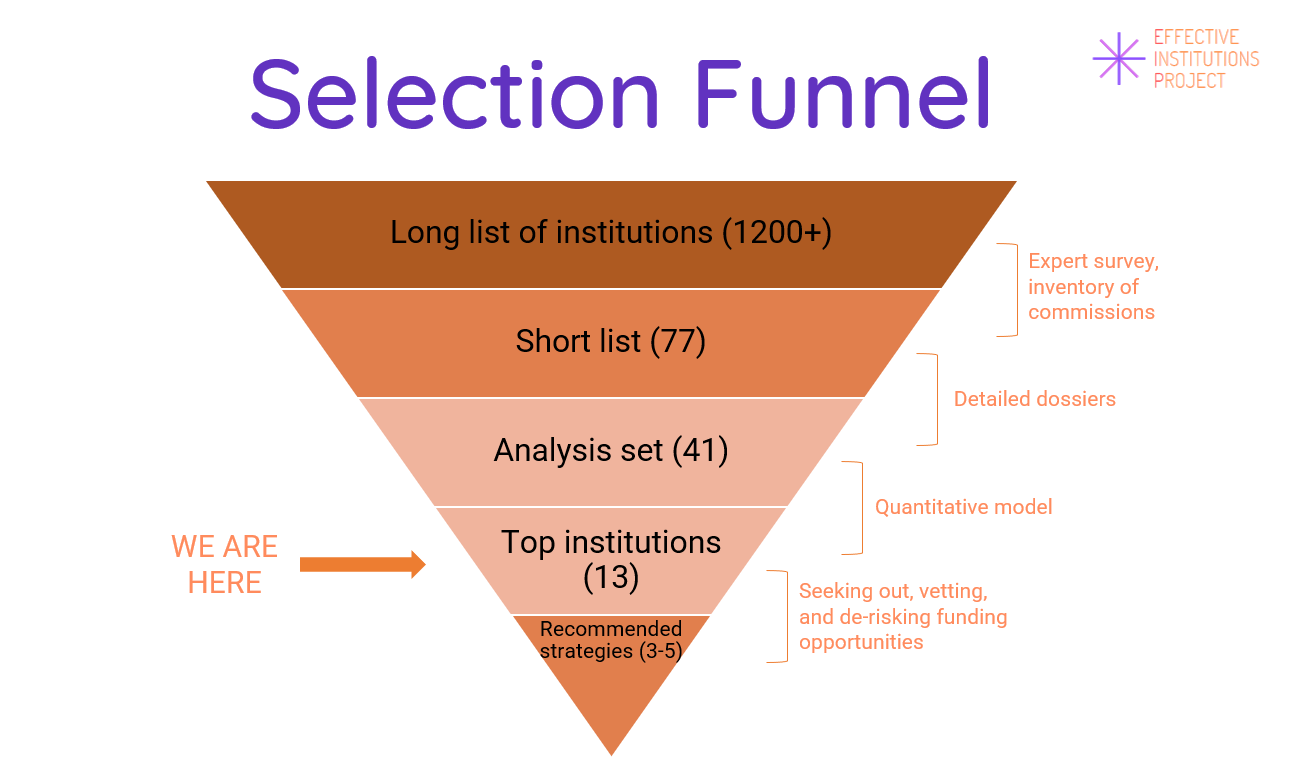
I would offer a few points that I think are worth keeping in mind when considering questions like these:
- Institutional improvement opportunities are highly contingent. As you pointed out, lots of internal and external factors drive an institution's capacity for change. Often it's easiest to drive change when lots of other changes need to happen anyway; I see Jan Kulveit's sequence on practical longtermism during the COVID pandemic as an illustration of this. One implication is that, absent a very high degree of clarity about what's going on with an organization (see point #2 below), it probably makes more sense for people interested in this space to prioritize developing general capacities that can be useful in a number of different situations rather than jump to bets on very specific pathways to impact, as the latter can easily be upset by a changing landscape.
- Judging institutional tractability usually requires insider knowledge: One of the general capacities I think is particularly valuable in these situations is developing a detailed understanding of what's happening inside an institution. To take the example of the employee activism at Meta, you're right that it's had little visible impact so far, but it's hard to know from the outside if that means that path is just hopeless and we should try something else, or if alternatively it's changed the circumstances surrounding the organization quite a bit and greatly increased the success probability of future interventions. I think the only way you would be able to judge this with precision is to get to know an organization like that really, really well, and that takes a lot more time on a per-organization basis than we've invested to date. One of the main purposes of this article was to help us decide which organizations are worth that investment. For this same reason, to echo Nathan's point below, the estimates of tractability and neglectedness for most institutions on our list are pretty fuzzy because we don't have that level of inside knowledge for most of them. But the nature of estimating things quantitatively is that you do the best you can with the information you do have and communicate your remaining uncertainties honestly, and that's what we tried to do here.
- Institutions are multifaceted: Most of the top institutions on our list have many different avenues for impacting people's lives. The US presidency will indeed be among the most important players in the AI governance conversation, but it's among the central actors on a host of other policy issues and cause areas as well. So I wouldn't over-update on the intersection between AI heavyweights and this list; with the exception of OpenAI and DeepMind, my personal takeaway is more in the direction of "institutions that are powerful anyway will also be really important to AI governance" more than "the only institutional improvement conversations worth having are about AI governance."
howdoyousay? @ 2022-03-29T08:11 (+12)
I will be completely honest and share that I downvoted this response as I personally felt it was more defensive than engaging with the critiques, and didn't engage with specific points that were asked - for example, capacity for change. That said, I recognise I'm potentially coming late to the party in sharing my critiques of the approach / method, and in that sense I feel bad about sharing them now. But usually authors are ultimately open to this input, and I suspect this group is no different :)
A few further points:
- I understand the premise of "our unit of analysis was the institutions themselves, so we could focus in on the most likely to be 'high leverage' to then gain the contextual understanding required to make a difference". I would not be surprised if the next step proves less fruitful than expected for a number of reasons, such as:
- difficult to gain access to the 'inner rings' to ascertain this knowledge on how to make impact
- the 'capacity for change' / 'neglectedness, tractability' turns out to be a significantly lower leverage point within those institutions, which potentially reinforces the point we might have made a reasonable guess at: that impact / scale can be inversely correlated with flexibility / capacity for change / tractability / etc
- I get a sense from having had a brief look at the methodology that insider knowledge from making change in these organisations could have been woven in earlier; either by talking to EAs / EA aligned types working within government or big tech companies or whatever else. This would have been useful for deciding what unit of analysis should be, or just sense-checking 'will what we produce be useful?'
- If this was part of the methodology, my apologies: it's on me for skim-reading.
- I'm a bit concerned by choosing to build a model for this, given as you say this work is highly contextual and we don't have most of this context. My main concerns are something like...:
- quant models are useful where there are known and quantifiable distinguishers between different entities, and where you have good reason to think you can:
- weight the importance of those distinguishers accordingly
- change the weights of those distinguishers as new information comes in
- but as Ian says, 'capacity for change' in highly contextual, and a critical factor in which organisations should be prioritised
- however, the piece above reads like 'capacity for change' was factored into the model. If so, how? And why now when there's lowoer info on it?
- just from a time resource perspective, models cost a lot, and sometimes are significantly less efficient than a qualitative estimate especially where things are highly contextual; so I'm keen to learn more about what drove this
- quant models are useful where there are known and quantifiable distinguishers between different entities, and where you have good reason to think you can:
This is all intended to be constructive even if challenging. I work in these kinds of contexts, so this work going well is meaningful to me, and I want to see the results as close to ground truth and actionable as possible. Admittedly, I don't consider the list of top institutions necessarily actionable as things stand or that they provide particularly new information, so I think the next step could add a lot of value.
IanDavidMoss @ 2022-03-29T13:52 (+2)
Constructive critique is always welcome! I'm sorry the previous response didn't sufficiently engage with your points. I guess the main thing I didn't address directly was your question of "were concerns like these taken into account," and the answer is "basically yes," although not to the level of detail or precision that would be ideal going forward. Some of the prompts we asked our volunteer researchers to consider included:
- How are decisions made in this institution? Who has ultimate authority? Who has practical authority?
- How and under what circumstances does this institution make changes to a) its overall priorities and b) the way that it operates? Is it possible to influence relevant subdivisions of this institution even if its overall leadership or culture is resistant to change?
- Is this institution likely to become more or less important on the world stage in the next 10 years? What about the next 100? Please note any relevant contingencies, e.g. potential mergers or splits.
FYI, the full model is now posted in my response above to MathiasKB; it sounds like it might be helpful for you to take a look if you have further questions.
Continuing on:
the 'capacity for change' / 'neglectedness, tractability' turns out to be a significantly lower leverage point within those institutions, which potentially reinforces the point we might have made a reasonable guess at: that impact / scale can be inversely correlated with flexibility / capacity for change / tractability / etc
As I mentioned in my response to weeatquince, this inverse relationship is already baked into the analysis in the sense that absent institution-specific evidence to the contrary, we assumed that a larger and more bureaucratic or socially-distant-from-EA organization would be harder to influence. I really want to emphasize that the list in the article is not just a list of the most important institutions, it is the list we came up with after we took considerations about tractability into account. Now, it is entirely possible that we underrated those concerns overall. Still, I suspect you may be overrating them -- for example, just checking my LinkedIn I find that I have seven connections in common with the current Chief of Staff to the President of the United States...and not because I have been consciously cultivating those connections, but simply because our social and professional circles are not that distant from each other. And I'm just one person: when you combine all of the networks of everyone involved in EA and everyone connected to EIP and the improving institutional decision-making community more broadly, that's a lot of potential network reach.
I get a sense from having had a brief look at the methodology that insider knowledge from making change in these organisations could have been woven in earlier; either by talking to EAs / EA aligned types working within government or big tech companies or whatever else. This would have been useful for deciding what unit of analysis should be, or just sense-checking 'will what we produce be useful?'
I'm not sure what you mean by "unit of analysis" in this context, could you give an example? In an ideal world, I think you're right that the project would have benefited from more engagement with the types of folks you're talking about. However, members of the project team did include a person working at one of the big tech companies on our list, another person working at a top consulting firm, another person who is at the World Bank, a couple of people who work for the UK government, etc. And one of our advisors chairs an external working group at the WHO. So we did have some of the kinds of access you're talking about, which I think is a decent start given that we were putting all of this together essentially on a $25k grant.
I'm a bit concerned by choosing to build a model for this, given as you say this work is highly contextual and we don't have most of this context.
Yeah, this is a totally fair observation, and I'll confess that at one point I considered ditching the model entirely and just publishing the survey results. In the end, however, I think it proved really useful to us. I'm a big believer in the principle that high uncertainty need not preclude a quantitative approach (Doug Hubbard's book How to Measure Anything is a really useful resource on this topic -- see Luke Muehlhauser's summary/review here). I personally got a lot out of fiddling with the numbers and learning how robust they were to different assumptions, and that helped give me the confidence to include it in our analysis. It's not to say that I don't expect changes to the topline takeaways once we get better information -- I do expect them -- but I'd be moderately surprised if they were so drastic that it makes this earlier analysis look completely silly in retrospect.
Charlie Dougherty @ 2022-03-22T09:32 (+9)
@IanDavidMoss, thanks for the reply. I would love if you could go a little deeper into what is an institution to you. How do you characterize it, and why is this nomenclature important? I just would like to go back to my apples to apples comparison question. My first instinct is that comparing Meta to Blackrock to the Bill and Melinda Gates foundation to the Office of the President of the USA to the CCP Central Commitee is going to create some false parallels and misunderstandings of degree of importance or possibility for change ( I will just call this power).
I would suspect that the amount of power of the President of the USA is orders of magnitude greater than the Bill and Melinda Gates foundation. So while they might be on a long list together, they are a bit like comparing our moon and the Sun. So we would have a magnitude issue.
In addition we would have a capabilites issue. The office of the President is much more powerful than Mark Zuckerberg, I would argue, but Meta can also do things that the President could only dream of. Facebook has been an incredible tool for spreading information, both for good and nefarious purposes. The US government could only wish for that ability to reach peoples' brains.
These thoughts lead me to imagine what you final recommendations will look like, and I am not sure. I suspect you will discover that you end up making very specific suggestions for different insitutions. Other than a standard 80k be flexible and build up your career capital suggestion, I think it might be difficult to give thematic recommendations that are equally useful in all of the types of organizations you tackle here.
IanDavidMoss @ 2022-03-24T20:49 (+3)
Hi Charlie, if you haven't already read the post we wrote last year introducing the prioritization framework used in this article, I recommend you do so as it goes over many of these theoretical questions in depth. In that post we offered the following characterization of an institution:
The definition of an institution is not completely standardized across disciplines or academic fields, but in this context we mean a formally organized group of people, usually delineated by some sort of legal entity (or an interconnected set of such entities) in the jurisdiction(s) in which that group operates. In tying our definition to explicit organizations, we are not including more nebulous concepts like “the media” or “the public,” even as we recognize how broader societal contexts (such as behavior norms, history, etc.) help define and constrain the choices available to institutions and the people working within them. By “key” or “powerful” institutions, we are referring in particular to institutions whose actions can significantly influence the circumstances, attitudes, beliefs, and/or behaviors of a large number of morally relevant beings.
As you can see, under this definition there is nothing particularly weird about grouping government and non-government organizations together; they are both formally organized groups of people delineated by legal entities. And even if you were to limit your analysis to, say, tech companies, you would still face the same issue of vastly divergent magnitudes and capabilities within that set of organizations and have to figure out a way to derive meaning from that. Basically, I don't disagree at all with the observations you're making, but my takeaway is "yes, and this is all the more reason why a holistic and cross-sector analysis is relevant and valuable," not "well, I guess we shouldn't bother because this is all too hard."
I suspect you will discover that you end up making very specific suggestions for different insitutions.
This has always been the plan. I've believed and argued for a long time that while institutions have some common features and problems, identifying the most actionable and promising levers for change requires a highly tailored approach. And this type of work seems to me more neglected within EA than more general, intervention-level analysis (e.g., here and here). So I think we are on the same page.
weeatquince @ 2022-03-26T16:12 (+17)
Hi Ian. Great you have done this. Prioritisation is a crucial and challenging part of EA decision making and It is great to see an exercises dedicated to openly trying to to prioritise where to focus time and attention.
I did have one question / doubt about one of your key conclusion. You said:
Our findings suggest that ... opportunities [to drive better world outcomes] are highly concentrated in a fairly small number of organizations .
However as far as I can tell you do not at any point in the post seem to justify this or give the reader a reason to believe this claim to be true. Would be great to hear your thoughts on this topic.
You conclusion could be true, but there are reasons to doubt it:
- Organisations that are more powerful and more in the public's eye are often harder to influence in the ways you want. So perhaps there are greater opportunities for impact to be found by influencing less powerful organisations.
- There are path dependencies so the best opportunities at any point in time to move towards a particular goal might not be with the most powerful institutions. If you want an institutional change adopted it might make sense to try it first at a city or state level or in a receptive country before moving to one of the institutions listed. (E.g. animal welfare cage free advocates influenced corporates before policy makers. )
- It is possible to construct stories of high impact intuitional reforms that are not within the "most powerful or influential" institutions. For example one could imagine the value of spending $100m to get university career services around the world to adopt EA/ 80k style impact focused career advise to be greater than the value of spending $100m on improving the US president's office.
The expert survey didn’t seem to address this question (focusing on listing the "most powerful or influential" institutions) and the model had some fairly speculative estimates of tractability and only looked at institutions that were already deemed the "most powerful or influential".
So yeah, curious to hear your reasons for thinking opportunities are highly concentrated and not spread very broadly.
IanDavidMoss @ 2022-03-26T23:58 (+1)
Hi Sam, the key passage was in this text from the outset of the "Top Institutions" section:
Furthermore, over 70% of the total opportunity for x-risk reduction was concentrated in the top ten of those 41 institutions, and many of those in the next tier are subdivisions, competitors, or otherwise closely related to the top-ranked entities.
While we don’t have a similar global estimate of the total wellbeing-adjusted life-years at stake in any given year, we similarly observed a level of concentration among the top ten scorers in that category of about 70%.
Granted, we are only talking about the 41 organizations that were included in the model, but having spent some time with those numbers, I would be at least somewhat surprised if the 70% figure were to go down by all that much (say, below 55%) if we expanded the analysis to our entire short list of 77 organizations, and so on and so forth.
However, I would say that my overall credence in the claim that you quoted is moderately low, which was intended to be conveyed by the use of the verb "suggest" rather than a stronger word. Probably a better way to characterize it is that it's something like a working hypothesis that I believe to be true based on this analysis but am still seeking to confirm in a larger sense.
Regarding your specific counterarguments:
- More public and powerful organizations being harder to influence -- this assumption is already taken into account in our analysis, albeit with a lot of uncertainty around the relevant variables.
- Path dependencies -- I think you are right about the general point, but I don't think it really challenges the claim about concentration of opportunity. The basic case we're talking about is when you invest in one or a few demonstration projects with lower-profile institutions, where the hope is that this will help grease the wheels for spreading the idea or intervention being demonstrated to a higher-profile institution where it matters more. I think if we're serious about that being the goal, it probably makes more sense to view those investments as part of a longer-term strategy targeting the higher-profile institution, and one could even budget for the demonstration projects as part of the same $100M (or whatever amount) philanthropic investment.
- Non-targeted institutional reforms -- yes, this is along the lines of what I called the "product-centered" theory of change in the "Insights and Takeaways" section. What I understand you to be saying is that even if I'm right that there is a significant relative concentration of reform opportunities on an institution-by-institution basis, it doesn't necessarily imply that the most promising interventions targeting specific institutions will perform better on a per-dollar cost-effectiveness basis than the most promising interventions that don't. This is a good point and I'm glad you raised it.
weeatquince @ 2022-03-27T13:54 (+6)
I still expect we would if have some disagreement on how likely it is for this concentrated opportunities hypothesis to be true.
An interesting cheap (but low veracity) test of this hypothesis that could be to list out a handful of institutions (or collections of institutions) that you think would certainly NOT be considered as the "most powerful" but might matter (E.g.: university career services, EA community institutions, the Biological Weapons Convention implementation unit, AI/tech regulatory bodies, top business schools, tech start-ups, etc, etc) and then include a few of those in your modelling exercise and see how $100m to improve institutions in those cases compares to $100m to influence the most powerful institutions.
On the "path dependencies" point you make – I largely agree. I just think there is a risk (which I am sure you are already thinking through) that if you overly focus future research on just the most powerful institutions you might miss out on finding these indirect opportunities.
evelynciara @ 2022-03-21T02:39 (+16)
...making Amazon perhaps a prime growth market.
Haha, I love that wordplay!
MaxRa @ 2022-03-21T21:35 (+6)
Really cool project, thanks for the work! I like that you tried to model x-risk reduction and also look forward to see how you concretely applied your model to the institutions here.
Random thought, but have you considered including FTX and OpenPhil as particularly influential institutions? I kinda would've shortlisted them probably.
I also wondered why the surveyed experts didn't include more longtermist EAs, since I would naively have been more interested in their takes for particularly important institutions.
IanDavidMoss @ 2022-03-24T20:16 (+6)
Open Phil was in fact on our short list but it only made it into the third priority tier (it was only mentioned by one survey respondent, I think), so we didn't get to developing a profile for it and thus it wasn't among the 41 institutions that we developed quantitative estimates for. FTX is an interesting oversight, it wasn't mentioned by anyone who took the survey although I agree it should have been. I'm somewhat skeptical (less so with FTX) that either of these would have been competitive with the 13 that we highlighted in the end, for two reasons. First, the marginal/counterfactual room for improvement is not that high with either of these funders and that would bring down our estimate of the opportunity space associated with each of them. In addition, funders are generally at least one step removed from the actual decisions that matter most, so that's another factor that would hold their score down. With that said, when we do this again I'll certainly want to include both in the analysis.
The mix of survey respondents was an intentional choice. I definitely wanted EA and longtermist voices represented (and they are - about 1/3 of the respondents had some connection to those communities), but I also felt it was really important to draw from perspectives across the broader landscape of institutions, geographies, and policy domains while ensuring that all respondents were approaching the question through a global and cross-issue lens. I'd also note that EAs are disproportionately early-career, which means that they have had fewer opportunities to experience important institutions directly from the inside and develop diverse professional networks that can give them access to relevant non-public (or just not well-known) information about those institutions. As a result I tried to balance things by inviting some very senior and retired people into the mix. I actually thought this would lead to more divergence in the survey results than we saw, but basically the EAs and non-EAs mostly picked up on the same top institutions in the end.
meraxion @ 2022-04-04T10:49 (+4)
Hi Ian. This write-up made me even more excited to follow the work of the EIP in the coming years.
One thing I wanted to ask for more information about was the "system-centered" approach you detailed towards the end of the post. I'm not sure I see entirely the difference between an approach which "seeks out and prioritizes the highest-impact opportunities without artificial boundaries on scope related to issues, audiences, or interventions." and using a mish-mash of interventions from the traditional improvement strategies.
Is the proposal that e.g. instead of coming to an organisation and saying "we think you need forecasting", there should instead be more of an open-ended analysis of their structure, needs, incentives, etc., to tailor both *which* interventions/products to implement *and* (as usually done) how to implement them? And that before even coming to an organisation you will have carried out an analysis like the one in this post, and by doing these two things, be issue-, audience-, and product-agnostic?
I am not familiar with too many examples or case studies of carrying out large changes in organization, but in an area I *am* familiar with, performance psychology, one of the large limiters on this kind of approach is resources. As a one-person operation, you don't have the capacity to play around with approaches, and have to specialize in one issue/implementation, e.g. mindfulness in athletes. Do you expect that the EIP will be structured so as to, and have the resources to, do the needs analysis of relevant institutions, and then have individually specialised, expert staff on demand to deploy as the needs analysis suggests, or that you will have general competency staff at these different levels?
I see that these issues might be things that will be considered and dealt with in due time, and if you think they are not timely for the current state of EIP, that's fine.
IanDavidMoss @ 2022-04-17T14:57 (+3)
Sorry for missing this comment earlier! Some brief responses below:
Is the proposal that e.g. instead of coming to an organisation and saying "we think you need forecasting", there should instead be more of an open-ended analysis of their structure, needs, incentives, etc., to tailor both *which* interventions/products to implement *and* (as usually done) how to implement them? And that before even coming to an organisation you will have carried out an analysis like the one in this post, and by doing these two things, be issue-, audience-, and product-agnostic?
Yes, that's basically right. As part of this, and as we've written about before, it's also important to make a distinction between trying to help an organization be more effective at what it's already trying to do, vs. trying to shift an organization's priorities to be more aligned with those of humanity/society as a whole. If you're not confident that there's sufficient alignment on the latter front, it may be premature or unwise to try to increase its technical capabilities through means like forecasting.
Do you expect that the EIP will be structured so as to, and have the resources to, do the needs analysis of relevant institutions, and then have individually specialised, expert staff on demand to deploy as the needs analysis suggests, or that you will have general competency staff at these different levels?
I mostly expect that EIP will not be deploying or running programs itself, at least in the near term. Instead, we have three resources we can leverage to make things happen: 1) funding, via relationships with funders in the EA community as well as outside of it; 2) talent, via relationships with 80,000 Hours and other entities providing direction and guidance to people looking for impactful ways to make a difference in their careers; and 3) our network of experts and practitioners who either study important institutions or are directly part of their orbit. Via the combination of these, we can both a) identify existing interventions/campaigns/ideas that can be boosted through dollars/people/knowledge/etc., and b) generate ideas for new interventions that we can help get off the ground by recruiting leaders and/or facilitating funding for them.
Denis @ 2023-05-24T08:29 (+3)
Just reading this now. I love the approach and especially the tangibility of it - identifying specific institutions, eventually developing specific plans to influence them in specific ways.
Of course there are people spending billions of dollars to influence some of these institutions, but that doesn't mean that a small, well-organised group cannot have an important impact with a well-designed, focused campaign.
My first reaction to the list itself is that it feels quite narrow (government institutions and IT companies). I wonder if this reflects reality or is just a result of the necessary limitations of a first iteration with quite limited resources. Nobody could question that every institution on this list is an important, influential institution, and if you manage to influence any of them in a positive way, that will be a great success.
My point is more that there seem to be whole classes of organisations and institutions with huge impact which are not represented at all in the list. And while one could perhaps argue that any one of the institutions listed is more important than any one of the institutions I suggest below, I'm not sure that necessarily means that the most effective way forward is to focus on just the types of institutions listed, vs. looking at what potential there is in a much broader group - especially focusing on the question of influenceability (on which I'd suggest several of the institutions listed above might score quite poorly).
Here are a few of the categories I would have expected to see in the list, but didn't:
- Oil companies, whose decisions are critical to the climate-crisis. Also OPEC, Gazprom and others.
- Pharma companies who have a critical role to play in global health - for example their decisions about enforcing patents in developing countries or allowing low-cost copies.
- FMCG Companies (Procter & Gamble, Unilever, ...) whose products are used by billions of people every single day, so anything they decide about health, or waste, or water-quality impacts so many people across the globe immediately. They also directly and indirectly (including supply-chain and distribution and sales) employ vast numbers of people all around the world, and their employment practices and policies directly impact many millions.
- Weapons manufacturers. Probably not realistic to hope they all just decide to stop making weapons, but they could do a lot by just not selling weapons so freely to authoritarian and inhumane regimes.
- Scientific bodies. So much of the world is driven by technology, which isn't just IT. It's true that bodies like governments and the EU do influence scientific research, but there may be many much-more-easily-influenced bodies which can also impact this. Maybe something as simple as requesting a speaking slot at an annual conference in which we'd highlight the gaps and opportunities could help create a new body of relevant research and development.
- Etc.
I'm not criticising the work - I think it's fantastic! - but rather wondering if it would be worth someone doing a second iteration and looking at some of these bodies which may not have been so obvious. All the ones I've listed above just reflect my personal expertise and experience as a chemical engineer, so probably is also far too narrow. Would be interesting to see what ideas we might get from a group of others, maybe historians, geologists, teachers, lawyers, biologists, astronomers, ...
Really looking forward to following this and seeing where it leads!
Nathan Young @ 2022-03-21T15:18 (+3)
Thanks to all who worked on this. It gave me a framework to think about this Which I realise I was missing.
This seems to me to describe clearly the "importance" aspect of different institutions, but most of "tractable" and "neglected" is in the model. I'd like to be better able to critique the choices there. For instance, what do you think the most tractable and neglected strategies are for improving these institutions? And why do you think they have been missed by others seeking to lobby these organisations?
.
IanDavidMoss @ 2022-03-21T23:06 (+2)
FWIW I tried to address this in my comment here.
Denkenberger @ 2022-12-03T19:33 (+2)
I've been wondering about cost-effectiveness in this space for a long time, so thanks for writing this and especially for releasing the quantitative model! At the top, it looks like you are saying that $100 million per year for 10 years could reduce x risk by about one percentage point, meaning about 100 basis points (0.01%) per billion dollars-is that correct? In the model column AF in tab X-risk, you say that the effort would be over a century, so does that mean spending $10 billion total? Elsewhere you say you consider spending $100 million per year just on one institution, so are you really talking about spending $100 billion this century on the top 10 institutions? Then this would be about 1 basis point per $billion. This is in the range of cost effectiveness values collected here.
So if I'm understanding you correctly, $10 billion spent over the century would reduce the existential risk from the US Government Executive Office of the President by 50%, and 40% of that would have happened anyway, so you are reducing overall existential risk by 0.135%, which would be 1.35 basis points per $billion?
IanDavidMoss @ 2022-12-03T21:08 (+2)
Hi David, thanks for your interest in our work! I need to preface this by emphasizing that the primary purpose of the quantitative model was to help us assess the relative importance of and promise of engaging with different institutions implicated in various existential risk scenarios. There was less attention given to the challenge of nailing the right absolute numbers, and so those should be taken with a super-extra-giant grain of salt.
With that said, the right way to understand the numbers in the model is that the estimates were about the impact over 100 years from a single one-time $100M commitment (perhaps distributed over multiple years) focusing on a single institution. The comment in the summary about $100 million/year was assuming that the funder(s) would focus on multiple institutions. Thus, the 100 basis points per billion figure is the "correct" one provided our per-institution estimates are in the right order of magnitude.
We're about to get started on our second iteration of this work and will have more capacity to devote to the cost-effectiveness estimates this time around, so hopefully that will result in less speculative outputs.
Denkenberger @ 2022-12-04T00:25 (+2)
Thanks for the clarification. I would say this is quite optimistic, but I look forward to your future cost-effectiveness work.