How much does performance differ between people?
By Max_Daniel, Benjamin_Todd @ 2021-03-25T22:56 (+119)
by Max Daniel & Benjamin Todd
[ETA: See also this summary of our findings + potential lessons by Ben for the 80k blog.]
Some people seem to achieve orders of magnitudes more than others in the same job. For instance, among companies funded by Y Combinator the top 0.5% account for more than ⅔ of the total market value; and among successful bestseller authors, the top 1% stay on the New York Times bestseller list more than 25 times longer than the median author in that group.
This is a striking and often unappreciated fact, but raises many questions. How many jobs have these huge differences in achievements? More importantly, why can achievements differ so much, and can we identify future top performers in advance? Are some people much more talented? Have they spent more time practicing key skills? Did they have more supportive environments, or start with more resources? Or did the top performers just get lucky?
More precisely, when recruiting, for instance, we’d want to know the following: when predicting the future performance of different people in a given job, what does the distribution of predicted (‘ex-ante’) performance look like?
This is an important question for EA community building and hiring. For instance, if it’s possible to identify people who will be able to have a particularly large positive impact on the world ahead of time, we’d likely want to take a more targeted approach to outreach.
More concretely, we may be interested in two different ways in which we could encounter large performance differences:
- If we look at a random person, by how much should we expect their performance to differ from the average?
- What share of total output should we expect to come from the small fraction of people we’re most optimistic about (say, the top 1% or top 0.1%) – that is, how heavy-tailed is the distribution of ex-ante performance?
(See this appendix for how these two notions differ from each other.)
Depending on the decision we’re facing we might be more interested in one or the other. Here we mostly focused on the second question, i.e., on how heavy the tails are.
This post contains our findings from a shallow literature review and theoretical arguments. Max was the lead author, building on some initial work by Ben, who also provided several rounds of comments.
You can see a short summary of our findings below.
We expect this post to be useful for:
- (Primarily:) Junior EA researchers who want to do further research in this area. See in particular the section on Further research.
- (Secondarily:) EA decision-makers who want to get a rough sense of what we do and don’t know about predicting performance. See in particular this summary and the bolded parts in our section on Findings.
- We weren’t maximally diligent with double-checking our spreadsheets etc.; if you wanted to rely heavily on a specific number we give, you might want to do additional vetting.
To determine the distribution of predicted performance, we proceed in two steps:
- We start with how ex-post performance is distributed. That is, how much did the performance of different people vary when we look back at completed tasks? On these questions, we’ll review empirical evidence on both typical jobs and expert performance (e.g. research).
- Then we ask how ex-ante performance is distributed. That is, when we employ our best methods to predict future performance by different people, how will these predictions vary? On these questions, we review empirical evidence on measurable factors correlating with performance as well as the implications of theoretical considerations on which kinds of processes will generate different types of distributions.
Here we adopt a very loose conception of performance that includes both short-term (e.g. sales made on one day) and long-term achievements (e.g. citations over a whole career). We also allow for performance metrics to be influenced by things beyond the performer’s control.
Our overall bottom lines are:
- Ex-post performance appears ‘heavy-tailed’ in many relevant domains, but with very large differences in how heavy-tailed: the top 1% account for between 4% to over 80% of the total. For instance, we find ‘heavy-tailed’ distributions (e.g. log-normal, power law) of scientific citations, startup valuations, income, and media sales. By contrast, a large meta-analysis reports ‘thin-tailed’ (Gaussian) distributions for ex-post performance in less complex jobs such as cook or mail carrier[1]: the top 1% account for 3-3.7% of the total. These figures illustrate that the difference between ‘thin-tailed’ and ‘heavy-tailed’ distributions can be modest in the range that matters in practice, while differences between ‘heavy-tailed’ distributions can be massive. (More.)
- Ex-ante performance is heavy-tailed in at least one relevant domain: science. More precisely, future citations as well as awards (e.g. Nobel Prize) are predicted by past citations in a range of disciplines, and in mathematics by scores at the International Maths Olympiad. (More.)
- More broadly, there are known, measurable correlates of performance in many domains (e.g. general mental ability). Several of them appear to remain valid in the tails. (More.)
- However, these correlations by itself don’t tell us much about the shape of the ex-ante performance distribution: in particular, they would be consistent with either thin-tailed or heavy-tailed ex-ante performance. (More.)
- Uncertainty should move us toward acting as if ex-ante performance was heavy-tailed – because if you have some credence in it being heavy-tailed, it’s heavy-tailed in expectation – but not all the way, and less so the smaller our credence in heavy-tails. (More.)
- To infer the shape of the ex-ante performance distribution, it would be more useful to have a mechanistic understanding of the process generating performance, but such fine-grained causal theories of performance are rarely available. (More.)
- Nevertheless, our best guess is that moderately to extremely heavy-tailed ex-ante performance is widespread at least for ‘complex’ and ‘scaleable’ tasks. (I.e. ones where the performance metric can in practice range over many orders of magnitude and isn’t artificially truncated.) This is based on our best guess at the causal processes that generate performance combined with the empirical data we’ve seen. However, we think this is debatable rather than conclusively established by the literature we reviewed. (More.)
- There are several opportunities for valuable further research. (More.)
Overall, doing this investigation probably made us a little less confident that highly heavy-tailed distributions of ex-ante performance are widespread, and we think that common arguments for it are often too quick. That said, we still think there are often large differences in performance (e.g. some software engineers have 10-times the output of others[2]), these are somewhat predictable, and it’s often reasonable to act on the assumption that the ex-ante distribution is heavy-tailed in many relevant domains (broadly, when dealing with something like ‘expert’ performance as opposed to ‘typical’ jobs).
Some advice for how to work with these concepts in practice:
- In practice, don’t treat ‘heavy-tailed’ as a binary property. Instead, ask how heavy the tails of some quantity of interest are, for instance by identifying the frequency of outliers you’re interested in (e.g. top 1%, top 0.1%, …) and comparing them to the median or looking at their share of the total.[3]
- Carefully choose the underlying population and the metric for performance, in a way that’s tailored to the purpose of your analysis. In particular, be mindful of whether you’re looking at the full distribution or some tail (e.g. wealth of all citizens vs. wealth of billionaires).
In an appendix, we provide more detail on some background considerations:
- The conceptual difference between ‘high variance’ and ‘heavy tails’: Neither property implies the other. Both mean that unusually good opportunities are much better than typical ones. However, only heavy tails imply that outliers account for a large share of the total, and that naive extrapolation underestimates the size of future outliers. (More.)
- We can often distinguish heavy-tailed from light-tailed data by eyeballing (e.g. in a log-log plot), but it’s hard to empirically distinguish different heavy-tailed distributions from one another (e.g. log-normal vs. power laws). When extrapolating beyond the range of observed data, we advise to proceed with caution and to not take the specific distributions reported in papers at face value. (More.)
- There is a small number of papers in industrial-organizational psychology on the specific question whether performance in typical jobs is normally distributed or heavy-tailed. However, we don’t give much weight to these papers because their broad high-level conclusion (“it depends”) is obvious but we have doubts about the statistical methods behind their more specific claims. (More.)
- We also quote (in more detail than in the main text) the results from a meta-analysis of predictors of salary, promotions, and career satisfaction. (More.)
- We provide a technical discussion of how our metrics for heavy-tailedness are affected by the ‘cutoff’ value at which the tail starts. (More.)
Finally, we provide a glossary of the key terms we use, such as performance or heavy-tailed.
For more details, see our full write-up.
Acknowledgments
We'd like to thank Owen Cotton-Barratt and Denise Melchin for helpful comments on earlier drafts of our write-up, as well as Aaron Gertler for advice on how to best post this piece on the Forum.
Most of Max's work on this project was done while he was part of the Research Scholars Programme (RSP) at FHI, and he's grateful to the RSP management and FHI operations teams for keeping FHI/RSP running, and to Hamish Hobbs and Nora Ammann for support with productivity and accountability.
We're also grateful to Balsa Delibasic for compiling and formatting the reference list.
[2] Similarly, don’t treat ‘heavy-tailed’ as an asymptotic property – i.e. one that by definition need only hold for values above some arbitrarily large value. Instead, consider the range of values that matter in practice. For instance, a distribution that exhibits heavy tails only for values greater than 10^100 would be heavy-tailed in the asymptotic sense. But for e.g. income in USD values like 10^100 would never show up in practice – if your distribution is supposed to correspond to income in USD you’d only be interested in a much smaller range, say up to 10^10. Note that this advice is in contrast to the standard definition of ‘heavy-tailed’ in mathematical contexts, where it usually is defined as an asymptotic property. Relatedly, a distribution that only takes values in some finite range – e.g. between 0 and 10 billion – is never heavy-tailed in the mathematical-asymptotic sense, but it may well be in the “practical” sense (where you anyway cannot empirically distinguish between a distribution that can take arbitrarily large values and one that is “cut off” beyond some very large maximum).
- ^
For performance in “high-complexity” jobs such as attorney or physician, that meta-analysis (Hunter et al. 1990) reports a coefficient of variation that’s about 1.5x as large as for ‘medium-complexity' jobs. Unfortunately, we can’t calculate how heavy-tailed the performance distribution for high-complexity jobs is: for this we would need to stipulate a particular type of distribution (e.g. normal, log-normal), but Hunter et al. only report that the distribution does not appear to be normal (unlike for the low- and medium-complexity cases).
- ^
Claims about a 10x output gap between the best and average programmers are very common, as evident from a Google search for ‘10x developer’. In terms of value rather than quantity of output, the WSJ has reported a Google executive claiming a 300x difference. For a discussion of such claims see, for instance, this blog post by Georgia Institute of Technology professor Mark Guzdial. Similarly, slide 37 of this version of Netflix's influential 'culture deck' claims (without source) that "In creative/inventive work, the best are 10x better than the average".
- ^
Similarly, don’t treat ‘heavy-tailed’ as an asymptotic property – i.e. one that by definition need only hold for values above some arbitrarily large value. Instead, consider the range of values that matter in practice. For instance, a distribution that exhibits heavy tails only for values greater than 10^100 would be heavy-tailed in the asymptotic sense. But for e.g. income in USD values like 10^100 would never show up in practice – if your distribution is supposed to correspond to income in USD you’d only be interested in a much smaller range, say up to 10^10. Note that this advice is in contrast to the standard definition of ‘heavy-tailed’ in mathematical contexts, where it usually is defined as an asymptotic property. Relatedly, a distribution that only takes values in some finite range – e.g. between 0 and 10 billion – is never heavy-tailed in the mathematical-asymptotic sense, but it may well be in the “practical” sense (where you anyway cannot empirically distinguish between a distribution that can take arbitrarily large values and one that is “cut off” beyond some very large maximum).
Khorton @ 2021-03-26T00:18 (+73)
"the top 1% stay on the New York Times bestseller list more than 25 times longer than the median author in that group."
FWIW my intuition is not that this author is 25x more talented, but rather that the author and their marketing team are a little bit more talented in a winner-takes-most market.
I wanted to point this out because I regularly see numbers like this used to justify claims that individuals vary significantly in talent or productivity. It's important to keep the business model in mind if you're claiming talent based on sales!
(Research citations are also a winner-takes-most market; people end up citing the same paper even if it's not much better than the next best paper.)
Max_Daniel @ 2021-03-26T08:32 (+17)
I fully agree with this, and think we essentially say as much in the post/document. This is e.g. why we've raised different explanations in the 2nd paragraph, immediately after referring to the phenomenon to be explained.
Curious if you think we could have done a better job at clarifying that we don't think differences in outcomes can only be explained by differences in talent?
esantorella @ 2021-03-27T14:01 (+35)
Let me try a different framing and see if that helps. Economic factors mediate how individual task performance translates into firm success. In industries with winner-takes-most effects, small differences in task performance cause huge differences in payoffs. "The Economics of Superstars" is a classic 1981 paper on this. But many industries aren't like that.
Knowing your industry tells you how important it is to hire the right people. If you're hiring someone to write an economics textbook (an example from the "Superstars" paper), you'd better hire the best textbook-writer you can find, because almost no one buys the tenth-best economics textbook. But if you're running a local landscaping company, you don't need the world's best landscaper. And if your industry has incumbent "superstar" firms protected by first-mover advantages, economies of scale, or network effects, it may not matter much who you hire.
So in what kind of "industry" are the EA organizations you want to help with hiring? Is there some factor that multiplies or negates small individual differences in task performance?
Benjamin_Todd @ 2021-03-30T19:46 (+5)
I think that's a good summary, but it's not only winner-takes-all effects that generate heavy-tailed outcomes.
You can get heavy tailed outcomes if performance is the product of two normally distributed factors (e.g. intelligence x effort).
It can also arise from the other factors that Max lists in another comment (e.g. scalable outputs, complex production).
Luck can also produce heavy tailed outcomes if it amplifies outcomes or is itself heavy-tailed.
esantorella @ 2021-04-02T11:41 (+10)
My point is more "context matters," even if you're talking about a specific skill like programming, and that the contexts that generated the examples in this post may be meaningfully different from the contexts that EA organizations are working in.
I don't necessarily disagree with anything you and Max have written; it's just a difference of emphasis, especially when it comes to advising people who are making hiring decisions.
MichaelPlant @ 2021-03-26T12:42 (+22)
I was going to raise a similar comment to what others have said here. I hope this adds something.
I think we need to distinguish quality and quantity of 'output' from 'success' (the outcome of their output). I am deliberately not using 'performance' as it's unclear, in common language, which one of the two it refers to. Various outputs are sometimes very reproducible - anyone can listen to a music track, or read an academic paper. There are often huge rewards to being the best vs second best - eg winning in sports. And sometimes success generates further success (the 'Matthew effect') - more people want to work with you, etc. Hence, I don't find it all weird to think that small differences in outputs, as measured on some cardinal scale, sometimes generate huge differences in outcomes.
I'm not sure exactly what follows from this. I'm a bit worried you're concentrated on the wrong metric - success - when it's outputs that are more important. Can you explain why you focus on outcomes?
Let's say you're thinking about funding research. How much does it matter to fund the best person? I mean, they will get most of the credit, but if you fund the less-than-best, that person's work is probably not much worse and ends up being used by the best person anyway. If the best person gets 1,000 more citations, should you be prepared to spend 1,000 more to fund their work? Not obviously.
I'm suspicious you can do a good job of predicting ex ante outcomes. After all, that's what VCs would want to do and they have enormous resources. Their strategy is basically to pick as many plausible winners as they can fund.
It might be interesting to investigate differences in quality and quantity of outputs separately. Intuitively, it seems the best people do produce lots more work than the good people, but it's less obvious the quality of the best people is much higher than of the good. I recognise all these terms are vague.
Benjamin_Todd @ 2021-03-28T19:40 (+22)
On your main point, this was the kind of thing we were trying to make clearer, so it's disappointing that hasn't come through.
Just on the particular VC example:
I'm suspicious you can do a good job of predicting ex ante outcomes. After all, that's what VCs would want to do and they have enormous resources. Their strategy is basically to pick as many plausible winners as they can fund.
Most VCs only pick from the top 1-5% of startups. E.g. YC's acceptance rate is 1%, and very few startups they reject make it to series A. More data on VC acceptance rates here: https://80000hours.org/2014/06/the-payoff-and-probability-of-obtaining-venture-capital/
So, I think that while it's mostly luck once you get down to the top 1-5%, I think there's a lot of predictors before that.
Also see more on predictors of startup performance here: https://80000hours.org/2012/02/entrepreneurship-a-game-of-poker-not-roulette/
John_Maxwell @ 2021-03-30T22:40 (+14)
YC having a low acceptance rate could mean they are highly confident in their ability to predict ex ante outcomes. It could also mean that they get a lot of unserious applications. Essays such as this one by Paul Graham bemoaning the difficulty of predicting ex ante outcomes make me think it is more the latter. ("it's mostly luck once you get down to the top 1-5%" makes it sound to me like ultra-successful startups should have elite founders, but my take on Graham's essay is that ultra-successful startups tend to be unusual, often in a way that makes them look non-elite according to traditional metrics -- I tend to suspect this is true of exceptionally innovative people more generally)
MichaelPlant @ 2021-03-29T12:45 (+2)
Hello Ben.
I'm not trying to be obtuse, it wasn't super clear to me on a quick-ish skim; maybe if I'd paid more attention I've have clocked it.
Yup, I was too hasty on VCs. It seems like they are pretty confident they know what the top >5% are, but not that can say anything more precise than. (Although I wonder what evidence indicates they can reliably tell the top 5% from those below, rather than they just think they can).
Ben_West @ 2021-03-31T21:30 (+38)
(Although I wonder what evidence indicates they can reliably tell the top 5% from those below, rather than they just think they can).
The Canadian inventors assistance program provides a rating of how good an invention is to inventors for a nominal fee. A large fraction of the people who get a bad rating try to make a company anyway, so we can judge the accuracy of their evaluations.
55% of the inventions which they give the highest rating to achieve commercial success, compared to 0% for the lowest rating.
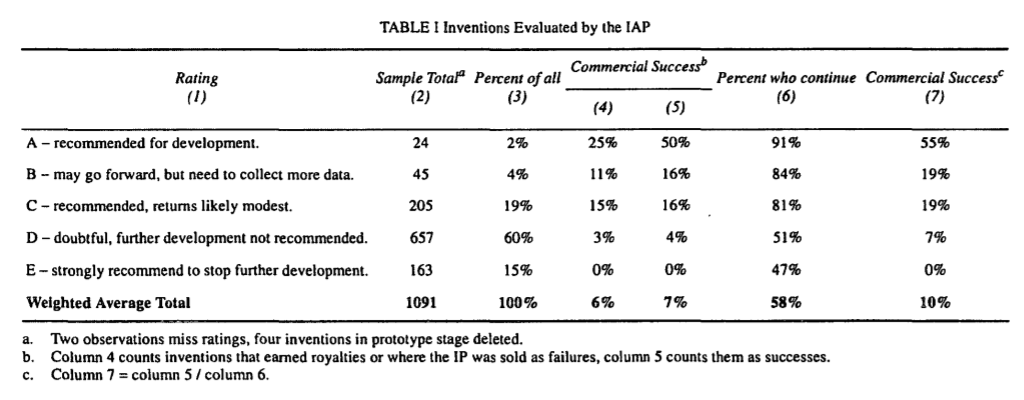
MichaelPlant @ 2021-04-01T08:23 (+9)
ah, this is great. evidence the selectors could tell the top 2% from the rest, but 2%-20% was much of a muchness. Shame that it doesn't give any more information on 'commercial success'.
Paulindrome @ 2021-04-01T19:54 (+5)
This is amazing data, and not what I would have expected - I've just had my mind changed on the predictability of invention success. Thanks!
Linch @ 2021-04-01T15:37 (+4)
This is really cool, thank you!
Max_Daniel @ 2021-04-01T07:45 (+4)
That's very interesting, thanks for sharing!
ETA: I've added this to our doc acknowledging your comment.
Max_Daniel @ 2021-03-29T15:09 (+18)
I'm not trying to be obtuse, it wasn't super clear to me on a quick-ish skim; maybe if I'd paid more attention I've have clocked it.
FWIW I think it's the authors' job to anticipate how their audience is going to engage with their writing, where they're coming from etc. - You were not the only one who reacted by pushing back against our framing as evident e.g. from Khorton's much upvoted comment.
So no matter what we tried to convey, and what info is in the post or document if one reads closely enough, I think this primarily means that I (as main author of the wording in the post) could have done a better job, not that you or anyone else is being obtuse.
Max_Daniel @ 2021-03-26T13:41 (+6)
I'm suspicious you can do a good job of predicting ex ante outcomes. After all, that's what VCs would want to do and they have enormous resources. Their strategy is basically to pick as many plausible winners as they can fund.
I agree that looking at e.g. VC practices is relevant evidence. However, it seems to me that if VCs thought they couldn't predict anything, they would allocate their capital by a uniform lottery among all applicants, or something like that. I'm not aware of a VC adopting such a strategy (though possible I just haven't heard of it); to the extent that they can distinguish "plausible" from "implausible" winners, this does suggest some amount of ex-ante predictability. Similarly, my vague impression is that VCs and other investors often specialize by domain/sector, which suggests they think they can utilize their knowledge and network when making decisions ex ante.
Sure, predictability may be "low" in some sense, but I'm not sure we're saying anything that would commit us to denying this.
MichaelPlant @ 2021-03-26T14:49 (+2)
Yeah, I'd be interested to know if VC were better than chance. Not quite sure how you would assess this, but probably someone's tried.
But here's where it seems relevant. If you want to pick the top 1% of people, as they provide so much of the value, but you can only pick the top 10%, then your efforts to pick are much less cost-effective and you would likely want to rethink how you did it.
Max_Daniel @ 2021-03-26T17:17 (+9)
I think it's plausible that VCs aren't better than chance when choosing between a suitably restricted "population", i.e. investment opportunities that have passed some bar of "plausibility".
I don't think it's plausible that they are no better than chance simpliciter. In that case I would expect to see a lot of VCs who cut costs by investing literally zero time into assessing investment opportunities and literally fund on a first-come first-serve or lottery basis.
Max_Daniel @ 2021-03-26T17:21 (+2)
And yes, I totally agree that how well we can predict (rather than just the question whether predictability is zero or nonzero) is relevant in practice.
If the ex-post distribution is heavy-tailed, there are a bunch of subtle considerations here I'd love someone to tease out. For example, if you have a prediction method that is very good for the bottom 90% but biased toward 'typical' outcomes, i.e. the median, then you might be better off in expectation to allocate by a lottery over the full population (b/c this gets you the mean, which for heavy-tailed distributions will be much higher than the median).
Ben_West @ 2021-03-31T21:35 (+11)
Data from the IAP indicates that they can identify the top few percent of successful inventions with pretty good accuracy. (Where "success" is a binary variable – not sure how they perform if you measure financial returns.)
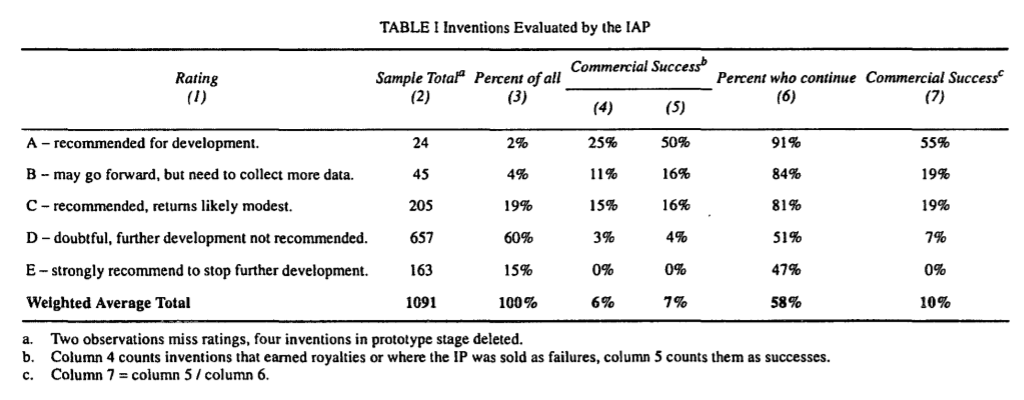
Max_Daniel @ 2021-03-26T13:34 (+5)
I'm not sure exactly what follows from this. I'm a bit worried you're concentrated on the wrong metric - success - when it's outputs that are more important. Can you explain why you focus on outcomes?
I'm not sure I agree that outputs are more important. I think it depends a lot on the question or decision we're considering, which is why I highlighted a careful choice of metric as one of the key pieces of advice.
So e.g. if our goal is to set performance incentives (e.g. salaries), then it may be best to reward people for things that are under their control. E.g. pay people more if they work longer hours (inputs), or if there are fewer spelling mistakes in their report (cardinal output metric) or whatever. At other times, paying more attention to inputs or outputs rather than outcomes or things beyond the individual performer's control may be justified by considerations around e.g. fairness or equality.
All of these things are of course really important to get right within the EA community as well, whether or not we care about them instrumentally or intrinsically. There are lot of tricky and messy questions here.
But if we can say anything general, then I think that especially in EA contexts we care more, ore more often, about outcomes/success/impact on the world, and less about inputs and outputs, than usual. We want to maximize well-being, and from 'the point of view of the universe' it doesn't ultimately matter if someone is happy because someone else produced more outputs or because the same outputs had greater effects. Nor does it ultimately matter if impact differences are due to differences in talent, resource endowments, motivation, luck, or ...
Another way to see this is that often actors that care more about inputs or outputs do so because they don't internalize all the benefits from outcomes. But if a decision is motivated by impartial altruism, there is a sense in which there are no externalities.
Of course, we need to make all the usual caveats against 'naive consequentialism'. But I do think there is something important in this observation.
MichaelPlant @ 2021-03-26T15:00 (+5)
I was thinking the emphasis on outputs might be the important part as those are more controllable than outcomes, and so the decision-relevant bit, even though we want to maximise impartial value (outcomes).
I can imagine someone thinking the following way: "we must find and fund the best scientists because they have such outsized outcomes, in terms of citations." But that might be naive if it's really just the top scientist who gets the citations and the work of all the good scientists has a more or less equal contribution to impartial value.
FWIW, it's not clear we're disagreeing!
Max_Daniel @ 2021-03-26T13:13 (+3)
Thanks for this comment!
I'm sympathetic to the point that we're lumping together quite different things under the vague label "performance", perhaps stretching its beyond its common use. That's why I said in bold that we're using a loose notion of performance. But it's possible it would have been better if I had spent more time to come up with a better terminology.
MichaelPlant @ 2021-03-26T14:50 (+7)
Okay good! Yeah, I would be curious to see how much it changed the analysis distinguishing outputs from outcomes and, further, between different types of outputs.
Khorton @ 2021-03-26T09:19 (+6)
I think the language of a person who "achieves orders of magnitude more" suggests that their output (research, book, etc) is orders of magnitude better, instead of just being more popular. Sometimes more popular is better, but often in EA that's not what we're focused on.
I also believe you're talking about hiring individuals in this piece(?), but most of your examples are about successful teams, which have different qualities to successful individuals.
I thought your examples of Math Olympiad scores correlating with Nobel Prize wins was a useful exception to this trend, because those are about an individual and aren't just about popularity.
Max_Daniel @ 2021-03-26T09:41 (+7)
Thanks for clarifying!
FWIW I think I see the distinction between popularity and other qualities as less clear as you seem to do. For instance, I would expect that book sales and startup returns are also affected by how "good" in whatever other sense the book or startup product is. Conversely, I would guess that realistically Nobel Prizes and other scientific awards are also about popularity and not just about the quality of the scientific work by other standards. I'm happy to concede that, in some sense, book sales seem more affected by popularity than Nobel Prizes, but it seems a somewhat important insight to me that neither is "just about popularity" nor "just about achievement/talent/quality/whatever".
It's also not that clear to me whether there is an obviously more adequate standard of overall "goodness" here: how much joy the book brings readers? What literary critics would say about the book? I think the ultimate lesson here is that the choice of metric is really important, and depends a lot on what you want to know or decide, which is why "Carefully choose the underlying population and the metric for performance" is one of our key points of advice. I can see that saying something vague and general like "some people achieve more" and then giving examples of specific metrics pushes against this insight by suggesting that these are the metrics we should generally most care about. FWIW I still feel OK about our wording here since I feel like in an opening paragraph we need to balance nuance/detail and conciseness / getting the reader interested.
As an aside, my vague impression is that it's somewhat controversial to what extent successful teams have different qualities to successful individuals. In some sense this is of course true since there are team properties that don't even make sense for individuals. However, my memory is that for a while there was some more specific work in psychology that was allegedly identifying properties that predicted team success better than the individual abilities of its members, which then largely didn't replicate.
Stefan_Schubert @ 2021-03-26T11:09 (+6)
However, my memory is that for a while there was some more specific work in psychology that was allegedly identifying properties that predicted team success better than the individual abilities of its members, which then largely didn't replicate.
Woolley et al (2010) was an influential paper arguing that individual intelligence doesn't predict collective intelligence well. Here's one paper criticising them. I'm sure there are plenty of other relevant papers (I seem to recall one paper providing positive evidence that individual intelligence predicted group performance fairly well, but can't find it now).
Max_Daniel @ 2021-03-26T11:25 (+4)
Great, thank you! I do believe work by Woolley was what I had in mind.
Stefan_Schubert @ 2021-03-26T00:37 (+16)
Fwiw, I wrote a post explaining such dynamics a few years ago.
reallyeli @ 2021-03-26T07:03 (+4)
Agreed. The slight initial edge that drives the eventual enormous success in the winner-takes-most market can also be provided by something other than talent — that is, by something other than people trying to do things and succeeding at what they tried to do. For example, the success of Fifty Shades of Grey seems best explained by luck.
Erich_Grunewald @ 2021-03-26T07:44 (+4)
I was going to comment something to this effect, too. The authors write:
For instance, we find ‘heavy-tailed’ distributions (e.g. log-normal, power law) of scientific citations, startup valuations, income, and media sales. By contrast, a large meta-analysis reports ‘thin-tailed’ (Gaussian) distributions for ex-post performance in less complex jobs such as cook or mail carrier: the top 1% account for 3-3.7% of the total.
But there’s an important difference between these groups – the products involved in the first group are cheaply reproducible (any number of people can read the same papers, invest in the same start-up or read the same articles – I don’t know how to interpret income here) & those in the second group are not (not everyone can use the same cook or mail carrier).
So I propose that the difference there has less to do with the complexity of the jobs & more to do with how reproducible the products involved are.
Max_Daniel @ 2021-03-26T08:39 (+13)
I think you're right that complexity at the very least isn't the only cause/explanation for these differences.
E.g. Aguinis et al. (2016) find that, based on an analysis of a very large number of productivity data sets, the following properties make a heavy-tailed output distribution more likely:
- Multiplicity of productivity,
- Monopolistic productivity,
- Job autonomy,
- Job complexity,
- No productivity ceiling (I guess your point is a special case of this: if the marginal cost of increasing output becomes too high too soon, there will effectively be a ceiling; but there can also e.g. be ceilings imposed by the output metric we use, such as when a manager gives a productivity rating on a 1-10 scale)
As we explain in the paper, I have some open questions about the statistical approach in that paper. So I currently don't take their analysis to be that much evidence that this is in fact right. However, they also sound right to me just based on priors and based on theoretical considerations (such as the ones in our section on why we expect heavy-tailed ex-ante performance to be widespread).
In the part you quoted, I wrote "less complex jobs" because the data I'm reporting is from a paper that explicitly distinguishes low-, medium-, and high-complexity jobs, and finds that only the first two types of job potentially have a Gaussian output distribution (this is Hunter et al. 1990). [TBC, I understand that the reader won't know this, and I do think my current wording is a bit sloppy/bad/will predictably lead to the valid pushback you made.]
[References in the doc linked in the OP.]
Erich_Grunewald @ 2021-03-26T21:27 (+3)
Thanks for the clarification & references!
Benjamin_Todd @ 2021-03-30T15:30 (+2)
This is cool.
One theoretical point in favour of complexity is that complex production often looks like an 'o-ring' process, which will create heavy-tailed outcomes.
AGB @ 2021-04-02T18:31 (+37)
Hi Max and Ben, a few related thoughts below. Many of these are mentioned in various places in the doc, so seem to have been understood, but nonetheless have implications for your summary and qualitative commentary, which I sometimes think misses the mark.
- Many distributions are heavy-tailed mathematically, but not in the common use of that term, which I think is closer to 'how concentrated is the thing into the top 0.1%/1%/etc.', and thus 'how important is it I find top performers' or 'how important is it to attract the top performers'. For example, you write the following:
What share of total output should we expect to come from the small fraction of people we’re most optimistic about (say, the top 1% or top 0.1%) – that is, how heavy-tailed is the distribution of ex-ante performance?
- Often, you can't derive this directly from the distribution's mathematical type. In particular, you cannot derive it from whether a distribution is heavy-tailed in the mathematical sense.
- Log-normal distributions are particuarly common and are a particular offender here, because they tend to occur whenever lots of independent factors are multiplied together. But here is the approximate* fraction of value that comes from the top 1% in a few different log-normal distributions:
EXP(N(0,0.0001)) -> 1.02%
EXP(N(0,0001)) -> 1.08%
EXP(N(0,0.01)) -> 1.28%
EXP(N(0,0.1)) -> 2.22%
EXP(N(0,1)) -> 9.5% - For a real-world example, geometric brownian motion is the most common model of stock prices, and produces a log-normal distribution of prices, but models based on GBM actually produce pretty thin tails in the commonsense use, which are in turn much thinner than the tails in real stock markets, as (in?)famously chronicled in Taleb's Black Swan among others. Since I'm a finance person who came of age right as that book was written, I'm particularly used to thinking of the log-normal distribution as 'the stupidly-thin-tailed one', and have a brief moment of confusion every time it is referred to as 'heavy-tailed'.
- The above, in my opinion, highlights the folly of ever thinking 'well, log-normal distributions are heavy-tailed, and this should be log-normal because things got multiplied together, so the top 1% must be at least a few percent of the overall value'. Log-normal distributions with low variance are practically indistinguishable from normal distributions. In fact, as I understand it many oft-used examples of normal distributions, such as height and other biological properties, are actually believed to follow a log-normal distribution.
***
I'd guess we agree on the above, though if not I'd welcome a correction. But I'll go ahead and flag bits of your summary that look weird to me assuming we agree on the mathematical facts:
By contrast, a large meta-analysis reports ‘thin-tailed’ (Gaussian) distributions for ex-post performance in less complex jobs such as cook or mail carrier [1]: the top 1% account for 3-3.7% of the total.
I haven't read the meta-analysis, but I'd tentatively bet that much like biological properties these jobs actually follow log-normal distributions and they just couldn't tell (and weren't trying to tell) the difference.
These figures illustrate that the difference between ‘thin-tailed’ and ‘heavy-tailed’ distributions can be modest in the range that matters in practice
I agree with the direction of this statement, but it's actually worse than that: depending on the tail of interest "heavy-tailed distributions" can have thinner tails than "thin-tailed distributions"! For example, compare my numbers for the top 1% of various log-normal distributions to the right-hand-side of a standard N(0,1) normal distribution where we cut off negative values (~3.5% in top 1%).
It's also somewhat common to see comments like this from 80k staff (This from Ben Todd elsewhere in this thread):
You can get heavy tailed outcomes if performance is the product of two normally distributed factors (e.g. intelligence x effort).
You indeed can, but like the log-normal distribution this will tend to have pretty thin tails in the common use of the term. For example, multipling two N(100,225) distributions together, chosen because this is roughly the distribution of IQ, gets you a distribution where the top 1% account for 1.6% of the total. Looping back to my above thought, I'd also guess that performance on jobs like cook and mail-carrier look very close to this, and empirically were observed to have similarly thin tails (aptitude x intelligence x effort might in fact be the right framing for these jobs).
***
Ultimately, the recommendation I would give is much the same as the bottom line presented, which I was very happy to see. Indeed, I'm mostly grumbling because I want to discourage anything which treats heavy-tailed as a binary property**, as parts of the summary/commentary tend to, see above.
Some advice for how to work with these concepts in practice:
- In practice, don’t treat ‘heavy-tailed’ as a binary property. Instead, ask how heavy the tails of some quantity of interest are, for instance by identifying the frequency of outliers you’re interested in (e.g. top 1%, top 0.1%, …) and comparing them to the median or looking at their share of the total. [2]
- Carefully choose the underlying population and the metric for performance, in a way that’s tailored to the purpose of your analysis. In particular, be mindful of whether you’re looking at the full distribution or some tail (e.g. wealth of all citizens vs. wealth of billionaires).
*Approximate because I was lazy and just simulated 10000 values to get these and other quoted numbers. AFAIK the true values are not sufficiently different to affect the point I'm making.
**If it were up to me, I'd taboo the term 'heavy-tailed' entirely, because having an oft-used term whose mathematical and commonsense notions differ is an obvious recipe for miscommunication in a STEM-heavy community like this one.
Max_Daniel @ 2021-04-03T10:22 (+4)
Yeah, I think we agree on the maths, and I'm quite sympathetic to your recommendations regarding framing based on this. In fact, emphasizing "top x% share" as metric and avoiding any suggestion that it's practically useful to treat "heavy-tailed" as a binary property were my key goals for the last round of revisions I made to the summary - but it seems like I didn't fully succeed.
FWIW, I maybe wouldn't go quite as far as you suggest in some places. I think the issue of "mathematically 'heavy-tailed' distributions may not be heavy-tailed in practice in the everyday sense" is an instance of a broader issue that crops up whenever one uses mathematical concepts that are defined in asymptotic terms in applied contexts.
To give just one example, consider that we often talk of "linear growth", "exponential growth", etc. I think this is quite useful, and that it would overall be bad to 'taboo' these terms and always replace them with some 'model-agnostic' metric that can be calculated for finitely many data points. But there we have the analog issue that depending on the parameters an e.g. exponential function can for practical purposes look very much like a linear function over the relevant finite range of data.
Another example would be computational complexity, e.g. when we talk about algorithms being "polynomial" or "exponential" regarding how many steps they require as function of the size of their inputs.
Yet another example would be attractors in dynamical systems.
In these and many other cases we encounter the same phenomenon that we often talk in terms of mathematical concepts that by definition only tell us that some property holds "eventually", i.e. in the limit of arbitrarily long amounts of time, arbitrarily much data, or similar.
Of course, being aware of this really is important. In practice it often is crucial to have an intuition or more precise quantitative bounds on e.g. whether we have enough data points to be able to use some computational method that's only guaranteed to work in the limit of infinite data. And sometimes we are better off using some algorithm that for sufficiently large inputs would be slower than some alternative, etc.
But on the other hand, talk in terms of 'asymptotic' concepts often is useful as well. I think one reason for why is that in practice when e.g. we say that something "looks like a heavy-tailed distribution" or that something "looks like exponential growth" we tend to mean "the top 1% share is relatively large / it would be hard to fit e.g. a normal distribution" or "it would be hard to fit a straight line to this data" etc., as opposed to just e.g. "there is a mathematically heavy-tailed distribution that with the right parameters provides a reasonable fit" or "there is an exponential function that with the right parameters provides a reasonable fit". That is, the conventions for the use of these terms are significantly influenced by "practical" considerations (and things like Grice's communication maxims) rather than just their mathematical definition.
So e.g. concretely when in practice we say that something is "log-normally distributed" we often do mean that it looks more heavy-tailed in the everyday sense than a normal distribution (even though it is a mathematical fact that there are log-normal distributions that are relatively thin-tailed in the everyday sense - indeed we can make most types of distributions arbitrarily thin-tailed or heavy-tailed in this sense!).
AGB @ 2021-04-03T11:27 (+21)
So taking a step back for a second, I think the primary point of collaborative written or spoken communication is to take the picture or conceptual map in my head and put it in your head, as accurately as possible. Use of any terms should, in my view, be assessed against whether those terms are likely to create the right picture in a reader's or listener's head. I appreciate this is a somewhat extreme position.
If everytime you use the term heavy-tailed (and it's used a lot - a quick CTRL + F tells me it's in the OP 25 times) I have to guess from context whether you mean the mathematical or commonsense definitions, it's more difficult to parse what you actually mean in any given sentence. If someone is reading and doesn't even know that those definitions substantially differ, they'll probably come away with bad conclusions.
This isn't a hypothetical corner case - I keep seeing people come to bad (or at least unsupported) conclusions in exactly this way, while thinking that their reasoning is mathematically sound and thus nigh-incontrovertible. To quote myself above:
The above, in my opinion, highlights the folly of ever thinking 'well, log-normal distributions are heavy-tailed, and this should be log-normal because things got multiplied together, so the top 1% must be at least a few percent of the overall value'.
If I noticed that use of terms like 'linear growth' or 'exponential growth' were similarly leading to bad conclusions, e.g. by being extrapolated too far beyond the range of data in the sample, I would be similarly opposed to their use. But I don't, so I'm not.
If I noticed that engineers at firms I have worked for were obsessed with replacing exponential algorithms with polynomial algorithms because they are better in some limit case, but worse in the actual use cases, I would point this out and suggest they stop thinking in those terms. But this hasn't happened, so I haven't ever done so.
I do notice that use of the term heavy-tailed (as a binary) in EA, especially with reference to the log-normal distribution, is causing people to make claims about how we should expect this to be 'a heavy-tailed distribution' and how important it therefore is to attract the top 1%, and so...you get the idea.
Still, a full taboo is unrealistic and was intended as an aside; closer to 'in my ideal world' or 'this is what I aim for my own writing', rather than a practical suggestion to others. As I said, I think the actual suggestions made in this summary are good - replacing the question 'is this heavy-tailed or not' with 'how heavy-tailed is this' should do the trick- and hope to see them become more widely adopted.
Max_Daniel @ 2021-04-03T16:31 (+4)
I'm not sure how extreme your general take on communication is, and I think at least I have a fairly similar view.
I agree that the kind of practical experiences you mention can be a good reason to be more careful with the use of some mathematical concepts but not others. I think I've seen fewer instances of people making fallacious inferences based on something being log-normal, but if I had I think I might have arrived at similar aspirations as you regarding how to frame things.
(An invalid type of argument I have seen frequently is actually the "things multiply, so we get a log-normal" part. But as you have pointed out in your top-level comment, if we multiply a small number of thin-tailed and low-variance factors we'll get something that's not exactly a 'paradigmatic example' of a log-normal distribution even though we could reasonably approximate it with one. On the other hand, if the conditions of the 'multiplicative CLT' aren't fulfilled we can easily get something with heavier tails than a log-normal. See also fn26 in our doc:
We’ve sometimes encountered the misconception that products of light-tailed factors always converge to a log-normal distribution. However, in fact, depending on the details the limit can also be another type of heavy-tailed distribution, such as a power law (see, e.g., Mitzenmacher 2004, sc. 5-7 for an accessible discussion and examples). Relevant details include whether there is a strictly positive minimum value beyond which products can’t fall (ibid., sc. 5.1), random variation in the number of factors (ibid., sc. 7), and correlations between factors.
)
Max_Daniel @ 2021-04-03T10:51 (+2)
As an aside, for a good and philosophically rigorous criticism of cavalier assumptions of normality or (arguably) pseudo-explanations that involve the central limit theorem, I'd recommend Lyon (2014), Why are Normal Distributions Normal?
Basically I think that whenever we are in the business of understanding how things actually work/"why" we're seeing the data distributions we're seeing, often-invoked explanations like the CLT or "multiplicative" CLT are kind of the tip of the iceberg that provides the "actual" explanation (rather then being literally correct by themselves), this iceberg having to do with the principle of maximum entropy / the tendency for entropy to increase / 'universality' and the fact that certain types of distributions are 'attractors' for a wide range of generating processes. I'm too much of an 'abstract algebra person' to have a clear sense of what's going on, but I think it's fairly clear that the folk story of "a lot of things 'are' normally distributed because of 'the' central limit theorem" is at best an 'approximation' and at worst misleading.
(One 'mathematical' way to see this is that it's fishy that there are so many different versions of the CLT rather than one clear 'canonical' or 'maximally general' one. I guess stuff like this also is why I tend to find common introductions to statistics horribly unaesthetic and have had a hard time engaging with them.)
Max_Daniel @ 2021-04-03T10:38 (+2)
I haven't read the meta-analysis, but I'd tentatively bet that much like biological properties these jobs actually follow log-normal distributions and they just couldn't tell (and weren't trying to tell) the difference.
I kind of agree with this (and this is why I deliberately said that "they report a Gaussian distribution" rather than e.g. "performance is normally distributed"). In particular, yes, they just assumed a normal distribution and then ran with this in all cases in which it didn't lead to obvious problems/bad fits no matter the parameters. They did not compare Gaussian with other models.
I still think it's accurate and useful to say that they were using (and didn't reject) a normal distribution as model for low- and medium-complexity jobs as this does tell you something about how the data looks like. (Since there is a lot of possible data where no normal distribution is a reasonable fit.)
I also agree that probably a log-normal model is "closer to the truth" than a normal one. But on the other hand I think it's pretty clear that actually neither a normal nor a log-normal model is fully correct. Indeed, what would it mean that "jobs actually follow a certain type of distribution"? If we're just talking about fitting a distribution to data, we will never get a perfect fit, and all we can do is providing goodness-of-fit statistics for different models (which usually won't conclusively identify any single one). This kind of brute/naive empiricism just won't and can't get us to "how things actually work". On the other hand, if we try to build a model of the causal generating mechanism of job performance it seems clear that the 'truth' will be much more complex and messy - we will only have finitely many contributing things (and a log-normal distribution is something we'd get at best "in the limit"), the contributing factors won't all be independent etc. etc. Indeed, "probability distribution" to me basically seems like the wrong type to talk about when we're in the business of understanding "how things actually work" - what we want then is really a richer and more complex model (in the sense that we could have several different models that would yield the same approximate data distribution but that would paint a fairly different picture of "how things actually work"; basically I'm saying that things like 'quantum mechanics' or 'the Solow growth model' or whatever have much more structure and are not a single probability distribution).
AGB @ 2021-04-03T10:55 (+4)
Briefly on this, I think my issue becomes clearer if you look at the full section.
If we agree that log-normal is more likely than normal, and log-normal distributions are heavy-tailed, then saying 'By contrast, [performance in these jobs] is thin-tailed' is just incorrect? Assuming you meant the mathematical senses of heavy-tailed and thin-tailed here, which I guess I'm not sure if you did.
This uncertainty and resulting inability to assess whether this section is true or false obviously loops back to why I would prefer not to use the term 'heavy-tailed' at all, which I will address in more detail in my reply to your other comment.
Ex-post performance appears ‘heavy-tailed’ in many relevant domains, but with very large differences in how heavy-tailed: the top 1% account for between 4% to over 80% of the total. For instance, we find ‘heavy-tailed’ distributions (e.g. log-normal, power law) of scientific citations, startup valuations, income, and media sales. By contrast, a large meta-analysis reports ‘thin-tailed’ (Gaussian) distributions for ex-post performance in less complex jobs such as cook or mail carrier
Max_Daniel @ 2021-04-03T16:20 (+2)
I think the main takeaway here is that you find that section confusing, and that's not something one can "argue away", and does point to room for improvement in my writing. :)
With that being said, note that we in fact don't say anywhere that anything 'is thin-tailed'. We just say that some paper 'reports' a thin-tailed distribution, which seems uncontroversially true. (OTOH I can totally see that the "by contrast" is confusing on some readings. And I also agree that it basically doesn't matter what we say literally - if people read what we say as claiming that something is thin-tailed, then that's a problem.)
FWIW, from my perspective the key observations (which I apparently failed to convey in a clear way at least for you) here are:
- The top 1% share of ex-post "performance" [though see elsewhere that maybe that's not the ideal term] data reported in the literature varies a lot, at least between 3% and 80%. So usually you'll want to know roughly where on the spectrum you are for the job/task/situation relevant to you rather than just whether or not some binary property holds.
- The range of top 1% shares is almost as large for data for which the sources used a mathematically 'heavy-tailed' type of distribution as model. In particular, there are some cases where we some source reports a mathematically 'heavy-tailed' distribution but where the top 1% share is barely larger than for other data based on a mathematically 'thin-tailed' distribution.
- (As discussed elsewhere, it's of course mathematically possible to have a mathematically 'thin-tailed' distribution with a larger top 1% share than a mathematically 'heavy-tailed' distribution. But the above observation is about what we in fact find in the literature rather than about what's mathematically possible. I think the key point here is not so much that we haven't found a 'thin-tailed' distribution with larger top 1% share than some 'heavy-tailed' distribution. but that the mathematical 'heavy-tailed' property doesn't cleanly distinguish data/distributions by their top 1% share even in practice.)
- So don't look at whether the type of distribution used is 'thin-tailed' or 'heavy-tailed' in the mathematical sense, ask how heavy-tailed in the everyday sense (as operationalized by top 1% share or whatever you care about) your data/distribution is.
So basically what I tried to do is mentioning that we find both mathematically thin-tailed and mathematically heavy-tailed distributions reported in the literature in order to point out that this arguably isn't the key thing to pay attention to. (But yeah I can totally see that this is not coming across in the summary as currently worded.)
As I tried to explain in my previous comment, I think the question whether performance in some domain is actually 'thin-tailed' or 'heavy-tailed' in the mathematical sense is closer to ill-posed or meaningless than true or false. Hence why I set aside the issue of whether a normal distribution or similar-looking log-normal distribution is the better model.
Linch @ 2021-03-26T00:52 (+34)
Thanks for this. I do think there's a bit of sloppiness in EA discussions about heavy-tailed distributions in general, and the specific question of differences in ex ante predictable job performance in particular. So it's really good to see clearer work/thinking about this.
I have two high-level operationalization concerns here:
- Whether performance is ex ante predictable seems to be a larger function of our predictive ability than of the world. As an extreme example of what I mean, if you take our world on November 7, 2016 and run high-fidelity simulations 1,000,000 times , I expect 1 million/1 million of those simulations to end up with Donald Trump winning the 2016 US presidential election. Similarly, with perfect predictive ability, I think the correlation between ex ante predicted work performance and ex post actual performance approach 1 (up to quantum) . This may seem like a minor technical point, but I think it's important to be careful of the reasoning here when we ask whether claims are expected to generalize from domains with large and obvious track records and proxies (eg past paper citations to future paper citations) or even domains where the ex ante proxy may well have been defined ex post (Math Olympiad records to research mathematics) to domains of effective altruism where we're interested in something like counterfactual/Shapley impact*.
- There's counterfactual credit assignment issues for pretty much everything EA is concerned with, whereas if you're just interested in individual salaries or job performance in academia, a simple proxy like $s or citations is fine. Suppose Usain Bolt is 0.2 seconds slower at running 100 meters. Does anybody actually think this will result in huge differences in the popularity of sports, or percentage of economic output attributable to the "run really fast" fraction of the economy, never mind our probability of spreading utopia throughout the stars? But nonetheless Usain Bolt likely makes a lot more money, has a lot more prestige, etc than the 2nd/3rd fastest runners. Similarly, academics seem to worry constantly about getting "scooped" whereas they rarely worry about scooping others, so a small edge in intelligence or connections or whatever can be leveraged to a huge difference in potential citations, while being basically irrelevant to counterfactual impact. Whereas in EA research it matters a lot whether being "first" means you're 5 years ahead of the next-best candidate or 5 days.
Griping aside, I think this is a great piece and I look forward to perusing it and giving more careful comments in the coming weeks!
*ETA: In contrast, if it's the same variable(s) that we can use to ex ante predict a variety of good outcomes of work performance across domains, then we can be relatively more confident that this will generalize to EA notions. Eg, fundamental general mental ability, integrity, etc.
Max_Daniel @ 2021-03-26T08:53 (+13)
Thanks for these points!
My super quick take is that 1. definitely sounds right and important to me, and I think it would have been good if we had discussed this more in the doc.
I think 2. points to the super important question (which I think we've mentioned somewhere under Further research) how typical performance/output metrics relate to what we ultimately care about in EA contexts, i.e. positive impact on well-being. At first glance I'd guess that sometimes these metrics 'overstate' heavy-tailedness of EA impact (for e.g. the reasons you mentioned), but sometimes they might also 'understate' them. For instance, the metrics might not 'internalize' all the effects on the world (e.g. 'field building' effects from early-stage efforts), or for some EA situations the 'market' may be even more winner-takes-most than usual (e.g. for some AI alignment efforts it only matters if you can influence DeepMind), or the 'production function' might have higher returns to talent than usual (e.g. perhaps founding a nonprofit or contributing valuable research to preparadigmatic fields is "extra hard" in a way not captured by standard metrics when compared to easier cases).
Benjamin_Todd @ 2021-03-30T19:48 (+18)
I tried to sum up the key messages in plain language in a Twitter thread, in case that helps clarify.
Benjamin_Todd @ 2021-05-09T13:39 (+9)
And now I've created a more accurate summary here: https://80000hours.org/2021/05/how-much-do-people-differ-in-productivity/
Charles He @ 2021-03-27T18:40 (+16)
Heyo Heyo!
C-dawg in the house!
I have concerns about how this post and research is framed and motivated.
This is because its methods imply a certain worldview and is trying to help hiring or recruiting decisions in EA orgs, and we should be cautious.
Star systems
Like, I think, loosely speaking, I think “star systems” is a useful concept / counterexample to this post.
In this view of the world, someone’s in a “star system” if a small number of people get all the rewards, but not from what we would comfortably call productivity or performance.
So, like, for intuition, most Olympic athletes train near poverty but a small number manage to “get on a cereal box” and become a millionaire. They have higher ability, but we wouldn’t say that Gold medal winners are 1000x more productive than someone they beat by 0.05 seconds.
You might view “Star systems” negatively because they are unfair—Yes, and in addition to inequality, they have may have very negative effects: they promote echo chambers in R1 research, and also support abuse like that committed by Harvey Weinstein.
However, “star systems” might be natural and optimal given how organizations and projects need to be executed. For intuition, there can be only one architect of a building or one CEO of an org.
It’s probably not difficult to build a model where people of very similar ability work together and end up with a CEO model with very unequal incomes. It’s not clear this isn’t optimal or even “unfair”.
So what?
Your paper is a study or measure of performance.
But as suggested almost immediately above, it seems hard (frankly, maybe even harmful) to measure performance if we don't take into account structures like "star systems", and probably many other complex factors.
Your intro, well written, is very clear and suggests we care about productivity because 1) it seems like a small number of people are very valuable and 2) suggests this in the most direct and useful sense of how EA orgs should hire.
Honestly, I took a quick scan (It’s 51 pages long! I’m willing to do more if there's specific need in the reply). But I know someone is experienced in empirical economic research, including econometrics, history of thought, causality, and how various studies, methodologies and world-views end up being adopted by organizations.
It’s hard not to pattern match this to something reductive like “Cross-country regressions”, which basically is inadequate (might say it’s an also-ran or reductive dead end).
Overall, you are measuring things like finance, number of papers, and equity, and I don’t see you making a comment or nod to the “Star systems” issue, which may be one of several structural concepts that are relevant.
To me, getting into performance/productivity/production functions seems to be a deceptively strong statement.
It would influence cultures and worldviews, and greatly worsen things, if for example, this was an echo-chamber.
Alternative / being constructive?
It's nice to try to end with something constructive.
I think this is an incredibly important area.
I know someone who built multiple startups and teams. Choosing the right people, from a cofounder to the first 50 hires is absolutely key. Honestly, it’s something akin to dating, for many of the same reasons.
So, well, like my 15 second response is that I would consider approaching this in a different way:
I think if the goal is help EA orgs, you should study successful and not successful EA orgs and figure out what works. Their individual experience is powerful and starting from interviews of successful CEOs and working upwards from what lessons are important and effective in 2021 and beyond in the specific area.
If you want to study exotic, super-star beyond-elite people and figure out how to find/foster/create them, you should study exotic, super-star beyond-elite people. Again, this probably involves huge amounts of domain knowledge, getting into the weeds and understanding multiple world-views and theories of change.
Well, I would write more but it's not clear there’s more 5 people who will read to this point, so I'll end now.
Also, here's a picture of a cat:
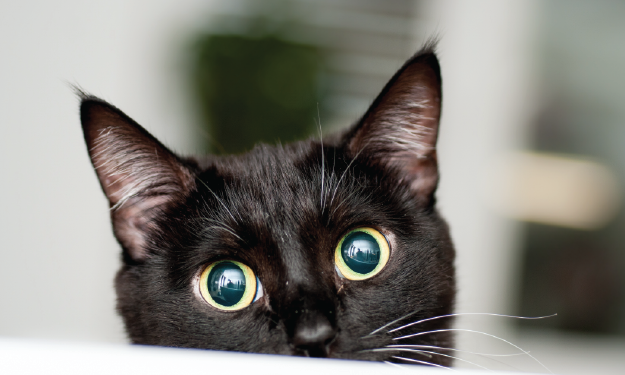
Ramiro @ 2021-05-31T16:52 (+9)
Your "star systems" point reminds me another problem which seems totally absent in this whole discussion - namely, things like agency conflicts and single-points-of-failure. For instance, I was reading about Alcibiades, and I'm pretty sure he was (one of) the most astonishing men alive in his age and overshadowed his peers - brilliant, creative, ridiculously gorgeous, persuasive, etc. Sorry for the cautionary tale: but he caused Athens to go to an unnecessary war, then defected to Sparta, & defected to Persia, prompted an oligarchic revolution in his homeland in order to return... and people enjoyed the idea because they knew he was awesome & possibly the only hope of a way out... then he let the oligarchy be replaced by a new democratic regime of his liking, became a superstar general who changed the course of the war, but then let his subordinate protégé lose a key battle because of overconfidence... and finally just exiled in his castle while the city lost the war.
I think one of the major advancements of our culture is that our institutions got less and less personal. So, while we are looking for star scientists, rulers, managers, etc. (i.e., a beneficious type of aristocracy) to leverage our output, we should also solve the resilience problems caused by agency conflicts and concentrating power and resources in few "points-of-failure".
(I mean, I know difference in perfomance is a complex factual question per se, without us having to worry about governance; I'm just pointing out that, for many relevant activities where differences in performance will be highlighted the most, we're likely to meet these related issues, and they should be taken into account if your organisation is acting based on "differences in performance are huge")
Charles He @ 2021-06-22T19:05 (+2)
Hey Ramiro,
I'm sorry but I just saw this comment now. My use of the forum can be infrequent.
I think your point is fascinating and your shift in perspective and using history is powerful.
I take your point about this figure and how disruptive (in the normal, typical sense of the word and not SV sense) he was.
I don't have much deep thoughts. I guess that it is true that institutions are more important now, at least for the reason since there's 8B people so single people should have less agency.
I am usually suspicious about stories like this since it's unclear how institutions and cultures are involved. But I don't understand the context well (classical period Greece). I guess they had https://en.wikipedia.org/wiki/Ostracism#Purpose for a reason.
vaidehi_agarwalla @ 2021-03-27T22:49 (+5)
On a meta-level and unrelated to the post, I very much appreciated the intro and the picture of the cat :)
Joseph Lemien @ 2023-11-17T19:10 (+4)
it's not clear there’s more 5 people who will read to this point
I just want to say that in late 2023, a few years after you wrote this, at least one person is still reading and appreciating your comments. :)
eca @ 2021-03-26T15:08 (+11)
Great post! Seems like the predictability questions is impt given how much power laws surface in discussion of EA stuff.
More precisely, future citations as well as awards (e.g. Nobel Prize) are predicted by past citations in a range of disciplines
I want to argue that things which look like predicting future citations from past citations are at least partially "uninteresting" in their predictability, in a certain important sense.
(I think this is related to other comments, and have not read your google doc, so apologies if I'm restating. But I think its worth drawing out this distinction)
In many cases I can think of wanting good ex-ante prediction of heavy-tailed outcomes, I want to make these predictions about a collection which is in an "early stage". For example, I might want to predict which EAs will be successful academics, or which of 10 startups seed rounds I should invest in.
Having better predictive performance at earlier stages gives you a massive multiplier in heavy-tailed domains: investing in a Series C is dramatically more expensive than a seed investment.
Given this, I would really love to have a function which takes in the intrinsic characteristics of an object, and outputs a good prediction of performance.
Citations are not intrinsic characteristics.
When someone is choosing who to cite, they look at - among other things- how many citations they have. All else equal, a paper/author with more citations will get cited more than a paper with less citations. Given the limited attention span of academics (myself as case in point) the more highly cited paper will tend to get cited even if the alternative paper is objectively better.
(Ed Boyden at MIT has this idea of "hidden gems" in the literature which are extremely undercited papers with great ideas: I believe the original idea for PCR, a molecular bio technique, had been languishing for at least 5 years with very little attention before later rediscover. This is evidence for the failure of citations to track quality.)
Domains in which "the rich get richer" are known to follow heavy-tailed distributions (with an extra condition or two) by this story of preferential attachment.
In domains dominated by this effect we can predict ex-ante that the earliest settlers in a given "niche" are most likely to end up dominating the upper tail of the power law. But if the niche is empty, and we are asked to predict which of a set would be able to set up shop in the niche--based on intrinsic characteristics--we should be more skeptical of our predictive ability, it seems to me.
Besides citations, I'd argue that many/most other prestige-driven enterprises have at least a non-negligible component of their variance explained by preferential attachment. I don't think it's a coincidence that the oldest Universities in a geography also seem to be more prestigious, for example. This dynamic is also present in links on the interwebs and lots of other interesting places.
I'm currently most interested in how predictable heavy-tailed outcomes are before you have seen the citation-count analogue, because it seems like a lot of potentially valuable EA work is in niches which don't exist yet.
That doesn't mean the other type of predictability is useless, though. It seems like maybe on the margin we should actually be happier defaulting to making a bet on whichever option has accumulated the most "citations" to date instead of trusting our judgement of the intrinsic characteristics.
Anyhoo- thanks again for looking into this!
Max_Daniel @ 2021-03-26T17:44 (+12)
Thanks! I agree with a lot of this.
I think the case of citations / scientific success is a bit subtle:
- My guess is that the preferential attachment story applies most straightforwardly at the level of papers rather than scientists. E.g. I would expect that scientists who want to cite something on topic X will cite the most-cited paper on X rather than first looking for papers on X and then looking up the total citations of their authors.
- I think the Sinatra et al. (2016) findings which we discuss in our relevant section push at least slightly against a story that says it's all just about "who was first in some niche". In particular, if preferential attachment at the level of scientists was a key driver, then I would expect authors who get lucky early in their career - i.e. publish a much-cited paper early - to get more total citations. In particular, citations to future papers by a fixed scientist should depend on citations to past papers by the same scientist. But that is not what Sinatra et al. find - they instead find that within the career of a fixed scientist the per-paper citations seem entirely random.
- Instead their model uses citations to estimate an 'intrinsic characteristic' that differs between scientists - what they call Q.
- (I don't think this is very strong evidence that such an intrinsic quality 'exists' because this is just how they choose the class of models they fit. Their model fits the data reasonably well, but we don't know if a different model with different bells and whistles wouldn't fit the data just as well or better. But note that, at least in my view, the idea that there are ability differences between scientists that correlate with citations looks likely on priors anyway, e.g. because of what we know about GMA/the 'positive manifold' of cognitive tasks or garden-variety impressions that some scientists just seem smarter than others.)
- Instead their model uses citations to estimate an 'intrinsic characteristic' that differs between scientists - what they call Q.
- The International Maths Olympiad (IMO) paper seems like a clear example of our ability to measure an 'intrinsic characteristic' before we've seen the analog of a citation counts. IMO participants are high school students, and the paper finds that even among people who participated in the IMO in the same year and got their PhD from the same department IMO scores correlate with citations, awards, etc. Now, we might think that maybe maths is extreme in that success there depends unusually much on fluid intelligence or something like that, and I'm somewhat sympathetic to that point / think it's partly correct. But on priors I would find it very surprising if this phenomenon was completely idiosyncratic to maths. Like, I'd be willing to bet that scores at the International Physics Olympiad, International Biology Olympiad, etc., as well as simply GMA or high school grades or whatever, correlate with future citations in the respective fields.
- The IMO example is particularly remarkable because it's in the extreme tail of performance. If we're not particularly interested in the tail, then I think some of studies on more garden-variety predictors such as GMA or personality we cite in the relevant section give similar examples.
eca @ 2021-03-28T01:13 (+4)
Interesting! Many great threads here. I definitely agree that some component of scientific achievement is predictable, and the IMO example is excellent evidence for this. Didn't mean to imply any sort of disagreement with the premise that talent matters; I was instead pointing at a component of the variance in outcomes which follows different rules.
Fwiw, my actual bet is that to become a top-of-field academic you need both talent AND to get very lucky with early career buzz. The latter is an instantiation of preferential attachment. I'd guess for each top-of-field academic there are at least 10 similarly talented people who got unlucky in the paper lottery and didn't have enough prestige to make it to the next stage in the process.
It sounds like I should probably just read Sinatra, but its quite surprising to me that publishing a highly cited paper early in one's career isn't correlated with larger total number of citations, at the high-performing tail (did I understand that right? Were they considering the right tail?). Anecdotally I notice that the top profs I know tend to have had a big paper/ discovery early. I.e. Ed Boyden who I have been thinking of because he has interesting takes on metascience, ~invented optogenetics in his PhD in 2005 (at least I think this was the story?) and it remains his most cited paper to this day by a factor of ~3.
On the scientist vs paper preferential attachment story, I could buy that. I was pondering while writing my comment how much is person-prestige driven vs. paper driven. I think for the most-part you're right that its paper driven but I decided this caches out as effectively the same thing. My reasoning was if number of citations per paper is power law-ish then because citations per scientist is just the sum of these, it will be dominated by the top few papers. Therefore preferential attachment on the level of papers will produce "rich get richer" on the level of scientists, and this is still an example of the things because its not an intrinsic characteristic.
That said, my highly anecdotal experience is that there is actually a per-person effect at the very top. I've been lucky to work with George Church, one of the top profs in synthetic biology. Folks in the lab literally talk about "the George Effect" when submitting papers to top journals: the paper is more attractive simply because George's name is on it.
But my sense is that I should look into some of the refs you provided! (thanks :)
Max_Daniel @ 2021-03-29T11:51 (+3)
its quite surprising to me that publishing a highly cited paper early in one's career isn't correlated with larger total number of citations, at the high-performing tail (did I understand that right? Were they considering the right tail?
No, they considered the full distribution of scientists with long careers and sustained publication activity (which themselves form the tail of the larger population of everyone with a PhD).
That is, their analysis includes the right tail but wasn't exclusively focused on it. Since by its very nature there will only be few data points in the right tail, it won't have a lot of weight when fitting their model. So it could in principle be the case that if we looked only at the right tail specifically this would suggest a different model.
It is certainly possible that early successes may play a larger causal role in the extreme right tail - we often find distributions that are mostly log-normal, but with a power-law tail, suggesting that the extreme tail may follow different dynamics.
eca @ 2021-03-28T01:32 (+1)
Sorry meant to write "component of scientific achievement is predictable from intrinsic characteristics" in that first line
Max_Daniel @ 2021-03-26T17:49 (+3)
Relatedly, you might be interested in these two footnotes discussing how impressive it is that Sinatra et al. (2016) - the main paper we discuss in the doc - can predict the evolution of the Hirsch index (a citation measure) over a full career based on the the Hirsch index after the 20 or 50 papers:
Note that the evolution of the Hirsch index depends on two things: (i) citations to future papers and (ii) the evolution of citations to past papers. It seems easier to predict (ii) than (i), but we care more about (i). This raises the worry that predictions of the Hirsch index are a poor proxy of what we care about – predicting citations to future work – because successful predictions of the Hirsch index may work largely by predicting (ii) but not (i). This does make Sinatra and colleagues’ ability to predict the Hirsch index less impressive and useful, but the worry is attenuated by two observations: first, the internal validity of their model for predicting successful scientific careers is independently supported by its ability to predict Nobel prizes and other awards; second, they can predict the Hirsch index over a very long period, when it is increasingly dominated by future work rather than accumulating citations to past work.
Acuna, Allesina, & Kording (2012) had previously proposed a simple linear model for predicting scientists’ Hirsch index. However, the validity of their model for the purpose of predicting the quality of future work is undermined more strongly by the worry explained in the previous footnote; in addition, the reported validity of their model is inflated by their heterogeneous sample that, unlike the sample analyzed by Sinatra et al. (2016), contains both early- and late-career scientists. (Both points were observed by Penner et al. 2013.)
eca @ 2021-03-28T01:28 (+3)
Neat. I'd be curious if anyone has tried blinding the predictive algorithm to prestige: ie no past citation information or journal impact factors. And instead strictly use paper content (sounds like a project for GPT-6).
It might be interesting also to think about how talent vs. prestige-based models explain the cases of scientists whose work was groundbreaking but did not garner attention at the time. I'm thinking, e.g. of someone like Kjell Keppe who basically described PCR, the foundational molbio method, a decade early.
If you look at natural experiments in which two groups publish the ~same thing, but only one makes the news, the fully talent-based model (I think?) predicts that there should not be a significant difference between citations and other markers of academic success (unless your model of talent is including something about marketing which seems like a stretch to me).
Max_Daniel @ 2021-03-29T11:44 (+2)
Ed Boyden at MIT has this idea of "hidden gems" in the literature which are extremely undercited papers with great ideas: I believe the original idea for PCR, a molecular bio technique, had been languishing for at least 5 years with very little attention before later rediscover.
A related phenomenon has been studied in the scientometrics literature under the label 'sleeping beauties'.
Here is what Clauset et al. (2017, pp. 478f.) say in their review of the scientometrics/'science of science' field:
However, some discoveries do not follow these rules, and the exceptions demonstrate that there can be more to scientific impact than visibility, luck, and positive feedback. For instance, some papers far exceed the predictions made by sim- ple preferential attachment (5, 6). And then there are the “sleeping beauties” in science: discoveries that lay dormant and largely unnoticed for long periods of time before suddenly attracting great attention (7–9). A systematic analysis of nearly 25 million publications in the natural and social sciences over the past 100 years found that sleeping beauties occur in all fields of study (9).
Examples include a now famous 1935 paper by Einstein, Podolsky, and Rosen on quantum me- chanics; a 1936 paper by Wenzel on waterproofing materials; and a 1958 paper by Rosenblatt on artificial neural networks. The awakening of slumbering papers may be fundamentally un- predictable in part because science itself must advance before the implications of the discovery can unfold.
[See doc linked in the OP for full reference.]
Lukas_Gloor @ 2021-03-29T13:03 (+4)
The awakening of slumbering papers may be fundamentally un- predictable in part because science itself must advance before the implications of the discovery can unfold.
Except to the authors themselves, who may often have an inkling that their paper is important. E.g., I think Rosenblatt was incredibly excited/convinced about the insights in that sleeping beauty paper. (Small chance my memory is wrong about this, or that he changed his mind at some point.)
I don't think this is just a nitpicky comment on the passage you quoted. I find it plausible that there's some hard-to-study quantity around 'research taste' that predicts impact quite well. It'd be hard to study because the hypothesis is that only very few people have it. To tell who has it, you kind of need to have it a bit yourself. But one decent way to measure it is asking people who are universally regarded as 'having it' to comment on who else they think also has it. (I know this process would lead to unfair network effects and may result in false negatives and so on, but I'm advancing a descriptive observation here; I'm not advocating for a specific system on how to evaluate individuals.)
Related: I remember a comment (can't find it anymore) somewhere by Liv Boeree or some other poker player familiar with EA. The commenter explained that monetary results aren't the greatest metric for assessing the skill of top poker players. Instead, it's best to go with assessments by expert peers. (I think this holds mostly for large-field tournaments, not online cash games.)
Max_Daniel @ 2021-03-29T15:11 (+6)
Related: I remember a comment (can't find it anymore) somewhere by Liv Boeree or some other poker player familiar with EA. The commenter explained that monetary results aren't the greatest metric for assessing the skill of top poker players. Instead, it's best to go with assessments by expert peers. (I think this holds mostly for large-field tournaments, not online cash games.)
If I remember correctly, Linchuan Zhang made or referred to that comment somewhere on the Forum when saying that it was similar with assessing forecaster skill. (Or maybe it was you? :P)
Linch @ 2021-03-29T20:35 (+4)
I have indeed made that comment somewhere. It was one of the more insightful/memorable comments she made when I interviewed her, but tragically I didn't end up writing down that question in the final document (maybe due to my own lack of researcher taste? :P)
That said, human memory is fallible etc so maybe it'd be worthwhile to circle back to Liv and ask if she still endorses this, and/or ask other poker players how much they agree with it.
alexrjl @ 2021-03-29T21:02 (+6)
I've been much less successful than LivB but would endorse it, though I'd note that there are substantially better objective metrics than cash prizes for many kinds of online play, and I'd have a harder time arguing that those were less reliable than subjective judgements of other good players. It somewhat depends on sample though, at the highest stakes the combination of v small playerpool and fairly small samples make this quite believable.
Lukas_Gloor @ 2021-03-29T13:14 (+2)
To give an example of what would go into research taste, consider the issue of reference class tennis (rationalist jargon for arguments on whether a given analogy has merit, or two people throwing widely different analogies at each other in an argument). That issue comes up a lot especially in preparadigmatic branches of science. Some people may have good intuitions about this sort of thing, while others may be hopelessly bad at it. Since arguments of that form feel notoriously intractable to outsiders, it would make sense if "being good at reference class tennis" were a skill that's hard to evaluate.
eca @ 2021-03-30T16:09 (+1)
Yeah this is great; I think Ed probably called them sleeping beauties and I was just misremembering :)
Thanks for the references!
Max_Daniel @ 2021-05-05T21:00 (+9)
[The following is a lightly edited response I gave in an email conversation.]
What is your overall intuition about positive feedback loops and the importance of personal fit? Do they make it (i) more important, since a small difference in ability will compound over time or (ii) less important since they effectively amplify luck / make the whole process more noisy?
My overall intuition is that the full picture we paint suggests personal fit, and especially being in the tail of personal fit, is more important than one might naively think (at least in domains where ex-post output really is very heavy-tailed). But also how to "allocate"/combine different resources is very important. This is less b/c of feedback loops specifically but more b/c of an implication of multiplicative models:
[So one big caveat is that the following only applies to situations that are in fact well described by a multiplicative model. It's somewhat unclear which these are.]
If ex-post output is very heavy-tailed, total ex-post output will be disproportionately determined by outliers. If ex-post output is multiplicative, then these outliers are precisely those cases where all of the inputs/factors have very high values.
So this could mean: total impact will be disproportionately due to people who are highly talented, have had lots of practice at a number of relevant skills, are highly motivated, work in a great & supportive environment, can focus on their job rather than having to worry about their personal or financial security, etc., and got lucky.
If this is right, then I think it adds interesting nuance on discussions around general mental ability (GMA). Yes, there is substantial evidence indicating that we can measure a 'general ability factor' that is a valid predictor for ~all more specific cognitive abilities. And it's useful to be aware of that, e.g. b/c almost all extreme outlier performers in jobs that rely crucially on cognitive abilities will be people with high GMA. (This is consistent with many/most people having the potential to be "pretty good" at these jobs.) However, conversely, it does NOT follow that GMA is the only thing paying attention to. Yes, a high-GMA person will likely be "good at everything" (because everything is at least moderately correlated). But there are differences in how good, and these really matter. To get to the global optimum, you really need to allocate the high-GMA person to a job that relies on whatever specific cognitive ability they're best at, supply all other 'factors of production' at maximal quality, etc. You have to optimize everything.
I.e. this is the toy model: Say we have ten different jobs, to . Output in all of them is multiplicative. They all rely on different specific cognitive abilities to (i.e. each is a factor in the 'production function' for but not the other). We know that there is a "general ability factor" that correlates decently well with all of the . So if you want to hire for any it can make sense to select on , especially if you can't measure the relevant directly. However, after you selected on you might end up with, say, 10 candidates who are all high on . It does NOT follow that you should allocate them at random between the jobs because " is the only thing that matters". Instead you should try hard to identify for any person which they're best at, and then allocate them to . For any given person, the difference between their and might look small b/c they're all correlated, but because output is multiplicative this "small" difference will get amplified.
(If true, this might rationalize the common practice/advice of: "Hire great people, and then find the niche they do best in.")
I think Kremer discusses related observations quite explicitly in his o-ring model. In particular, he makes the basic observation that if output is multiplicative you maximize total output by "assortative matching". This is basically just the observation that if and you have four inputs with etc., then
- i.e. you maximize total output by matching inputs by quality/value rather than by "mixing" high- and low-quality inputs. It's his explanation for why we're seeing "elite firms", why some countries are doing better than others, etc. In a multiplicative world, the global optimum is a mix of 'high-ability' people working in high-stakes environments with other 'high-ability' people on one hand, and on the other hand 'low-ability' people working in low-stakes environments with other 'low-ability' people. Rather than a uniform setup with mixed-ability teams everywhere, "balancing out" worse environments with better people, etc.
(Ofc as with all simple models, even if we think the multiplicative story does a good job at roughly capturing reality, there will be a bunch of other considerations that are relevant in practice. E.g. if unchecked this dynamic might lead to undesirable inequality, and the model might ignore dynamic effects such as people improving their skills by working with higher-skilled people, etc.)
Jan_Kulveit @ 2021-04-10T16:09 (+6)
1.
For different take on very similar topic check this discussion between me and Ben Pace (my reasoning was based on the same Sinatra paper).
For practical purposes, in case of scientists, one of my conclusions was
Translating into the language of digging for gold, the prospectors differ in their speed and ability to extract gold from the deposits (Q). The gold in the deposits actually is randomly distributed. To extract exceptional value, you have to have both high Q and be very lucky. What is encouraging in selecting the talent is the Q seems relatively stable in the career and can be usefully estimated after ~20 publications. I would guess you can predict even with less data, but the correct "formula" would be trying to disentangle interestingness of the problems the person is working on from the interestingness of the results.
2.
For practical purposes, my impression is some EA recruitment efforts could be more often at risk of over-filtering by ex-ante proxies and being bitten by tails coming apart, rather than at risk of not being selective enough.
Also, often the practical optimization question is how much effort you should spend on on how extreme tail of the ex-ante distribution.
3.
Meta-observation is someone should really recommend more EAs to join the complex systems / complex networks community.
Most of the findings from this research project seem to be based on research originating in complex networks community, including research directions such as "science of success", and there is more which can be readily used, "translated" or distilled.
Jsevillamol @ 2021-04-05T22:21 (+5)
Thank you for writing this!
While this is not the high note of the paper, I read with quite some interest your notes about heavy tailed distributions.
I think that the concept of heavy tailed distributions underpins a lot of considerations in EA, yet as you remark many people (including me) are still quite confused about how to formalize the concept effectively, and how often it applies in real life.
Glad to see more thinking going into this!
FlorianH @ 2021-04-01T04:12 (+5)
Surprised to see nothing (did I overlook?) about: The People vs. The Project/Job: The title, and the lead sentence,
Some people seem to achieve orders of magnitudes more than others in the same job.
suggest the work focuses essentially on people's performance, but already in the motivational examples
For instance, among companies funded by Y Combinator the top 0.5% account for more than ⅔ of the total market value; and among successful bestseller authors [wait, it's their books, no?], the top 1% stay on the New York Times bestseller list more than 25 times longer than the median author in that group.
(emphasis and [] added by me)
I think I have not explicitly seen discussed whether at all it is the people, or more the exact project (the startup, the book(s)) they work on, that is the successful element, although the outcome is a sort of product of the two. Theoretically, in one (obviously wrong) extreme case: Maybe all Y-Combinator CEOs were similarly performing persons, but some of the startups simply are the right projects!
My gut feeling is that making this fundamental distinction explicit would make the discussion/analysis of performance more tractable.
Addendum:
Of course, you can say, book writers and scientists, startuppers, choose each time anew what next book and paper to write, etc., and this choice is part of their 'performance', so looking at their output's performance is all there is. But this would be at max half-true in a more general sense of comparing the general capabilities of the persons, as there are very many drivers that lead persons to very specific high-level domains (of business, of book genres, etc.) and/or of very specific niches therein, and these may have at least as much to do with personal interest, haphazard personal history, etc.
Ben_West @ 2021-04-17T03:59 (+4)
Basic statistics question: the GMA predictors research seems to mostly be using the Pearson correlation coefficient, which I understand to measure linear correlation between variables.
But a linear correlation would imply that billionaires have an IQ of 10,000 or something which is clearly implausible. Are these correlations actually measuring something which could plausibly be linearly related (e.g. Z score for both IQ and income)?
I read through a few of the papers cited and didn't see any mention of this. I expect this to be especially significant at the tails, which is what you are looking at here.
Max_Daniel @ 2021-04-17T10:04 (+2)
Are these correlations actually measuring something which could plausibly be linearly related (e.g. Z score for both IQ and income)?
I haven't looked at the papers to check and don't remember but my guess would be that it's something like this. Plus maybe some papers looking at things other than correlation.
MaxRa @ 2021-04-02T12:20 (+4)
Nice, I think developing a deeper understanding here seems pretty useful, especially as I don't think the EA community can just copy the best hiring practices of existing institutions due to lack in shared goals (e.g. most big tech firms) or suboptimal hiring practices (e.g. non-profits & most? places in academia).
I'm really interested in the relation between the increasing number of AI researchers and the associated rate of new ideas in AI. I'm not really sure how to think about this yet and would be interested in your (or anybody's) thoughts. Some initial thoughts:
If the distribution of rates of ideas over all people that could do AI research is really heavy-tailed, and the people with the highest rates of ideas would've worked on AI even before the funding started to increase, maybe one would expect less of an increase in the rate of ideas (ignoring that more funding will make those researchers also more productive).
- my vague intuition here is that the distribution is not extremely heavy-tailed (e.g. the top 1% researchers with the most ideas contribute maybe 10% of all ideas?) and that more funding will capture many AI researchers that will end up landing in the top 10% quantile (e.g. every doubling of AI researchers will replace 2% of the top 10%?)
- I'm not sure to which if any distribution in your report I could relate the distribution of rates of ideas over all people who can do AI research. Number of papers written over the whole career might fit best, right? (see table extracted from your report)
Quantity | Share of the total held by the top ... | ||||
---|---|---|---|---|---|
20% | 10% | 1% | 0.1% | 0.01% | |
Papers written by scientist (whole career) [Sinatra et al. 2016] | 39% | 24% | 4.0% | .59% | .083% |
Max_Daniel @ 2021-04-02T13:00 (+6)
I'm really interested in the relation between the increasing number of AI researchers and the associated rate of new ideas in AI.
Yeah, that's an interesting question.
One type of relevant data that's different from looking at the output distribution across scientists is just looking at the evolution of total researcher hours on one hand and measures of total output on the other hand. Bloom and colleagues' Are ideas getting harder to find? collects such data and finds that research productivity, i.e. roughly output her researcher hour, has been falling everywhere they look:
The number of researchers required today to achieve the famous doubling of computer chip density is more than 18 times larger than the number required in the early 1970s. More generally, everywhere we look we find that ideas, and the exponential growth they imply, are getting harder to find.
MaxRa @ 2021-04-02T15:37 (+4)
Thanks, yes, that seems much more relevant. The cases in that paper feel slightly different in that I expect AI and ML to currently be much more "open" fields where I expect orders of magnitude more paths of ideas that can lead towards transformative AI than
- paths of ideas leading to higher transistor counts on a CPU (hmm, because it's a relatively narrow technology confronting physical extremes?)
- paths of ideas leading to higher crop yields (because evolution already invested a lot of work in optimizing energy conversion?)
- paths of ideas leading to decreased mortality of specific diseases (because this is about interventions in extremely complex biochemical pathways that are still not well understood?)
Maybe I could empirically ground my impression of "openness" by looking at the breadth of cited papers at top ML conferences, indicating how highly branched the paths of ideas currently are compared to other fields? And maybe I could look at the diversity of PIs/institutions of the papers that report new state-of-the-art results in prominent benchmarks, which indicates how easy it is to come into the field and have very good new ideas?
james_aung @ 2021-03-26T11:30 (+3)
Minor typo: "it’s often to reasonable to act on the assumption" probably should be "it’s often reasonable to act on the assumption"
Max_Daniel @ 2021-03-26T11:54 (+2)
Thanks! Fixed in post and doc.
John_Maxwell @ 2021-03-30T23:29 (+2)
It might be worth discussing the larger question which is being asked. For example, your IMO paper seems to be work by researchers who advocate looser immigration policies for talented youth who want to move to developed countries. The larger question is "What is the expected scientific impact of letting a marginal IMO medalist type person from Honduras immigrate to the US?"
These quotes from great mathematicians all downplay the importance of math competitions. I think this is partially because the larger question they're interested in is different, something like: "How many people need go into math for us to reap most of the mathematical breakthroughs that this generation is capable of?"