Complex Systems for AI Safety [Pragmatic AI Safety #3]
By TW123, Dan H @ 2022-05-24T00:04 (+49)
This is the third post in a sequence of posts that describe our models for Pragmatic AI Safety.
It is critical to steer the AI research field in a safer direction. However, it’s difficult to understand how it can be shaped, because it is very complex and there is often a high level of uncertainty about future developments. As a result, it may be daunting to even begin to think about how to shape the field. We cannot afford to make too many simplifying assumptions that hide the complexity of the field, but we also cannot afford to make too few and be unable to generate any tractable insights.
Fortunately, the field of complex systems provides a solution. The field has identified commonalities between many kinds of systems and has identified ways that they can be modeled and changed. In this post, we will explain some of the foundational ideas behind complex systems and how they can be applied to shaping the AI research ecosystem.
Along the way, we will also demonstrate that deep learning systems exhibit many of the fundamental properties of complex systems, and we show how complex systems are also useful for deep learning AI safety research.
A systems view of AI safety
Background: Complex Systems
When considering methods to alter the trajectory of empirical fields such as deep learning, as well as preventing catastrophe from higher risk systems, it is necessary to have some understanding of complex systems. Complex systems is an entire field of study, so we cannot possibly describe every relevant detail here. However, we will try to describe some of its most important aspects.
Complex systems are systems consisting of many interacting components that exhibit emergent collective behavior. Complex systems are highly interconnected, making decomposition and reductive analysis less effective: breaking the system down into parts and analyzing the parts cannot give a good explanation of the whole. However, complex systems are also too organized for statistics, since the interdependencies in the system break fundamental independence assumptions in much of statistics. Complex systems are ubiquitous: financial systems, power grids, social insects, the internet, weather systems, biological cells, human societies, deep learning models, the brain, and other systems are all complex systems.
For more background on complex systems, see this video. For background on emergence, a key property of complex systems, see this video.
It can be tricky to compare AI safety to making other specific systems safer. Is making AI safe like making a rocket, power plant, or computer program safe? While analogies can be found, there are many disanalogies. It’s more generally useful to talk about making complex systems safer. For systems theoretic hazard analysis, we can abstract away from the specific content and just focus on shared structure across systems. Rather than talk about what worked well for one high-risk technology, with a systems view we can talk about what worked well for a large number of them, which prevents us from overfitting to a particular example.
The central lesson to take away from complex systems theory is that reductionism is not enough. It’s often tempting to break down a system into isolated events or components, and then try to analyze each part and then combine the results. This incorrectly assumes that separation does not distort the system’s properties. In reality, parts do not operate independently, and are subject to feedback loops and nonlinear interactions. Analyzing the pairwise interactions between parts is not sufficient for capturing the full system complexity (this is partially why a bag of n-grams is far worse than attention).
Hazard analysis once proceeded by reductionism alone. In earlier models, accidents are broken down into a chain of events thought to have caused that accident, where a hazard is a root cause of an accident. Complex systems theory has supplanted this sort of analysis across many industries, in part because the idea of an ultimate “root cause” of a catastrophe is not productive when analyzing a complex system. Instead of looking for a single component responsible for safety, it makes sense to identify the numerous factors, including sociotechnical factors, that are contributory. Rather than break events down into cause and effect, a systems view instead sees events as a product of a complex interaction between parts.
For an in-depth explanation of contemporary hazard analysis that justifies them more completely than this post can, please see this lecture.
Recognizing that we are dealing with complex systems, we will now discuss how to use insights from complex systems to help make AI systems safer.
Improving Contributing Factors
“Direct impact,” that is impact produced from a simple, short, and deterministic causal chain, is relatively easy to analyze and quantify. However, this does not mean that direct impact is always the best route to impact. If someone only focuses on direct impact, they won’t optimize for diffuse paths towards impact. For instance, EA community building is indirect, but without it there would be far fewer funds, fewer people working on certain problems, and so on. Becoming a billionaire and donating money is indirect, but without this there would be significantly less funding. Similarly, safety field-building may not have an immediate direct impact on technical problems, but it can still vastly change the resources devoted to solving those problems, in turn contributing to solving them (note that “resources” does not (just) mean money, but rather competent researchers capable of making progress). In a complex system, such indirect/diffuse factors have to be accounted for and prioritized.
AI safety is not all about finding safety mechanisms, such as mechanisms that could be added to make superintelligence completely safe. This is a bit like saying computer security is all about firewalls, which is not true. Information assurance evolved to address blindspots in information security, because it is understood that we cannot ignore complex systems, safety culture, protocols, and so on.
Often, research directions in AI safety are thought to need to have a simple direct impact story: if this intervention is successful, what is the short chain of events towards it being useful for safe and aligned AGI? “How does this directly reduce x-risk” is a well-intentioned question, but it leaves out salient remote, indirect, or nonlinear causal factors. Such diffuse factors cannot be ignored, as we will discuss below.
A note on tradeoffs with simple theories of impact
AI safety research is complex enough that we should expect that understanding a theory of impact might require deep knowledge and expertise about a particular area. As such, a theory of impact for that research might not be easily explicable to somebody without any background in a short amount of time. This is especially true of theories of impact that are multifaceted, involve social dynamics, and require an understanding of multiple different angles of the problem. As such, we should not only focus on theories of impact that are easily explicable to newcomers.
In some cases, pragmatically one should not always focus on the research area that is most directly and obviously relevant. At first blush, reinforcement learning (RL) is highly relevant to advanced AI agents. RL is conceptually broader than supervised learning such that supervised learning can be formulated as an RL problem. However, the problems considered in RL that aren’t considered in supervised learning are currently far less tractable. This can mean that in practice, supervised learning may provide more tractable research directions.
However, with theories of impact that are less immediately and palpably related to x-risk reduction, we need to be very careful to ensure that research remains relevant. Less direct connection to the essential goals of the research may cause it to drift off course and fail to achieve its original aims. This is especially true when research agendas are carried out by people who are less motivated by the original goal of the research, and could potentially lead to value drift where previously x-risk-motivated researchers become motivated by proxy goals that are no longer relevant. This means that it is much more important for x-risk-motivated researchers and grantmakers to maintain the field and actively ensure research remains relevant (this will be discussed later).
Thus, there is a tradeoff involved in only selecting immediately graspable impact strategies. Systemic factors cannot be ignored, but this does not eliminate the need for understanding causal (whether indirect/nonlinear/diffuse or direct) links between research and impact.
Examples of the importance of systemic factors
The following examples illustrate the extreme importance of systemic factors (and the limitations of direct causal analysis and complementary techniques such as backchaining):
- Increasing wealth is strongly associated with a reduction in childhood mortality. But one cannot always credit the survival of any particular child to an increase of the wealth of their country. Nonetheless, a good way to reduce childhood mortality is still to increase overall wealth.
- Community building, improving institutions, and improving epistemics can usually not be linked directly to specific outcomes, but in aggregate they clearly have large effects.
- Smoking does not guarantee you will get cancer. If you smoke and get cancer, it is not necessarily because you smoked. Still, avoiding smoking is clearly a good way to avoid cancer. Contrariwise, exercise does not guarantee that you will be healthy, but it robustly helps.
- Intelligence (e.g. as measured by IQ) has an enormous impact on the ability of people to perform various tasks. But it is implausible to point to a particular multiple choice test question that somebody answered correctly and say “they got this question because their IQ was above x.” Similarly, forecasting and rationality could increase the “IQ” of the superorganism, but it similarly could not be expected to produce one single definite outcome. Improving the rationality waterline helps with outcomes, even if we cannot create a simple chain of events showing that it will prevent a particular future catastrophe.
- Any particular hurricane or wildfire cannot be attributed to the effects of climate change, but reducing climate change is a good way to reduce the prevalence of those extreme weather events.
In the cases above, it is possible to use statistics to establish the relationship between the variables given enough data. Some can be causally established through randomized controlled trials. However, we do not have the ability or time to run an RCT on diffuse factors that reduce x-risk from AI. Unlike the situations above, we do not get to observe many different outcomes because an existential catastrophe would be the last observation we would make. This does not mean diffuse factors are unimportant; on the contrary, they are extremely important. We can instead identify time-tested factors that have been robustly useful in similar contexts in the past.
On a more societal scale, the following diffuse factors are quite important for reducing AI x-risk. Note that these factors may interact in some cases: for instance, proactivity about risks might not help much if malevolent actors are in power.
- People having improved epistemics: Irrationality could cause people to ignore warning signs, dismiss correct claims, and barrel ahead when they shouldn’t.
- Proactivity about (tail) risks: Causing humanity as a collective to care more about tail risks would be a boon for safety. Work on mitigating tail risks is currently underincentivized due to the human tendency to ignore tail risks.
- Expanded moral circles: The term “moral circle” describes the beings that one considers to be morally relevant (e.g. people in your community, people across the world, future people, non-human animals, etc.). People do not need a large moral circle to want to avoid their own deaths, but it can strengthen the perceived importance of reducing x-risk.
- Keeping (misaligned) malevolent actors (egoists/Machiavellians/psychopaths) out of power: Contending with actively malevolent leaders is even more difficult than contending with apathetic leaders. Getting even-handed, cautious, and altruistic people into positions of power is likely to reduce x-risk.
Sociotechnical Factors
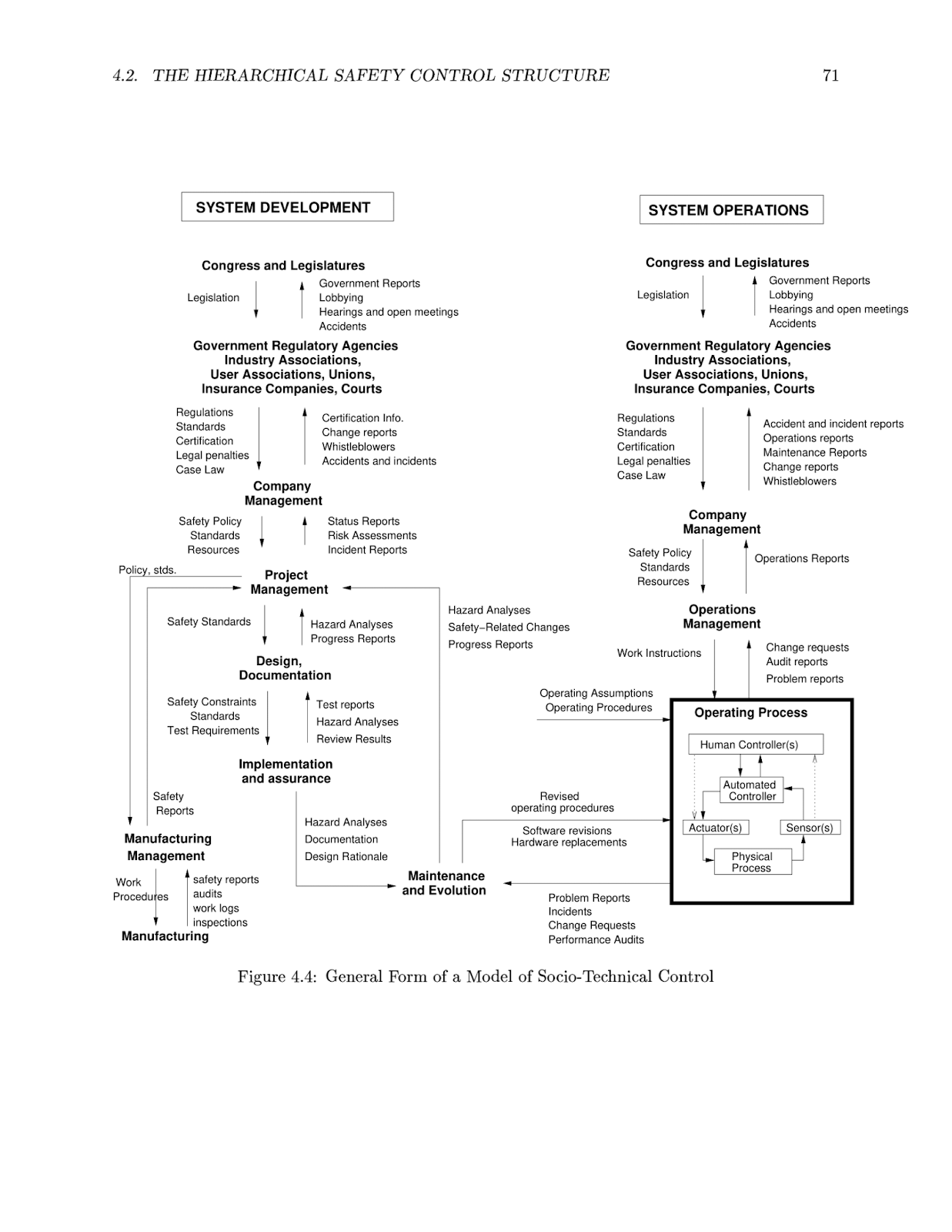
We can now speak about specific diffuse factors that have shown to be highly relevant to making high-risk technological systems safer, which are also relevant to making present and future AI systems safer. The following sociotechnical factors (compiled from Perrow, La Porte, Leveson, and others) tend to influence hazards:
- Rules and regulations, perhaps including internal policies as well as legal governance.
- Social pressures, including those from the general public as well as well-connected powerful people.
- Productivity pressures, or pressure to deliver quickly.
- Incentive structures within the organization, such as benefits to delivering quickly or retaliation for whistleblowing.
- Competition pressures from other actors who may have different safety standards, or otherwise be able to move faster.
- Safety budget and compute allocation: are safety teams capable of running the experiments they need to? Is a significant proportion of the budget and compute dedicated to safety?
- Safety team size, which is related to budget. The number of researchers, engineers, and top researchers on the safety team matters a lot.
- Alarm fatigue: if many false alarms are raised about safety issues which were never borne out, this could reduce willingness to care about safety.
- Reduction in inspection and preventative maintenance, which is perhaps less relevant for a forward-looking problem like safety. However, if people do not keep a close eye on capabilities, this could allow for emergent capabilities (or actors) to take us by surprise.
- Lack of defense in depth: overlapping systems that provide multiple layers of defense against hazards.
- Lack of redundancy: multiple systems which accomplish similar safety tasks, so as to remove single points of failure.
- Lack of fail-safes: features that allow a system to fail gracefully.
- Safety mechanism cost: how much does it cost to make a system safe?
- Safety culture, or the general attitude towards safety within an organization or field.
According to Leveson, who has been consulted on the design of high-risk technologies across numerous industries, “the most important [contributing factor] to fix if we want to prevent future accidents” is safety culture.
Safety Culture
Safety culture is not an easy risk factor to address, though it is likely to be one of the most important. Many ML researchers currently roll their eyes when asked about alignment or safety: usually, one cannot simply go straight to discussing existential risks from superintelligences without suffering social costs or efforts potentially backfiring. This is a sign of a deficient safety culture.
How do we improve safety culture? Safety needs to be brought to the forefront through good incentive structures and serious research. Pushing research cultures in a safer direction is bottlenecked by finding interesting, shovel-ready, safety-relevant tasks for people to do and funding them to complete those tasks.
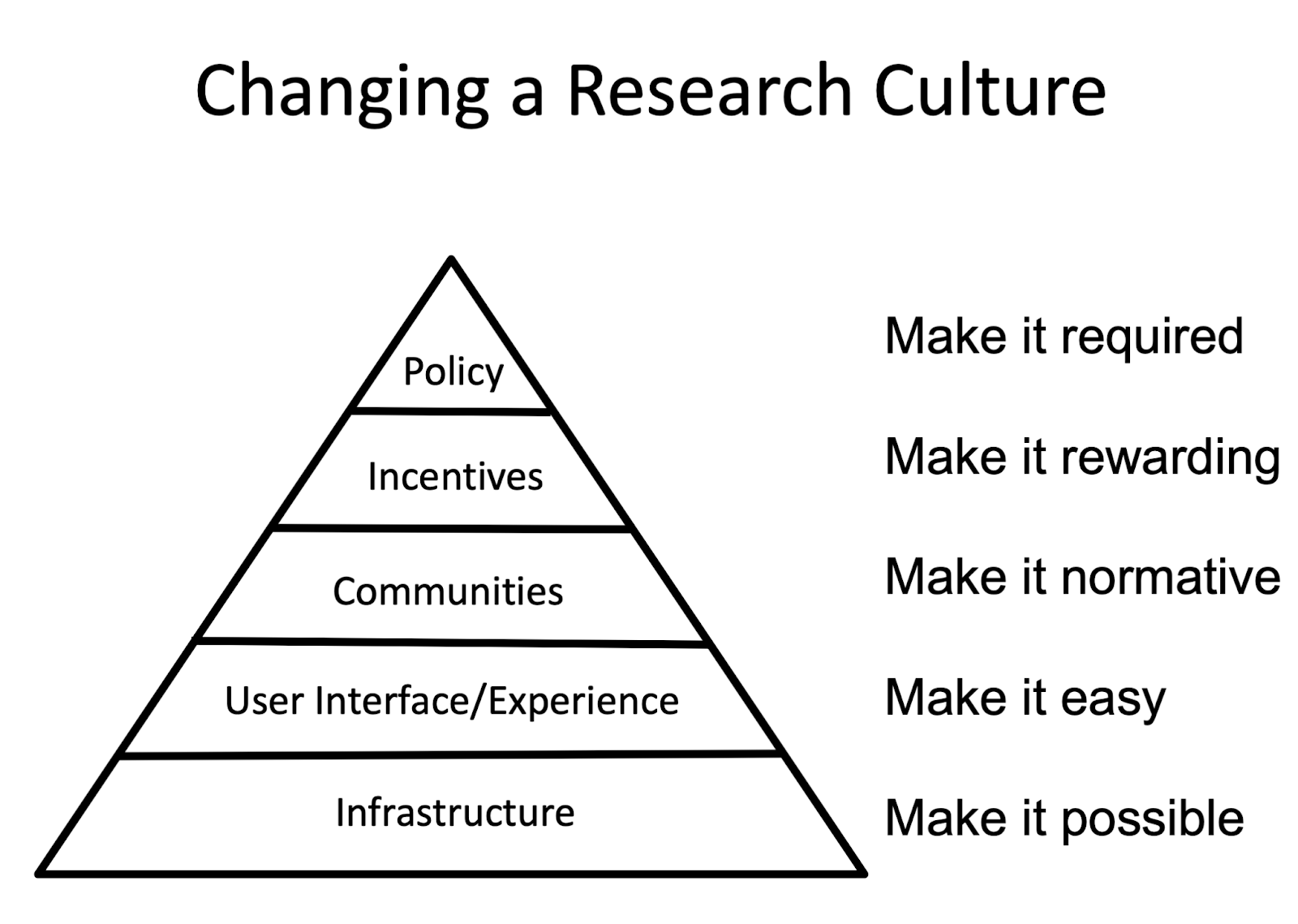
As suggested by the speculative pyramid above, it is not realistic to immediately try to make safety into a community norm. Before this can be done, we need to make it clear what safety looks like and we need infrastructure to make AI safety research as easy as possible. Researchers need to accept arguments about risks and they need clear, concrete, low-risk research tasks to pursue. This involves creating funding opportunities, workshops, and prizes, as well as clearly defining problems through metrics.
Some contributing factors that can improve safety culture are as follows:
- Preoccupation with failure, especially black swan events and unseen failures.
- Reluctance to simplify interpretations and explain failures using only simplistic narratives.
- Sensitivity to operations, which involves closely monitoring systems for unexpected behavior.
- Commitment to resilience, which means being rapidly adaptable to change and willing to try new ideas when faced with unexpected circumstances.
- Under-specification of organizational structures, where new information can travel throughout the entire organization rather than relying only on fixed reporting chains.
For mainstream culture, public outreach can help. One plausible way that AI systems could become more safe is due to a broader cultural desire for safety, or fear of lack of safety. Conversely, if AI safety is maligned or not valued in the general public, there may be other public pressures (e.g. winning the AI race, using AI to achieve some social good quickly) that could push against safety. Again, mainstream outreach should not be so extreme as to turn the research community against safety. Overton windows must be shifted with care.
Currently, safety is being attacked by critics who believe that it detracts from work on AI fairness and bias and does not heavily prioritize current power inequalities, which they view as the root cause of world problems. Criticisms have been connected to criticisms of longtermism, particularly absurd-seeming expected value calculations of the number of future beings, as well as the influence of EA billionaires. These criticisms threaten to derail safety culture. It is tricky but necessary to present an alternative perspective while avoiding negative side effects.
Some technical problems are instrumentally useful for safety culture in addition to being directly useful for safety. One example of this is reliability: building highly reliable systems trains people to specifically consider the tail-risks of their system, in a way that simply building systems that are more accurate in typical settings does not. On the other hand, value learning, while it is also a problem that needs to be solved, is currently not quite as useful for safety culture optimization.
Composition of top AI researchers
We will now discuss another contributing factor that is important to improve: the composition of top AI researchers. In the future, experimenting with the most advanced AI systems will be extraordinarily expensive (in many cases, it already is). A very small number of people will have the power to set research directions for these systems. Though it’s not possible to know exactly who will be in this small group, it could comprise any number of the top AI researchers today. However, one thing is known: most top AI researchers are not sympathetic to safety. Consequently, there is a need to increase the proportion of buy-in among top researchers, especially including researchers in China, and also to train more safety-conscious people to be top researchers.
It’s tempting to think that top AI researchers can simply be bought. This is not the case. To become top researchers, they had to be highly opinionated and driven by factors other than money. Many of them entered academia, which is not a career path typically taken by people who mainly care about money. Yann LeCun and Geoffrey Hinton both still hold academic positions in addition to their industry positions at Meta and Google, respectively. Yoshua Bengio is still in academia entirely. The tech companies surely would be willing to buy more of their time for a higher price than academia, so why are the three pioneers of deep learning not all in the highest-paying industry job? Pecuniary incentives are useful for externally motivated people, but many top researchers are mostly internally motivated.
As discussed in the last post, a leading motivation for researchers is the interestingness or “coolness” of a problem. Getting more people to research relevant problems is highly dependent on finding interesting and well-defined subproblems for them to work on. This relies on concretizing problems and providing funding for solving them.
Due to the fact that many top researchers are technopositive, they are not motivated by complaints about the dangers of their research, and they are likely to be dismissive. This is especially true when complaints come from those who have not made much of a contribution to the field. As a result, it is important to keep the contribution to complaint ratio high for those who want to have any credibility. “Contribution” can be a safety contribution, but it needs to be a legible contribution to ML researchers. Top researchers may also associate discussion of existential risk with sensationalist stories in the media, doom-and-gloom prophecies, or panic that “we’re all going to die.”
Causes of Neglectedness
There are a number of additional factors which contribute to the general neglectedness of AI safety. It is important to optimize many of these factors in order to improve safety. A more general list of these factors is as follows.
- Corporate: myopic desire for short-term shareholder returns, safety features may take a long time to pay off, some human values may be difficult to incorporate in prices or pecuniary incentives
- Temperamental: techno-optimism, distaste for discussing risks
- Political: AI safety is seen to compete with more politically popular causes like climate change and reducing inequality
- Technical Background: safety problems are outside of one’s existing skill set or training, and likewise machine ethics and sociotechnical concerns and do not as easily as easily comport with their quantitative inclinations
- Socioeconomic distance: many AI researchers live in tech bubbles, which can cause researchers to devalue or implicitly underemphasize cosmopolitan approaches towards loading human values
- Tail risks: highly consequential black swans and tail risks are systematically neglected
- Respectability: distaste for talk of AGI, feeling an area is not prestigious, areas associated with people who hold other unpopular or weird-seeming ideas
- Temporal: future risks and future people are highly neglected
Complex Systems for AI Safety
Complex systems studies emphasizes that we should focus on contributing factors (as events are the product of the interaction of many contributing factors), and it helps us identify which contributing factors are most important across many real-world contexts. They also provide object-level insight about deep learning, since deep learning systems are themselves complex systems.
Deep learning exhibits many halmarks of complex systems:
- Highly distributed functions: partial concepts are encoded redundantly and highly aggregated
- Numerous weak nonlinear connections: Connection parameters are nonzero (rather than sparse) and neural networks contain nonlinear activation functions
- Self-organization: optimizing a loss automatically specifies a model’s internal content
- Adaptivity: few-shot models and online models are adaptive
- Feedback loops: Self-play, human in the loop, auto-induced distribution shift
- Scalable structure: scaling laws show that models scale simply and consistently
- Emergent capabilities: numerous unplanned capabilities spontaneously “turn on”
As such, insights from complex systems are quite applicable to deep learning. Similarly, like all large sociotechnical structures, the AI research community can also be considered to be a complex system. The organizations operating AI systems are also complex systems.
Complex systems is a predictive—not just explanatory—model for various problems, including AI safety. In fact, many important concepts in AI safety turn out to be specific instances of more general principles. Here are examples of highly simplified lessons from complex systems, mostly from The Systems Bible (1975):
- Systems develop goals of their own the instant they come into being.
- Explanation: A system’s goal is seldom merely the initial goal it was tasked with. Rather, other goals emerge from the organization of the system.
- Implications for AI: One salient example are instrumental goals for self-preservation or power-seeking.
- Intrasystem goals come first.
- Explanation: Systems often decompose goals into subparts for different intrasystem components to solve. During this decomposition, goals are often distorted. A common failure mode is that the system's explicitly written objective is not necessarily the objective that the system operationally pursues, and this can result in misalignment. A system’s subgoals can supersede its actual goals. For example, a bureaucratic department (a subsystem) can capture power and have the company pursue goals unlike its original goals.
- Implications for AI: A related phenomenon is already well known to the community as mesa-optimization; it has been predicted on a more general level by systems theory for decades.
- The mode of failure of a complex system cannot ordinarily be predicted from its structure.
- Explanation: Simply examining a complex system will not necessarily give you a good idea for how it might fail. Failures are usually identified from experience and testing.
- Implications for AI: It is difficult to understand how all the ways a neural network might fail simply by examining its weights or architecture or through armchair/whiteboard analysis. We can count on some failures being unpredictable. (Although failures are inevitable, catastrophes are not.)
- Implications for strategy: An approach of “think about the problem really hard and make sure there are no holes in the solution” is unlikely to turn up a solution that truly has no holes. Preventing failure in a complex system is not a math problem. In complex systems there are few symmetries, few necessary and sufficient conditions or boolean connectives (no root cause), circular relationships, numerous partial concepts (combinatorial explosion), self-organization, high distributivity. All of these properties make complex systems very difficult to analyze from an armchair/whiteboard or with proofs.
- The crucial variables are discovered by accident.
- Explanation: It is difficult to know what the most important parts of a system are by inspection. The highest points of leverage are not obvious. Likewise, the methods that will work best are often found by tinkering or serendipity.
- Implications for AI: Many of the greatest breakthroughs in AI are not discovered purely by principled, highly structured investigation, but instead by tinkering.
- Implications for strategy: Many current approaches to research bet on AGI being best represented as a mathematical object rather than a complex system, which seems unrealistic given current AI systems as well as other intelligent systems we know (e.g. humans, corporations).
- A large system, produced by expanding the dimensions of a smaller system, does not behave like the smaller system.
- Explanation: Purely scaling up a system does not only make it better at whatever it was doing before. We should expect to see new qualitative properties and emergent capabilities.
- Implications for AI: We should expect to also see emergent capabilities that did not exist at all in smaller versions. For example, at low levels of capabilities, deception is not a good idea for an intelligence, but as it becomes more intelligent, deception may be a better strategy for achieving goals.
- Implications for strategy: Scaling up an aligned system and expecting it to be fully aligned is not an airtight idea. Scaling, even of a highly reliable system, needs to be done carefully.
- (From Gilb) Gilb’s Laws of Unreliability: any system which depends on human reliability is unreliable.
- Explanation: Humans are not reliable. Reliance on them will create unreliability.
- Implications for strategy: AI systems may be too explosive and fast-moving for depending heavily on human feedback or human-in-the-loop methods. We will need a more reliable strategy for preserving human values, perhaps through oversight from other AI systems.
- A complex system that works is invariably found to have evolved from a simple system that works.
- Explanation: Complex systems cannot be created from scratch and expected to work. Rather, they have to evolve from simpler functioning systems.
- Implications for strategy: Working on safety for simpler systems, and attempting to (carefully) scale them up is more likely to be successful than starting by trying to build an aligned complex system from scratch. Although systems behave differently when scaled, the ones that work are evolved from smaller systems. If one is unable to align a simpler version of a complex system, it is unlikely that one can align the more complex version. On this view a top priority is making today’s simpler systems safer.
Diversification
There are many different facets involved in making complex systems work well; we cannot simply rely on a single contributing factor or research direction. The implication is that it makes sense to diversify our priorities.
Since an individual has limited ability to become specialized and there are many individuals, it often makes sense to bet on the single highest expected value (EV) research approach. However, it would be a mistake to think of the larger system in the same way one thinks of an individual within the system. If the system allocates all resources into the highest EV option, and that sole option does not pay off, then the system fails. This is a known fact in finance and many other fields that take a portfolio approach to investments. Do not make one big bet or only bet on the favorite (e.g., highest estimated EV) avenue. The factor with the highest return on investment in isolation is quite different from the highest return on investment profile spanning multiple factors. The marginal benefit of X might be higher than Y, but the system as a whole is not forced to choose only one. As the common adage goes, “don’t put all your eggs in one basket.”
One example of obviously suboptimal resource allocation is that the AI safety community spent a very large fraction of its resources on reinforcement learning until relatively recently. While reinforcement learning might have seemed like the most promising area for progress towards AGI to a few of the initial safety researchers, this strategy meant that not many were working on deep learning. Deep learning safety researchers were encouraged to focus on RL environments because it is “strictly more general,” but just because one can cast a problem as a reinforcement learning problem does not mean one should. At the same time, the larger machine learning community focused more on deep learning than reinforcement learning. Obviously, deep learning appears now to be at least as promising as reinforcement learning, and a lot more safety research is being done in deep learning. Due to tractability, the value of information, iterative progress in research, and community building effects, it might have been far better had more people been working on deep learning from an earlier date. This could readily have been avoided had the community leaders heeded the importance of heavily diversifying research.
If we should address multiple fronts simultaneously, not bet the community on a single area or strategy, we will pay lower costs from neglecting important variables. Since costs often scale superlinearly with the time a problem has been neglected, which has serious practical implications, it makes sense to apply resources to pay costs frequently, rather than only applying resources after costs have already blown up. The longer one waits, the more difficult it could be to apply an intervention, and if costs are convex (e.g. quadratic rather than logarithmic), costs are exacerbated further. Diversification implicitly keeps these costs lower.
AI safety is an area with extremely high uncertainty: about what the biggest problems will be, what timelines are, what the first AGI system will look like, etc. At the highest levels of uncertainty, it is most important to improve the virtues of the system (e.g., meritocratic structures, sheer amount of talent, etc.). If your uncertainty level is slightly less, you additionally want to make a few big bets and numerous small bets created in view of a range of possible futures. Moreover, under high uncertainty or when work is inchoate, it is far more effective to follow an “emergent strategy,” not define the strategy with a highly structured, perfected direction.
With diversification, we do not need to decisively resolve all of the big questions before acting. Will there be a slow takeoff, or will AI go foom? Are the implicit biases in SGD beneficial to us, or will they work against us? Should we create AI to pursue a positive direction, or should we just try to maximize control to prevent it from taking over? So long as answers to these questions are not highly negatively correlated, we can diversify our bets and support several lines of research. Additionally, research can help resolve these questions and can inform which future research should be included in the overall portfolio. Seeing value in diversification saves researchers from spending their time articulating their tacit knowledge and highly technical intuitions to win the court of public opinion, as perhaps the question cannot be resolved until later. Diversification makes researchers less at odds with each other and lets them get on with their work, and it reduces our exposure to risks from incorrect assumptions.
Diversification does not mean that one should not be discretionary about ideas. Some ideas, including those commonly pursued in academia and industry, may not be at all useful to x-risk, even if they are portrayed that way. Just because variables interact nonlinearly does not mean that resources should be devoted to a variable that is not connected with the problem.
In addition, individuals do not necessarily need to have a diverse portfolio. There is a benefit to specialization, and so individuals may be better off choosing a single area where they are likely to reach the tail of impact through specialization. However, if everyone individually focused on what they viewed as the most important area of research overall, and their judgments on this were highly correlated, we would see a concentration of research into only a few areas. This would lead to problems, because even if these areas are the most important, they should not be single-mindedly pursued to the neglect of all other interventions.
In complex systems, we should expect many multiplicatively interacting variables to be relevant to the overall safety of a system (we will discuss this model more in the next post). If we neglect other safety factors only to focus on “the most important one,” we are essentially setting everything else to zero, which is not how one reduces the probability of risk in a multiplicative system. For instance, we should not just focus on creating technical safety solutions, let alone betting on one main technical solution. There are other variables that can be expected to nonlinearly interact with this variable: the cost of such a system, the likelihood of AGI being developed in a lab with a strong safety culture, the likelihood of other actors implementing an unaligned version, and the likelihood of the aligned system in question being the one that actually leads to AGI. These interactions and interdepencies imply that effort must be expended to push on all factors simultaneously. This can also help provide what is called defense in depth: if one measure for driving down x-risk fails, other already existing measures can help handle the problem.
Like many outcomes, impact is long tailed, and the impact of a grant will be dominated by a few key paths to impact. Likewise, in a diverse portfolio, the vast majority of the impact will likely be dominated by a few grants. However, the best strategies will sample heavily from the long tail distribution, or maximize exposure to long tail distributions. Some ways to increase exposure to the black swans are with broad interventions that could have many different positive impacts, as well as a larger portfolio of interventions. This contrasts with an approach that attempts to select only targeted interventions in the tails, which is often infeasible in large, complex systems because the tails cannot be fully known beforehand. Instead, one should prioritize interventions that have a sufficient chance of being in the tails.
Depending on what phase in the development of AI we are, targeted or broad interventions should be more emphasized in the portfolio. In the past, broad interventions would clearly have been more effective: for instance, there would have been little use in studying empirical alignment prior to deep learning. Even more recently than the advent of deep learning, many approaches to empirical alignment were highly deemphasized when large, pretrained language models arrived on the scene (refer to our discussion of creative destruction in the last post). Since the deep learning community is fairly small, it is relatively tractable to work on broad interventions (in comparison to e.g. global health, where interventions will need to affect millions of people).
At this stage, targeted interventions to align particular systems are not currently likely to deliver all the impact, nor are broad approaches that hope to align all possible systems. This is because there is still immense upside in optimizing contributing factors to good research, which will in turn cause both of these approaches to be dramatically more effective. The best interventions will look less like concrete stories for how the intervention impacts a particular actor during the creation of AGI and more like actions that help to improve the culture/incentives/buy-in of several possible actors
This suggests that a useful exercise might be coming up with broad interventions that equip the safety research field to deal with problems more effectively and be better placed to deliver targeted interventions in the future. Note that some broad interventions, like interventions that affect safety culture, are not simply useful insofar as they accelerate later targeted interventions, but also in that they may increase the likelihood of those targeted interventions being successfully adopted.
We also need to have targeted interventions, and they may need to be developed before they are known to be needed due to the risk of spontaneously emergent capabilities. There is also an argument that developing targeted interventions now could make it easier to develop targeted interventions in the future. As a result, a mixture of targeted and broad interventions is needed.
Conclusion
It can be daunting to even begin to think how to influence the AI research landscape due to its size and complexity. However, the study of complex systems illuminates some common patterns that can help make this question more tractable. In particular, in many cases it makes more sense to focus on improving contributing factors rather than only try to develop a solution that has a simple, direct causal effect on the intended outcome. Complex systems are also useful for understanding machine learning safety in general, since both the broader research community, deep learning systems, and the organizations deploying deep learning systems are all complex systems.
Resources on Complex Systems
Complex systems is a whole field of study that can't possibly be fully described in this post. We've added this section with resources for learning more.
- (If you only look at one, look at this:) An introduction to contemporary hazard analysis that justifies the methods far more completely than this post can.
- A short video introduction to complex systems.
- A short video introduction to emergence, a key property of complex systems.
- Systemantics by John Gall, one of the foundational texts of complex systems.
- A class introduction to complex systems.
Rory Greig @ 2022-05-26T13:43 (+2)
Thanks for writing this, in my opinion the field of complex systems provides a useful and under-explored perspective and set of tools for AI safety. I particularly like the insights you provide in the "Complex Systems for AI Safety" section, for example that ideas in complex systems foreshadowed inner alignment / mesa-optimisation.
I'd be interested in your thoughts on modelling AGI governance as a complex system, for example race dynamics.
I previously wrote a forum post on how complex systems and simulation could be a useful tool in EA for improving institutional decision making, among other things: https://forum.effectivealtruism.org/posts/kWsRthSf6DCaqTaLS/what-complexity-science-and-simulation-have-to-offer
Jay Bailey @ 2022-05-24T00:54 (+2)
Possibly a newbie question: I noticed I was confused about the paragraph around deep learning vs. reinforcement learning.
"One example of obviously suboptimal resource allocation is that the AI safety community spent a very large fraction of its resources on reinforcement learning until relatively recently. While reinforcement learning might have seemed like the most promising area for progress towards AGI to a few of the initial safety researchers, this strategy meant that not many were working on deep learning."
I thought that reinforcement learning was a type of deep learning. My own understanding is that deep learning is any form of ML using multilayered neural networks, and that reinforcement learning today uses multilayered neural networks, and thus could be called "deep reinforcement learning", but is generally just RL for short. If that were true that would mean RL research was also DL research.
Am I misunderstanding some of the terminology?
ThomasWoodside @ 2022-05-26T17:33 (+3)
The terminology around AI (AI, ML, DL, RL) is a bit confused sometimes. You're correct that deep reinforcement learning does indeed use deep neural nets, so it could be considered a part of deep learning. However, colloquially deep learning is often taken to mean the parts that aren't RL (so supervised, unsupervised, and self-supervised deep learning). RL is pretty qualitatively different from those in the way it is trained, so it makes sense that there would be a different term, but it can create confusion.
anonymous6 @ 2022-05-26T17:57 (+2)
One way I think it is plausible to draw lines between RL/core DL is that post-AlphaGo a lot of people were very bullish on specifically deep networks + reinforcement learning. Part of the idea was that supervised learning required inordinately costly human labeling, whereas RL would be able to learn from cheap simulations and even improve itself online in the world. OpenAI was originally almost 100% RL-focused. That thread of research is far from dead but it has certainly not panned out the way people hoped at the time (e.g. OpenAI has shifted heavily away from RL).
Meanwhile non-RL deep learning methods, especially generative models that kind of sidestep the labeling issue, have seen spectacular success.
philgoetz @ 2022-11-17T07:33 (+1)
I was hoping for an essay about deliberately using nonlinear systems in constructing AI, because they can be more-stable than the most-stable linear systems if you know how to do a good stability analysis. This was instead an essay on using ideas about nonlinear systems to critique the AI safety research community. This is a good idea, but it would be very hard to apply non-linear methods to a social community. The closest thing I've seen to doing that was the epidemiological models used to predict the course of Covid-19.
The essay says, "The central lesson to take away from complex systems theory is that reductionism is not enough. It’s often tempting to break down a system into isolated events or components, and then try to analyze each part and then combine the results. This incorrectly assumes that separation does not distort the system’s properties." I hear this a lot, but it's wrong. It assumes that reductionism is linear--that you want to break a nonlinear system into isolated components, then relate them to each other with linear equations.
Reductionism can work on nonlinear systems if you use statistics, partial differential equations, and iteration. Epidemiological models and convergence proofs for neural networks are examples. Both use iteration, and may give only statistical claims, so you might still say "reductionism is not enough" if you want absolute certainty, e.g., strict upper bounds on distributions. But absolute certainty is only achievable in formal systems (unapplied math and logic), not in real life.
The above essay seems to me to be trying to use linear methods to understand a nonlinear system, decomposing it into separable heuristics and considerations to be attended to, such as the line-items in the flow charts and bulleted lists above. That was about the best you could do, given the goal of managing the AI safety community.
I'd really like to see you use your understanding of complex systems either to try to find some way of applying stability analysis to different AI architectures, or to study the philosophical foundations of AI safety as it exists today. The latter use assumptions of linearity, analytic solvability, distrust of noise and evolution, and a classical (i.e., ancient Greek) theory of how words work, which expects words to necessarily have coherent meanings, and for those meanings to have clear and stable boundaries, and requires high-level foundational assumptions because the words are at a high level of abstraction. This is all especially true of ideas that trace back to Yudkowsky. I think these can all be understood as stemming from over-simplifications required for linear analysis. They're certainly strongly correlated with it.
I dumped a rant that's mostly about the second issue (the metaphysics of the AI safety community today) onto this forum recently, here, which is a little more specific, though I fear perhaps still not specific enough to be better than saying nothing.
Artyom K @ 2022-10-20T14:50 (+1)
Thanks for publishing this and your research! Few discussion points:
1. It is unclear how can we apply the safety culture in our current highly competitive environment (Google vs Facebook, China vs USA). What concretely should be the incentives or policies to adopt a safety culture? And who enforces them? If one adopts it, another will get a competitive advantage as they will spend more on capabilities and then 'kill you' (Yudkowsky, AGI Ruin).
2. Extremely high stakes, i.e. x-risk. While systems theory was developed for dangerous, mission-critical systems, it didn’t deal with those systems that might disempower all humanity forever. We don’t have a second try. So no use of systems theory? It should be an iterative process, but misaligned AI would kill us in a first wrong try?
3. Systems Theory developed for systems built by humans for humans. And humans have a certain limited intelligence level. Why is it true that it is likely that Systems Theory is applicable for a intelligence above human one?
4. Systems Theory implies the control of a better entity on a worse entity: government issues policies to control organizations, AI lab stops researches on a dangerous path, electrician complies with instructions, etc. Now, isn’t AGI a better entity to give control to? Does it imply the humanity's dis-empowerment? Particularly, when we introduce a moral parliament (discussed in PAIS #4) won’t it mean that now this parliament is in power, not humanity?