Mass media interventions probably deserve more attention (Founders Pledge)
By Rosie_Bettle @ 2022-11-17T15:01 (+146)
Acknowledgments
I would like to thank Matt Lerner for insightful comments and advice on earlier drafts. I would further like to thank everyone at Founders Pledge who bounced ideas around with me, and who gave useful comments and questions during an earlier presentation upon this topic.
(This shallow report is viewable as a google doc here, and has BOTECs here.)
Executive Summary
Mass media campaigns aim to promote behavior change (for example, to reduce rates of smoking or drinking alcohol) via programming over different media sources, such as radio and TV. Mass media interventions have not generally been prioritized within the global health field (e.g. see GiveWell’s 2012 report here), in part due to mixed RCT evidence regarding their effectiveness upon reducing mortality. In this report, I use power analyses (implemented in R, primarily via monte carlo simulations) to demonstrate that previous RCTs have been hugely underpowered to detect these effects. I therefore preferentially utilize other forms of evidence (including RCT evidence of the effect of mass media upon behavior change more broadly, which they are better powered to detect, and evidence from other forms of media such as advertising) to examine the likely effectiveness of mass media campaigns upon health and wellbeing. My BOTECs indicate that mass media campaigns can be extremely cost-effective (i.e. I estimate 26x GiveDirectly for a family planning campaign from Family Empowerment Media, and 31x GiveDirectly for a hypothetical initiative to reduce intimate partner violence). These values reflect that (1) mass media campaigns can be highly cost-effective at even a very small effect size, given the number of people that they can reach, and (2) information (unlike say, a bednet) does not necessarily disintegrate with time and can be freely passed onto others, and in small percentage of cases may contribute to broader changes in social norms. Overall, I think that mass media campaigns present a highly promising—albeit risky, relative to other global health interventions—area for philanthropic investment. I argue that (in many cases) this is a philanthropic investment worth taking. In particular, I highlight the potential role of mass media in correcting misconceptions about modern contraception, and in combating intimate partner violence.
Summary
The promise of mass media lies in its ability to reach a large audience, and to shift behavior by improving knowledge, awareness, and social attitudes towards particular areas of health. Mass media campaigns can operate via media including radio, TV, newspapers and the internet. They have been used for various purposes in high-income contexts, including reducing the use of tobacco and alcohol, promoting healthy eating, and increasing rates of cancer screening.
Estimating the potential impact of a mass media intervention involves estimating the degree to which a given media campaign changes behavior, as well as the causal effect of that behavior change upon relevant health metrics such as mortality. In this investigation, I show that (given the scale at which mass media campaigns operate) these campaigns can be cost-effective even if the expected changes upon behavior, and the corresponding effects of that behavior change upon mortality, are relatively small. I then examine the expected effect of mass media interventions upon behavior and health, primarily using existing evidence from randomized controlled trials (RCTs) in low and middle income countries (LMICs).
My power analyses find that existing RCTs are hugely underpowered to directly find effects of mass media upon mortality (including at effect sizes that my BOTEC suggests would be highly cost-effective)—although many RCTs have successfully found differences in rates of health-relevant behaviors, such as rates of clinic visits or use of modern contraception, which they are better-powered to detect. My BOTECs therefore uses this evidence (alongside evidence from other sources about how changes in these behaviors affect mortality) to assess the impact of mass media interventions.
Overall, my BOTECs suggest that these interventions are cost effective (4x - 31x GiveDirectly), despite including large discounts (~40%) for generalisability. In addition, I also argue that there is reason to expect that a small number of mass media campaigns may generate long lasting impact, as knowledge (unlike say, a bednet) does not always disintegrate over time and can be freely passed onto others—a factor that has generally not been accounted for in existing work. There may be a small number of campaigns that shift entire group attitudes and behaviors (for example, contributing towards a change in social norms about contraception, or about the social acceptability of intimate partner violence) that are hugely impactful.
Areas where mass media campaigns may be especially helpful include those where current misconceptions about health exist, or where unhelpful social norms persist. Given that current work suggests that the impact of mass media is very variable, I suggest that researchers attend to several features that have been linked to campaign effectiveness. These include cultural relevance, tackling misinformation or lack of information, and using these campaigns in areas where people have the resources to take the appropriate action. Examining these features with regards to particular campaigns may help grantmakers avoid donating funds to campaigns that are likely to have no impact.
Overall, I think that mass media campaigns are a promising—albeit risky—area for philanthropic donations. I am unsure about whether mass media campaigns are significantly underfunded in high income contexts, but it seems likely that they are underfunded in LMIC. Note that it is cheaper to run mass media campaigns in LMIC, where radio airtime is cheap, and there is some evidence that these interventions are especially effective in LMIC: experimental effect sizes have generally been larger in LMIC relative to HIC, despite stronger research designs in LMIC. I suspect that this is because there is ‘low-hanging fruit’ for mass media interventions in LMIC, in the sense that there are critical gaps in healthcare knowledge for many people in LMIC, who do not necessarily have easy access to high-quality healthcare information.[1] I identify several charities operating in this area, but I do not have a strong sense of their existing RFF; I recommend proceeding to investigate these charities.
Background: the potential of mass media
Mass media interventions work by exposing a large number of people to content, in the hope that they change their behavior. In high income countries, public health campaigns are routinely used by governments and health services—examples include COVID campaigns, the British THINK! Campaign against drink driving, and Australia’s Slip! Slap! Slop! campaign to improve sun safety behaviors. Some charities (such as Family Empowerment Media (FEM) and Development Media International (DMI)) conduct similar mass media campaigns in LMIC, with the hope of increasing rates of health-promoting behaviors—such as using modern contraceptives, or attaining treatment for children who show symptoms that are suggestive of diseases such as pneumonia.
Before examining the evidence in more detail, it is useful to lay out how we can understand the effectiveness of a mass media campaign. The potential of mass media comes from its ability to reach a huge number of people at once, relatively cheaply—especially in low and middle-income countries (LMIC) where radio airtime is inexpensive. This means that even if a very small percentage of people change their behavior (in a way that reduces mortality or morbidity), the intervention can be extremely effective.
To illustrate this mathematically, we can roughly model the effectiveness of a mass media intervention (that works by reducing mortality in young children) as follows;
Where N is the number of people reached by the intervention, B is the average change in behavior due to this intervention (across the people reached), E is the effect per unit of behavior change upon mortality, and C is the cost of the intervention.
We compare both life-improving and life-saving interventions in terms of cost-effectiveness. In order for a lifesaving intervention to be more cost-effective, we (Founders Pledge) estimate that it must be able to save 1 under-5 life for $38,571. This is because our current bar for recommendation is to be more cost-effective than GiveDirectly: we estimate that GiveDirectly can double someone’s income for an approximate cost of $299, and we value ‘saving an under-5 life’ at 129 ‘income-doubling equivalents’ (new moral weights report forthcoming). Note that this is our lowest bar for recommendation, and we believe that there are much more cost-effective ways to save lives (e.g. the Malaria Consortium for ~$3408). This means that the following equation should be true to meet our cost-effectiveness bar;
Data from Development Media International (DMI), who conduct mass media campaigns in LMIC, indicates that one of their trials reached 480,000 individuals (who stand to benefit from the intervention), at a cost of $3381381 in their first year. This is the start up costs plus the average annual operational costs (Kasteng et al. 2018; see pg. 5).[2] This is a cost of approximately $7 per intervention-relevant person (here, the parent of an under-5 YO or pregnant woman). Note that their cost per person is significantly lower when RCT costs are excluded (around £0.11; personal communication), but I use this $7 estimate to be highly conservative. So if we take DMI’s reach and cost to be typical for a similar program, this equation should be true in order for that program to meet our cost-effectiveness bar;
This means that a behavior-change intervention is cost-effective if;
The expected change upon behavior (as well as the effect of behavior change on mortality) can be very small and yet still result in a cost-effective intervention—this is a result of the ability to reach a large number of people cheaply. So if the effect of the intervention upon behavior is 2% (for example, 2% of the population would newly recognise a symptom of pneumonia in their child and take them to the clinic), and the average effect of that change in behavior is to reduce mortality by 1% (resulting in 1% decrease of mortality, for people who changed their behavior), then this would likely meet our cost-effectiveness bar.
A lot of the controversy around mass media interventions has come from establishing whether or not they can change behavior (B). For example, GiveWell’s evaluation of DMI concludes ‘our view is that we do not plan to prioritize additional consideration of this program, given the limitations of the evidence of the effect of DMI’s media campaigns on child mortality (Development Media International - July 2021 Version | GiveWell, n.d.).’ In the following sections, I will examine whether we should predict that these mass media interventions will change behavior (and the degree to which they may do so). I focus primarily upon randomized control trials of similar interventions in LMIC contexts, but given the limitations of this data (see below) I also pull from evidence of other contexts where media aims to shift behavior—such as advertising, and public health campaigns in HIC contexts. I then examine the link between behavior change and effects on mortality (E), as well as whether there is likely to be room for funding for organizations conducting these interventions.
Can mass media successfully change behavior?
The strongest (and most direct) evidence about mass media change from campaigns in LMIC contexts comes from a series of randomized controlled trials in Burkina Faso and other areas. Unfortunately, it is difficult—but not impossible—to experimentally test mass media in this way.
Problem 1: It is difficult to randomize mass media exposure
It is very difficult to control (on an individual level) someone’s exposure to a mass media campaign.[4] Consequently, many tests of mass media campaigns use cluster randomization, often at the level of the community or region. In this design, different groups (or ‘clusters’) are randomized to receive the intervention of not (e.g. Banerjee et al., 2019; Croker et al., 2012; Sarrassat et al., 2018). Clusters undergo pre-test and post-test measures of the behavior in question, to observe if there is more change within the test clusters. However, while cluster RCTs are methodologically strong, they also present several drawbacks. First, there are relatively few places where it is possible to run them; cluster RCTs are facilitated if researchers can locate distinct clusters that are broadly similar (to minimize selection effects), yet can be differentially assigned to test versus control groups with minimal spillover. Researchers have attempted to run these trials in Burkina Faso, a region where it is possible to gain a large number of clusters due to the unique set up of local radio (e.g. Glennerster et al., 2021; Sarrassat et al., 2018). Since there are many local languages spoken, it is possible to minimise spillover effects by running campaigns on particular radio shows (and therefore in particular languages) only. However, it is common for these local regions to vary by various socio-demographic characteristics, such as socioeconomic status and tribal group—issues that can be somewhat mitigated by statistically adjusting for these confounds, but leave open the possibility of confounds (e.g. see Sarrassat et al. 2018, who suffered this issue).
Note that Family Empowerment Media (FEM) are currently developing and testing a new transmitter that can selectively transmit radio programs to different groups of people; this may help to solve the problem of effective randomisation to media exposure. FEM hope to publish a paper on their transmitter early next year.
Secondly, cluster RCTs (relative to individual RCTs) have lower statistical power. This is because in a cluster trial, the responses of individuals within each cluster are often correlated with other variables (such as socioeconomic factors, environmental factors etc)—many of which may be unspecified. This leads to an increase of within-cluster correlation and between-cluster variability in terms of the specific health outcomes that are being measured. Failing to account for the effects of clustering in analysis results in inflated type 1 error rates, or false positives (Dron et al., 2021; Golzarri-Arroyo et al., 2020; Parallel Group- or Cluster-Randomized Trials (GRTs) | Research Methods Resources, n.d.). Note that the power of a cluster trial depends to a large extent on the number of clusters, not just cluster sizes—I believe this is a key (and perhaps unavoidable) problem in the Sarassat et al. (2018) trial, which GiveWell’s analysis of DMI critically relies upon (see the section about this trial).
Another RCT research design is to expose randomly-selected individuals to mass media in a controlled fashion. For example, Banerjee et al. (2019) selected individuals to watch screenings of the show MTVShuga, which educates people about HIV risk behaviors. This has the advantage of a cleaner research design, but it is very unclear whether results from this work are generalisable to mass media contexts. In the real world, there is an extremely crowded media environment: it seems very possible that people who do exhibit behavior change in response to watching MTVShuga in a controlled environment might not take the time to watch the show outside of the study context.
Finally, there appears to be different norms about study design across research fields. Campaign research (a field within the broader topic of public health, that generally focuses upon public health campaigns in high income contexts) often uses pretest-posttest control group designs: one group is exposed to the campaign and one is not, with baseline tests of the behavior in question prior to exposure to media, and posttest tests after exposure (Noar, 2009). This is clearly stronger than research designs that do not include a pretest (which are also common in this field; Meyer et al., 2003, Mohammed, 2001) and are therefore vulnerable to maturation effects, or designs that do not even include a control group and are therefore vulnerable to maturation and selection effects (which are also common, see Noar, 2009). However, pretest-posttest control group designs are nevertheless vulnerable to regression to the mean—namely, if the two groups are nonequivalent with regards to the behavior in question at baseline. They are also vulnerable to selection effects, since the group that is assigned to each condition is not usually random, but instead a sample of convenience (Hornik et al., 2002; Noar, 2009).
Other research designs that test the effectiveness of mass media include instrumental variables analysis, but there are a limited number of studies of this type. Presumably, this is because researchers have struggled to identify suitable instrumental variables that systematically vary with mass media exposure, yet are unlikely to have a causal relationship with mass media exposure—but an interesting exception comes from a study that uses Rwanda’s topography (since certain topographies block radio signals) to estimate the effect of radio campaigns upon participitation in the Rwandan genocide (Yanagizawa-Drott, 2014). Regression discontinuity designs have also occasionally been used, but primarily in high income contexts. For example, Spenkuch and Toniatti (2018) took advantage of advertising differences resulting from FCC regulations to find an effect of advertising upon US political candidate vote shares.
Problem 2: Small effect sizes
The nature of mass media effects makes them difficult to experimentally examine; these effects are expected to be very small, but the power of mass media comes from its potential to expose a huge number of people at once. To the best of my understanding, many existing RCTs are hugely underpowered. For example, Sarassat et al. (2018)—the largest RCT in an LMIC, and the paper which GiveWell’s analysis of DMI primarily focuses upon—is only powered to detect a 20% reduction in their outcome (all-cause post-neonatal under-5 child mortality), but it seems extremely over-optimistic to assume such a high effect size. Note that the ‘benchmark’ expectation for behavior change in high-income countries (in response to a mass media campaigns, see this section) is around 5%, and my BOTEC in the ‘Background’ section suggests that even effects of significantly less than <5% may still be cost-effective. I cover this study in greater detail below.
Problem 3: Few attempts to measure long-lasting change
The existing RCTs measure behavioral effects upon people who have been directly exposed to a given media campaign, usually during that campaign’s duration. However, mass media interventions work by informing people—importantly, knowledge (unlike say, a bednet) does not necessarily disintegrate over time. For example, if a person learns to recognise the symptoms of malaria in their child and takes them to a clinic, they are presumably more likely to repeat this behavior if they see that symptom again in a few years time. They could also potentially spread this information to other people.
It is extremely unclear how long knowledge gained from mass media interventions continues to exert an effect upon behavior: to the best of my understanding, we should expect these effects to be very right-skewed, with a very small number of interventions exhibiting very long-lasting effects. That is, most campaigns may shift behavior solely during the duration of the campaign—for example, because the campaign works by reminding people to do things that they would otherwise forget to do. At the other end of the spectrum, behavioral effects could shift behavior throughout a person’s lifetime (for example, because they learn that the behavior in question achieves their desired outcome, such as improving their children’s health), or could even contribute towards shifting group norms about typical ways to behave. In the latter case, these interventions could be hugely impactful, since they would affect many more people beyond those who are directly exposed to the campaign. An example of a shifting social norm in a HIC context is that of ‘considerate smoking behavior’; over time, it has become less socially acceptable to expose others to one’s own cigarette smoke (Nyborg & Rege, 2003). Beyond smoking, social norms are also thought to be important in understanding other behaviors that impact upon health, such as violence against women (García-Moreno et al., 2015), and adolescent contraceptive use (Sedlander & Rimal, 2019).
Are social norms malleable to change via mass media? At present, this is an open question. There is evidence suggesting that social mechanisms of information spread are important; Arias (2019) contrasted the effect of an anti-violence radio campaign that was disseminated either privately (to individuals via CD) or publicly (via radio), relative to a baseline condition in which people did not hear the campaign (the local topography prevented the transmission of this program over the radio). He found that people were less likely to report that violence against women is socially acceptable following the campaign, but only in the public condition—one interpretation of this evidence is that social norms are based in part upon an understanding of what others are likely to think, given the information that they have been exposed to.
Vogt et al. (2016) used insights from work that models the spread of social norms, in an attempt to design a mass media intervention that could shift social norms about female genital mutilation (FGM). In particular, they chose an area where there was existing difference of opinion with regards to FGM. Note that work from cultural evolution has suggested that social norms are more liable to shift in areas where there is strong disagreement; given the role of conformist transmission (where people tend to copy the behaviors and opinions of the majority in their social group), it may be especially powerful to target communities where it is possible to ‘tip’ the majority of opinion towards one direction (Muthukrishna, 2020). I view this work as being in its infancy—for example, I’m not yet aware of any experiments which have tested participants over a significant length of time, or found an impact upon behavior—but Vogt et al. (2016) were successful in designing a movie that appeared to improve attitudes towards women who do not undergo FGM. It is unclear whether this was successful in actually shifting an entrenched social norm, but I view this as an area that may be promising if there are further research breakthroughs.
Taken together, I think that CEAs examining mass media intervention should include some estimate of potential long-term effects—but I think these effects should be weighted with a low probability. To give an example of this approach, my BOTEC for FEM[5] considered three scenarios. In the most likely scenario (70% probability), the benefits do not persist after the campaign ends. In the medium scenario (25% probability) the results last for 4 years in total, with strongly diminishing effects per year (60% discount rate per year). In the best case scenario (5% probability) the effects shift a social norm, so it becomes common knowledge in Kano that contraceptives are freely available and do not have significant health risks. In this scenario, I apply a low discount rate (8% per year) and assume that the benefits last 8 years—after 8 years, I assume that contraceptive access and knowledge would have come to Kano without FEM’s intervention. This is not meant to be a conclusive way to model potential long-term benefits (this is currently very rough and relies upon my own subjective estimates), but an illustrative exercise—this places FEM’s cost-effectiveness at ~27X GD.[6]
Existing evidence from RCTs in LMICs
There are a relatively small number of RCTs examining mass media effects in LMICs. These studies are also outlined in excel here, where I checked bias systematically using Cochrane criteria (but the important information is all outlined below).
Sarassat et al. 2018 (Burkina Faso, childhood survival with DMI)
This is a cluster-randomised trial, testing the impact of a mass radio campaign on family behaviors and child survival in Burkina Faso (note that there are two Burkina Faso mass media RCTs, which examine different mass media topics; the family planning RCT is listed separately below). The results of this trial were written up in GiveWell’s evaluation of Development Media International. This is the most ambitious RCT testing mass media in an LMIC that I am aware of, and the key result is that while the trial did not find an impact upon child mortality (it was under-powered to do so: I estimate below that their power to detect a 5% effect upon mortality was between 0.027 - 0.1), it did find an impact upon behaviors that have been linked to improved child mortality—including child consultations, attendance at antenatal care appointments, and deliveries in health facilities. There are a number of limitations to this study (see limitations below), making it challenging to interpret. I analyse this study in more detail (relative to the other RCTs), partly because of the difficulty in interpreting it, and secondly since my understanding is that the results of this trial are a key component of GiveWell’s decision not to pursue mass media interventions further: note that the endline results of this experiment were not available to GiveWell at the time of their evaluation (Development Media International - July 2021 Version | GiveWell, n.d.).
RCT design
The intervention ran from March 2012 to January 2015, and took advantage of Burkina Faso’s radio topography (namely, that there are a number of different local radio stations operating in different languages) to run a cluster-randomised RCT. The radio campaign focused on getting parents to recognise signs of pneumonia, malaria and severe diarrhea in their children—and seeking medical care in response to these symptoms. 7 clusters were randomly assigned to the intervention group, and 7 clusters to the control group. Household surveys were completed at baseline, midline (after 20 months of campaigning) and endline (after 32 months of campaigning), to assess under-5 mortality. Routine data from health facilities were also analysed for evidence of changes in use (namely, increases in consultations).
Results
Under-5 child mortality decreased from 93.3 to 58.5 per 1,000 livebirths in the control group, and from 125.1 to 85.1 in the intervention group; there was no evidence of an intervention effect (risk ratio 1:00, 95%CI 0.82-1.22). This is from the survey data.
In the first year of the intervention, under-5 consultations increased from 68,681 to 83,022 in the control group, and from 79,852 to 111,758 in the intervention group (using time-series analysis: 35% intervention effect, 95%CI 20-51, P<0.0001). New antenatal care attendances decreased from 13,129 to 12,997 in the control group, and increased from 19,658 to 20,202 in the intervention group in the first year (intervention effect 6%, 95% CI 2-10, p=0.004). Deliveries in health facilities decreased from 10,598 to 10,533 in the control group, and increased from 12,155 to 12,902 in the intervention group in the first year (intervention effect 7%, 95% CI 2-11, p = 0.004). This is from the routine data from health facilities.
Note that the researchers also used self-reported health behavior; I have not used this in my analysis, as I weight the government data more than self-report due to the possibility of social desirability bias.
Limitations
Reasons that the study results may underestimate the effectiveness of mass media campaigns:
- Power. The power of a study is the probability that it will successfully reject the null hypothesis (here, that the mortality rate is similar across control and test groups), when the null hypothesis is false. An underpowered study is therefore liable to make a Type 2 error, where it fails to detect a real effect in the data. Sarassat et al. (2018) write that their trial may have been underpowered;
‘The small number of clusters available for randomisation together with the substantial between-cluster heterogeneity at baseline, and rapidly decreasing mortality, limited the power of the study to detect modest changes in behavior or mortality.’
To test this empirically, I ran a power analysis using cpa.binary (from the ClusterPower package in R) using their data. To account for changing rates of mortality, I used the rate of child mortality in the control group at the end of the trial as the baseline mortality. I then estimated the trial’s statistical power to detect a 5% change in mortality, because I think that 5% is a realistic—if still quite optimistic—prediction. For example, a 5% change in mortality could occur if the proportion of parents seeking medical care in response to their child’s symptoms increased by 50%, and in 10% of those cases this saved the child’s life. Note that values well below a 5% decrease in mortality are still likely to be cost-effective (see Background section above). Overall, my analyses found that the study’s power to detect an effect of 5% upon childhood mortality was only 0.027; it is highly unlikely that the study would have successfully identified this effect.
To double check this result, I also estimated power via Monte Carlo simulations (using cps.binary from the same package) and found that the power was approximately 0.1; meaning that there was only a 10% chance that the study would successfully identify a positive result upon mortality. There are several factors contributing to this lack of power, meaning that changing one factor (such as the effect size) does not dramatically change the power.
Why is the power of this study so low? First, there is a very limited number of clusters (14). Note that the power analysis that is within Sarrassat et al. (2018) and referenced in their pre-registration was used to determine overall sample size, but not the number of clusters. On the one hand, this is understandable since they were presumably unable to achieve a higher number of clusters; they were limited by the number of different radio stations they could work with, who operated in different local languages. However, the problem is that this is a key bottleneck; Dron et al. (2021) write ‘a hypothetical cluster trial with hundreds of thousands of participants but only a few clusters might never reach 80% statistical power, owing to the diminishing returns that increasing participant numbers relative to increasing cluster numbers provides for cluster trials’. In my power analyses, increasing sample size further does not increase power—but increasing cluster numbers does.
Second, they were only powered (at 80%) to detect a 20% change in under-5 mortality—but it seems very optimistic to assume a 20% change in mortality. In a write-up from DMI about the trial on the EA forum, the CEO of DMI writes;
‘...there was no way of powering any study in the world better than this. With unlimited funds we could not have devised a design that would detect mortality reductions of less than 15%. It was impossible. There’s no country in the world where you could do that’.
Note that meta-analyses of mass media effects use a benchmark of around 5% change in behavior (see this section). Here, this would mean a 5% increase in health-seeking behaviors such as getting consultations in response to child illness, and a smaller effect upon mortality.
Third, child mortality decreased (across both control and intervention areas) through the trial—effectively reducing power even further. In addition, there was substantial between-cluster heterogeneity at baseline: accounting for the analysis methods used to attempt to adjust for this means the study effectively had even less power.
Given the low power, I do not take the child mortality results very seriously; I think it is very likely that they were underpowered to capture an effect upon child mortality.
- Results could last beyond trial end-point. Using the data about health consultations (Kasteng et al. 2018), there were increases in under-5 consultations (35%; p <0.0001), antenatal care attendances (6%, p = 0.004), and deliveries (7%, p = 0.004) in the intervention arm relative to the control arm, in the first year. In the second and third years, the estimated effect on antenatal care and deliveries remained relatively constant, but without statistical evidence for a difference between intervention and control group for antenatal care specifically. The effect on under-5 consultations seemed to decrease over time, but evidence of an intervention effect remained (year 2: 20%, p = 0.003, year 3: 16%, p = 0.049). The degree to which these effects persist is very unclear—my assumption is that they would slowly decrease year by year, rather than drop off a cliff after year 3. In my BOTEC, I average out the results across the three years and (similarly to my approach for FEM) weight three potential scenarios for the potential of long-term effects via my estimate of the probability of this scenario.
Reasons that the study may overstate the effectiveness of mass media campaigns
- Imbalances between control and intervention arms. The control group was ‘better off’ than the treatment group at baseline by several metrics, including child mortality rates, distance and access to health facilities, and the proportion of women giving birth in a health facility.
It is difficult to know the direction in which this would bias the result, but overall I think it is more likely to contribute towards overstating the study result. Assuming regression to the mean, this would suggest that the trial results may overstate the intervention impact—that is, some of the effects that were attributed to the intervention in the worse-off intervention group were actually the result of regression to the mean. But there is also reason to expect that changes to health-seeking behaviors would be strongest among people living closer to a healthcare facility (and indeed, this opinion is supported by the results that the intervention resulted in increased care seeking among families that were closer to a healthcare facility).
DMI ran a cluster-level adjustment for baseline levels in the analysis to adjust for these imbalances between control and intervention arms. Nonetheless, I think this is a reason to discount the study results somewhat; my BOTEC includes a discount of 30% to account for this and potential problems of generalizability/ p-hacking.
- Limits to generalizability. The primary purpose of this trial, as I understand it, was to test whether mass media could influence health behavior rather than to provide a test of whether similar initiatives will work. They therefore used a ‘saturation+’ approach that involved broadcasting ten times a day—other initiatives may be unable to have this kind of coverage. In addition, DMI did select radio stations partially on the basis of which ones allowed for promising opportunities to establish effective working partnerships; future work may be unable to find radio stations that would work as cheaply and provide as dense a coverage.
- Potential for systematic differences in the children who (as a result of the intervention) are taken to the clinic, relative to other children showing symptoms of illness. I think it is quite plausible that the children who are ‘newly’ taken to the clinic (i.e. as a result of the intervention, and would not have been taken to the clinic otherwise) are less likely to get severely ill than children showing these symptoms in general. That is, perhaps the children who are at higher risk of death would always have been taken to the clinic regardless, and the children who are ‘newly’ taken to the clinic are the borderline cases who are less likely to become severely ill. I included a discount of 20% in my BOTEC to account for this (when estimating the number of deaths averted as a result of the intervention).
- Potential for p-hacking. The project collected a number of different outcome measures, and the administrative data (where there was evidence for an intervention effect) is not the primary outcome.
- Blinding. The surveyors (who collected the data about child mortality) were not blinded, which could have biased the results. However, no intervention effect was observed for child mortality anyway.
Problems which seem likely to have no effect, or it’s unclear which direction the effect would go
- Contamination in the control group. Due to a radio station that neighbored a control group area using an illegally high radio strength, there was some contamination in one of the control group clusters. Given that these results were not excluded, this could mean that the effectiveness of the campaign is underestimated; however the researchers state that excluding the data has little effect.
Strengths
- This study worked in six different languages, with coverage of 63 different villages and around 480,000 under 5-YOs (Kasteng et al., 2018). It is the only RCT testing mass media of this size in an LMIC.
- The study is pre-registered here, and the authors state their intention to use government clinic data as a secondary outcome (the survey data about child mortality is the first outcome).
- There was no increase in consultations for illnesses that were not covered by the campaign (e.g. upper respiratory diseases) (Roy Head, n.d.; Kasteng et al. 2018). This suggests that the intervention was resulting in increased consultations specifically for the diseases that they campaigned about—rather than increasing numbers of consultations more generally.
- I think that it is likely that seeking treatment for pneumonia/ diarrhoea/ malaria improves health outcomes. For example, treatment of cerebral malaria (a severe form of malaria) with artemisinin is associated with a 78% reduction in in-hospital mortality (Conroy et al., 2021). Treatment of diarrhoea with oral rehydration salts has been associated with a reduction in mortality of ~93% (Munos et al., 2010). Note that I still discount these estimates in my BOTEC (because I am unsure about the quality of care may be lower in Burkina Faso); for example, I assume that attaining treatment for malaria (relative to no treatment) decreases the risk of death (0.01%, using prevalence and death data for under 5 year olds in Burkina Faso, from the global burden of disease) by 70%.
Research paper authors’ interpretation:
In the original paper, the authors state that there is no detectable effect on child mortality, and that there were substantial decreases in child mortality observed in both groups over the intervention period–reducing their ability to detect an effect. However, they also state that they did find evidence that mass media can change health-seeking behaviors.
In a separate paper, many of the same authors then used the Lives Saved Tool (LiST) to estimate the impact of those health-seeking behaviors (the increases in antenatal care appointments, and under-5 consultations) upon child mortality (Kasteng et al., 2018).[7]
My interpretation:
I think it is important to analyse this study within the context of the difficulty of testing a mass-media campaign via RCT on this scale. At the same time, I also think that there are significant limitations to this study. It is difficult to assess whether these limitations mean that the effect of the study is likely under or over-estimated.
Critically, I believe the study is hugely under-powered to establish an effect upon childhood mortality. For these reasons, I think it is fair to use the data about health-seeking behaviors (rather than rely solely upon the mortality data). However, there are also a few reasons to expect that the health-seeking data may overstate the potential effect of mass media interventions upon health if similar initiatives were scaled up in different areas. This study was able to achieve a very high level of coverage; similar interventions may struggle to achieve this. In addition, there is an imbalance between the intervention and control arms at baseline; I think it is quite possible that this therefore overstates the impact of the intervention if there is regression to the mean within this group. Finally, the studied population listens to the radio, is rural and yet is close to health clinics; these characteristics may not be true of other populations that could run similar initiatives. For these reasons, I include a discount of 30% in my BOTEC (and an additional 20% discount for my estimate of deaths averted).
On the other hand, there are also reasons to expect that this study may underestimate the effect of mass media interventions in general. First, the intervention location was selected primarily because it was possible to institute an RCT here; authors such as Deane (2018) have stressed that regions should be selected because there is an opportunity to correct misinformation/ provide lacking information. In these areas, people’s behavior may be more amenable to change. Second, there is (understandably) no data beyond 32 months after the intervention. Interestingly, there appears to still be an effect at this endline—if there exists an effect beyond this point, the overall effect of this intervention could be large.
With regard to the LiST modelling, I do not have a strong sense of whether LiST models tend to understate, overstate or provide a realistic impact of a program’s effectiveness—although I note that this is a well-established tool. I therefore produced my own BOTEC that draws from the data from the RCT to estimate how health-seeking behaviors predict mortality, rather than relying upon LiST.
For all these reasons, I included heavy discounts within my BOTECs for generalizability, and I used separate data sources from LiST to assess how the extra clinic attendances were likely to have affected mortality. My own results are somewhat less cost-effective than LiST modeling suggests (around $250 per DALY, relative to their estimate of $99 per DALY). However, note that I used the costings from the RCT trial (rather than the per person cost in a standard context, where an RCT was not being run)- this likely made the intervention appear less cost-effective in my BOTEC. A key question is to establish the cost-effectiveness of this program for scale-up, i.e. when an RCT is not beign completed at the same time.
Glennerster et al. 2021 (Family Planning, Burkina Faso, DMI)
This is a two-level randomized control trial covering 5 million people, testing (1) exposure to mass media (with 1500 women receiving radios) and (2) the impact of a 2.5 year family planning mass media campaign (cluster trial; 8 of 16 local radios received the campaign). This trial was also completed through DMI, and is more recent than the Sarassat et al. (2018) trial. It was not available at the time of GiveWell’s analysis of DMI.
Design
1550 women (who had no radio originally) were randomly selected to receive a radio. Half of these women were within broadcast range of radio stations randomly selected to receive an intensive family planning radio campaign, and half were in regions not covered by the campaign.
Two waves of survey data were used, from 7500 women (both those with and without radios at baseline), as well as monthly administrative data on the number of contraceptives distributed by all clinics in the study areas.
Results
Modern contraceptive use rose by 5.8 percentage points (p=0.030) in women who received a radio in campaign areas, compared to women who did not receive a radio in campaign areas. In campaign areas, family planning consultations were 32% higher, injectables 10% higher, and 22% more pills were distributed. Women aged 29-40 were 10% less likely to give birth in the year before the endline in campaign areas, although this was not the primary outcome (my understanding is that the authors believed they would be underpowered to capture this result, so did not include it on pre-registration).
Interestingly, women who received a radio in noncampaign areas reduced contraception use by 5.2 percentage points (p=0.039) and showed evidence for more conservative gender attitudes, compared to women who did not receive a radio in noncampaign areas (see Fig 1). In general, exposure to mass media lowered contraceptive use.
Fig 1: impact of radio campaign on modern contraception prevalence rate (Glennerster et al. 2021)
Limitations
- Generalisability: the survey results come from rural areas, without electricity access (so however people use a TV and more people rely upon radio), and where there is high contraceptive availability—an area that the authors estimate is only 7% of the country. So results may only be generalizable to a relatively small number of areas. For this reason, I discounted by 50% in my BOTEC. I am unsure of this value, and would spend more time here in a longer investigation.
- Blinding: the surveyors were not blinded to treatment condition, which could have impacted the results.
Strengths
- This is a large study that involved surveying 7,500 women (and broadcasting to around 5 million people in total). This study is sufficiently well-powered to capture changes in contraceptive use, the primary outcome of this study. Note that for this study, the outcome metric produced large changes in behavior (around 20%)—even if that behavior is less closely tied to survival, relative to the outcomes of the Sarasset et al. (2018) study.
- This study is pre-registered, and follows the pre-registration plan.
- As well as the data about health behavior (rates of contraceptive use), survey data also indicated improved knowledge about contraceptive methods, attitudes and towards family planning, and self-assessed health and well being in intervention relative to control areas. This data aligns with the primary findings of the study.
Interpretation
I think this study provides the strongest available evidence for an effect of behavior resulting from media exposure, in an LMIC context. Women who received a radio increased their contraceptive use by 5.8 percentage points in campaign areas, relative to women who did not receive a radio.
Banerjee et al. 2019 (HIV, Nigeria)
This study used an ‘edutainment’ TV series called MTV Shuga, aimed at providing information and changing attitudes and behaviors related to HIV/ AIDS. Unlike the two RCTs above, the participants were exposed to the show in an artificial experimental setting.
Design
Researchers visited a random selection of households, and then randomly selected one 18-25 year old in each household to be invited to an initial film screening (the film was different from MTV Shuga). The baseline survey took place at this point. Participants were randomised to treatment or control groups.
Control: participants watched a placebo TV series.
Treatment 1: Participants were shown MTV Shuga.
Treatment 2: Same as treatment 1, except that after the Shuga episodes, subjects were also shown video clips containing information on the beliefs and values of peers in other communities who watched Shuga.
Treatment 3: Half of T1 and T2 were offered the option of bringing two friends to the screenings. So T1 and T2 are randomised at cluster level, and T3 cross-cuts across T1 and T2 and is randomised at the individual level.
Results
There were not many differences in baseline characteristics between T1, T2, and T3, but there were in comparing the treatment groups as a whole to the control group. People in the treatment group were 3.1 percentage points more likely to test for HIV than those in the control group. Beliefs about the acceptability of concurrent sexual relationships decreased during the treatment period in the test group, although MTV Shuga did not induce greater condom use. However, the likelihood of testing positive for Chlamydia was 55% lower for women in the intervention groups (the impact on men was in the same direction but statistically insignificant).
Interpretation
MTV Shuga worked in that it improved HIV testing rates and potentially decreased rates of Chlamydia. I view this as further evidence that mass media can successfully shift health-seeking behaviors, but I think that the generalisability of this study is very limited in the context of charitable interventions (since most charity work operates via radio/TV shows, where there are competing demands on the viewer’s attention). I also note the large number of different outcomes they measured, and that they did not see consistent effects across all outcomes; the researchers did not use a bonferroni correction (or other method to account for the number of outcomes measured).
Evidence from meta-analyses of public health campaigns
I also looked at evidence from meta analyses of public health campaigns, for which there is a larger body of evidence. There are relatively few RCTs of public health campaigns (with the exception of those listed above, in an LMIC context)—Head et al. argue that this is due to the difficulties in selectively exposing people to mass media (without spillover), at a large scale (2015). Consequently, I note that I think these meta-analyses are not of an especially strong quality: they pull from papers which use weaker designs.
Snyder et al. (2004) is especially well-cited, and includes data from 48 campaigns in the USA. Overall, they find that on average 5% of the population reached by the intervention change their behavior (for campaigns where the behavior is not enforced, for example by legal consequences)—a value that (in conjunction with other work from Anker et al, 2016) has established the conventional use of a 5% behavior change benchmark, for public health researchers looking to work out adequate sample size in power analyses (Anker et al., 2016). However, I note that this paper does not attempt to test for or adjust for publication biases, and includes data from designs that are not low quality (e.g. pretest/ posttest without a control group). I therefore suspect that 5% is an overestimate, although I note that the RCTs in LMICs found similarly sized (or even larger) effects—my suspicion is that this is an overestimate for high-income countries specifically, where the media environment is more saturated already.
Anker et al. (2016) conducted a meta-analysis of 63 mass communication health campaigns across countries (but mainly focused in high-income countries). A strength of this study is that it had stricter inclusion criteria relative to Snyder et al. (2004); in particular, the authors only included studies with a nonequivalent groups pre-test/post-test design with a control group. That is, designs where campaigns are implemented in one or more different communities, while a demographically similar community serves as a control group. These designs are weaker than cluster randomised control trials, because the groups are not randomised with respect to who receives the mass media intervention—but they do at least use a control group. To be included, campaigns had to employ at least one of the following communication channels: television/ cinema, radio, billboard, magazine, newspaper and/ or advertisements on public transport. The campaigns also had to include a planned message designed for brief delivery (so long form entertainment-education programs were excluded), and campaigns that took place in a controlled setting such as a laboratory were excluded. The campaigns were across areas including nutrition, physical activity/ exercise, cardiovascular disease prevention, sexual health, cancer awareness. Campaign communities consistently demonstrated greater behavior change than control communities; r = 0.05 (95% CI 0.033, 0.075), k = 61. This is broadly congruent with the results from Snyder et al. (2004), despite being more selective about which papers they included.
Overall (and despite a weaker research design) these meta-analyses have generally suggested a smaller effect size upon behavior than the results from the RCTs in LMIC contexts (e.g. the effect of the family planning programs upon behavior was around ~20% in the Glennerster et al. trial, compared to a behavior change of around 5% in the meta-analyses listed here). My interpretation of this is that there is ‘low-hanging fruit’ within LMICs, in the sense that there are areas of considerable misinformation/ lack of information (e.g. about the use of contraceptives) that can be relatively easily addressed via mass media campaigns. I cover this in more detail in the section below. In addition, it could be the case that there is less media saturation in areas such as Burkina Faso, where people are less likely to watch TV.
Other evidence
Outside of the context of public health campaigns, I note that there is strong evidence that mass media can alter behavior—for example, via advertising and propaganda. Existing evidence suggests that these advertisements can alter decisions about which items we buy, as well as preferences and beliefs about particular items (e.g. Sethuraman et al., 2011; Kokkinaki & Lunt, 1999). Along similar lines, advertising can also influence voting decisions. For example, Green and Vasudevan (2016) ran an RCT testing the effect of a radio campaign that aimed to persuade voters not to vote for politicians who engaged in vote buying. 60 different radio stations were randomised to be either the test group (which received 60 second ads aimed to persuade voters or this) versus a control group (where there were no ads). They found that exposure to the ads decreased the vote share of these vote-buying parties by between 4 and 7, from around 4-7 percentage points. Another (more sinister) example of mass media influencing behavior comes from propaganda, where emotionally laden media is used to further a particular agenda. In particular, Yanagizawa-Drott (2014) used information about Rwanda’s topography (which affects radio reception) to estimate the effect of radio broadcasts upon participation in violence during the Rwandan genocide; he estimates that approximately 10% of the participation in the violence can be attributed to the effects of the radio. Overall, there is strong evidence that mass media can powerfully change behavior (see also; DellaVigna et al., 2014; Duflo & Saez, 2003; Gerber et al., 2011; La Ferrara, 2016).
Which media campaigns tend to work?
While meta-analyses of public health campaigns have suggested that they work, there is a significant degree of variability in how well different campaigns work (Noar, 2009). There is a broad literature which examines the effectiveness of different mass media campaigns, usually differentiated by public health topic (e.g. drink driving; (Elder et al., 2004), alcohol consumption; (Young et al., 2018), smoking; (Durkin et al., 2012), family planning; (Parry, 2013). Unfortunately, the key aspects of message design and coverage have not been systematically tested against each other. Therefore, my aim in this section is to highlight key features that other authors have identified as being important towards determining the power of a mass media campaign.
Resources to take the necessary actions
Clearly, behavior change cannot be large if the resources to take the necessary actions (such as the presence of local clinics to seek healthcare from, or free contraceptives) are not available. This point has been stressed in commentary surrounding the Sarrassat et al. 2018 trial; ‘demand-side interventions [such as DMI’s trial, which encouraged parents to take their child to a clinic] are not a substitute for health systems strengthening or other supply-side interventions’ (The Legitimacy of Modelling the Impact of an Intervention Based on Important Intermediate Outcomes in a Trial | BMJ Global Health, n.d.). It is vital that the necessary resources are in place (such as contraceptive access or functioning clinics) prior to a mass media intervention.
Tackling misinformation/ lack of information
I note that the three mass media campaigns in LMIC have tackled topics where there is ‘missing information’ for an area where the listeners are highly motivated to improve health. For example, DMI provides information to improve children’s health (to recognise particular symptoms, and take a child to the doctor in response), and FEM provides information about contraceptives (to tackle misinformation about their health risks, and generally to make contraceptives more socially acceptable). In contrast, a campaign that appears to have failed according to RCT results (the UK’s ‘Change4Life’ campaign; Croker et al., 2012) provided information that people may have known about already—such as that there are health benefits to eating 5 fruits or vegetables a day.
I suspect that there is ‘low hanging fruit’ in the context of LMIC, in the sense that there may be particular areas where people are very motivated to act once they are given the correct information. I think this is especially likely in regions where people have poor access to health information (i.e. where people cannot necessarily google WebMD or NHS direct).
Cultural relevance
I think we should be skeptical of any media campaigns that don’t have strong input from local advisors and writers—note that FEM uses local writers, and DMI works with local people to design their campaigns. There are many aspects of local context (e.g. nature of misinformation about contraceptives) that are barriers to behavior change, and would be difficult to ascertain without input from local people. Second, local people are likely best-placed to provide the message, due to their social standing as a trusted source and in-group member. For example, FEM were able to get a local religious leader to talk on one of their radio shows, which they suspect was a highly persuasive part of their campaign.
Social desirability
Many programs that have been very successful have used social desirability to their advantage For instance, one successful example of public health communication comes from the ‘truth’ campaign that was first conducted by the Florida Department of Health (and subsequently by the American Legacy Foundation). The campaign was aimed at youth between the ages of 12 and 17 years, and showed images of youths who were engaged in public demonstrations against the tobacco industry: youth rebelling against the tobacco industry, rather than using tobacco to appear rebellious. A quasi-experimental design taking advantage of variation in exposure to the campaign stemming from the presence or absence of local affiliates of the networks that broadcasted the ads estimated that the campaign accounted for 22% of the observed decline (25.3% to 18%; so the campaign is thought to have been responsible for around 1.6% of the total decline) in the prevalence of youth smoking between 1999 and 2002 (Farrelly et al., 2005). Note that I would still discount this estimate somewhat—due to potential confounds from their method of measuring exposure, and the potential of p-hacking and publication bias. Nonetheless, I think this highlights how appealing to ‘how people want to be perceived’ may be a powerful tool towards behavior change.
Coverage
In order to promote change at the individual or group level, mass media campaigns need to exhibit sufficient coverage that people can recall the campaign’s message. For example, notable mass media interventions failures include two campaigns designed to lower rates of diarrheal disease and increase rates of immunisation that were both carried out in the Democratic Republic of Congo and Lesotho, and Indonesia-West Java, by the HealthCom project. These failures have been blamed in part upon low exposure levels (Hornik et al., 2002). It has been argued that while public health communication has (rightly) focused upon the design of campaigns, they have not adequately concentrated on the challenge of achieving sufficient message exposure (Hornik et al., 2002).
To what degree could changing behavior improve health?
Assuming that mass media can shift behavior, a second component to examine is the degree to which these behavioral changes can improve morbidity and mortality. The Global Burden of Disease estimates the deaths attributed to various behavioral risk factors, see Fig 2. Note that the DALY burden from these behavioral risks is very large; to give a sense of scale, nearly 500 deaths per 10,000 of the global population are attributed to tobacco use.
Fig 2: deaths attributed to behavioral risk factors according to the Global Burden of Disease.
Mass media interventions can target a full range of potential behavioral interventions, with the disclaimer that some behaviors may be more amenable to successful change than others. Assuming that change occurs, the table below provides estimates of the resulting predicted impact in terms of % change upon mortality or DALYs. These reductions are sufficiently large to suggest that mass media interventions which cause behavior change in ~2% of the population will be cost effective.[8]
Behavior to modify | E: per person change in mortality/ DALY after behaviour change | Source |
Tobacco consumption | 5% reduction in mortality | |
Using contraceptives in Kano, Nigero | 1% reduction in mortality (based on avoiding maternal mortality) | |
Getting treatment for malaria when otherwise would not have done | 0.82% reduction in mortality | Mass Media BOTEC: no. of child deaths averted/ number who newly seek out treatment |
Going to antenatal care appointments | 0.98% reduction in infant mortality | Used to model effectiveness of Suvita |
Are mass media interventions underfunded?
Roy Head (the CEA of DMI) has argued that ‘mass media is not seen as a core component of public health’, in part due to the difficulty in proving effectiveness through randomised controlled trials (Head et al., 2015). I am not sure whether public health campaigns are necessarily underfunded in high-income contexts—where these campaigns are expensive to run, compete with multiple other sources of information, and the population already has easy access to high quality information about health. But it seems that mass media campaigns are actually especially well-suited to LMICs, given cheap radio airtime, reliance on fewer sources of information, and the ability to fill in ‘gaps’ about healthcare knowledge. To the best of my understanding, government-run public health campaigns in LMIC are relatively unusual—I could not find many examples online. For these reasons, while I couldn’t find actual data on the amount of money spent on mass media campaigns in LMIC, I suspect that this area is generally underfunded. There is some support from effective altruist organisations (such as The Life you Can Save, who recommend DMI).
As a general point, behavior is a key determinant of health (see Fig 2)—yet behavioral interventions are not generally regarded as a critical component of public health in the way that medicine is. Behavior change can effectively be a preventative measure—i.e. for reducing rates of STDs, for promoting heart-healthy behaviors, or by making it more likely that people will go to the clinic to receive treatment.
I think there are several nonprofits in this space that may have room for funding:
- GiveWell’s evaluation of DMI says that in 2015, DMI estimated that they could productively use approximately $12 million in unrestricted funds to scale up and launch more campaigns to reduce under-5 mortality. I am not aware of DMI’s current funding situation, but I note that they have ongoing work in the areas of early cognitive development, and designing videos that are designed to go viral on WhatsApp.
- Family Empowerment Media broadcast shows about contraceptives in Nigeria. I estimate a cost-effectiveness of around 27X GiveDirectly for their work in Kano. They currently have a funding gap for next year of ~$655K (published with permission from Anna Christina Thorsheim, co-founder/ director of FEM)
- Community Media Trust is a South African non-profit, that focuses on using social media and radio for health benefits. For example, one of their recent projects focused on increasing the uptake of antiretroviral treatment for people who are HIV positive.
- FarmRadio broadcasts resources, information and training to farmers in Africa, with the aim of increasing the ability to grow crops.
- BBC Media Action conducts various projects, such as producing radio shows tackling reproductive health taboos in South Sudan. However they may be well-funded already, as they recieve some funding from the UK government.
Things that are missing from this report
With more time, I would have analysed the effect of time upon media interventions in more detail. One thing I am curious about is whether mass media organisations can simply pivot to use their infrastructure to use different media campaigns year on year: this would then maximise their start up costs, and minimise exposing people (who have already gained the informational benefits from prior broadcasts) to the same information past the point of benefit.[9] More broadly, I think that understanding the long-term effects of mass media campaigns is vastly underexplored—and I suspect that some estimation of these (even if they are, by necessity, very rough) should go into CEAs.
I am also curious about spillover effects, which have not gone into my BOTECs. That is, do people pass the information on to others who have not been exposed to the campaign? I suspect that this is sometimes the case, if at a relatively low frequency.
I think that the possibility of mass media to contribute to positive norm shift is hugely underexplored, and potentially very impactful.
Conclusions
Mass media has huge potential, because (at least in LMIC contexts) it is possible to expose a huge number of people to a campaign very cheaply. This means that even if the effect of these campaigns is very small, it is still likely to be cost-effective—in my opinion, the importance of this factor in determining cost-effectiveness has been underappreciated. In addition, existing work using RCTs in LMICs suggests that the effect size is actually not especially small—for example, the ~35% increase for child consultations of malaria/ pneumonia/ diarrhoea from the Sarassat et al. (2018) trial. Finally, I think that the impact of LMIC mass media interventions is heavily right-skewed (and that the mean effect is probably still cost-effective). That is, since information can be shared with others freely and passed down, there may be some mass media interventions whose effect extends far beyond their actual campaigns. Overall, I recommend proceeding with this investigation to examine mass media charities in more detail.
About Founders Pledge
Founders Pledge is a community of over 1,700 tech entrepreneurs finding and funding solutions to the world’s most pressing problems. Through cutting-edge research, world-class advice, and end-to-end giving infrastructure, we empower members to maximize their philanthropic impact by pledging a meaningful portion of their proceeds to charitable causes. Since 2015, our members have pledged over $8 billion and donated more than $800 million globally. As a nonprofit, we are grateful to be community-supported. Together, we are committed to doing immense good. founderspledge.com
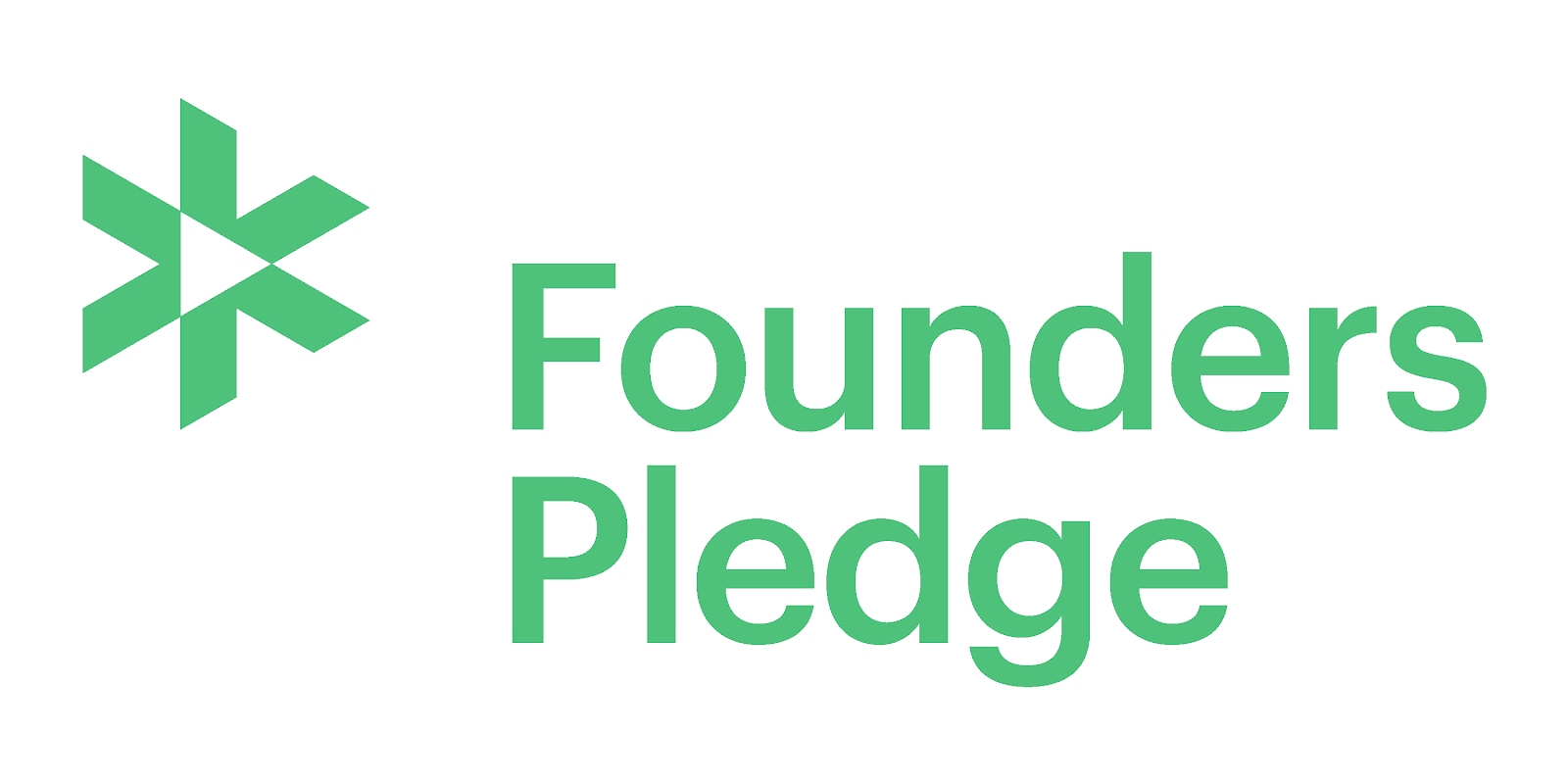
Bibliography
Muthukrishna, M. (2020). Cultural evolutionary public policy. Nature Human Behaviour, 4(1), 12–13.
- ^
I could not find broad data on people’s healthcare knowledge (for example, a survey of general healthcare knowledge across people living in different countries). However, while healthcare misconceptions are somewhat universal, I found a large number of examples of healthcare misconceptions in LMICs. For example, in prior work I came across the practice of infant oral mutilation in countries such as Tanzania, where traditional healers remove a child’s ‘tooth buds’ (emerging canine teeth) with a typically unsterilised tool, in the mistaken belief that this will prevent illness (Garve et al., 2016). Another example of a relatively common misconception in LMIC is that epilepsy is contagious (Newton & Garcia, 2012). There are many other misconceptions that I do not have space to list here, for example about albinism (Baker et al., 2010), effective contraceptive methods (Mbachu et al., 2021), and who is susceptible to COVID (Schmidt et al., 2020). I suspect that people living in LMIC often have more limited access to accurate healthcare information relative to people living in HIC, for example due to limited internet access; in 2019, only ~30% of people living in Sub-Saharan Africa used the internet (Individuals Using the Internet (% of Population) - Sub-Saharan Africa | Data, n.d.).
- ^
There are reasons to expect that cost-effectiveness will either decrease or increase after the first year. On the one hand, more people are newly reached in the first year—many of the people who listen to the campaign in year 2 will have already heard the campaign. On the other, costs are cheaper since there are no start-up costs.
- ^
For FEM: 2,240,000 women of reproductive age reached at cost of ~$400,000. Note that a large proportion of radio listenership is reproductive-age women. In addition, the radio stations that FEM works with have a large audience, and FEM are an especially lean organisation: together, these factors mean that FEM are able to reach a large group of people relatively cheaply.
- ^
One method is to assign people to conditions where they are deliberately exposed to a piece of media or not, for example at a group screening (the strategy of Banerjee et al. 2019). The problem here is that it is difficult to do this while mimicking a normal media environment—where there are many sources of information competing for attention at once, and where viewers or listeners are not explicitly told to attend to one particular media item.
- ^
We have granted to FEM in the past, through the Global Health and Development Fund.
- ^
Note that if we exclude long term effects entirely, I still estimate FEM’s cost-effectiveness at 23x GD.
- ^
LiST is a fairly well-established tool within public-health; it estimates the impact of scaling up on maternal, newborn, child health interventions in LMIC contexts. Typical users include NGOs, government partners, and researchers. It was developed at Johns Hopkins, and funded by the Gates Foundation (About, n.d.). The data that informs LiST comes from various sources including DHS, UNICEF, WHO, UN and the World Bank. The authors use the LiST tool to estimate a cost per DALY at $99 from a provider perspective (i.e. not accounting for the costs of seeking out healthcare, from the parent’s perspective) and $111 from a societal perspective (taking the cost of health seeking into account).
- ^
Recall from the Background section the estimation that if 0.00015 < B (change in behavior) x E (effect of behavior change on mortality), this suggests the campaign will be cost-effective.
- ^
More recent communication with Anna Christina Thorsheim (executive director at FEM) indicates that this is likely to be possible.
Tim Hua @ 2022-11-17T22:57 (+11)
I am planning on giving a talk in EAGxBerkeley on this topic! There's a paper that is media RCT that looks at randomly showing Ugandan children a motivational movie (The Queen of Katwe) versus a placebo movie (Miss Peregrine’s Home for Peculiar Children). Watching the Queen of Katwe has a persistent and significant effect on educational outcomes of girls. It reduces chance that a femal student in 10th grade fail math from 32% to 18%, increased the chances that a 12th grade female student apply to college by 15 percentage points (which closes the gender gap between girls and boys), and does a bunch of other good stuff (see here: https://doi.org/10.1162/rest_a_01153 )
Here's the link to the slides if anyone is interested: https://timhua.me/eagx.pdf
Rosie_Bettle @ 2022-11-18T14:16 (+1)
Interesting, thanks for sharing! I checked out the slides and am now curious about the cultural effects of Fox News...
Yadav @ 2022-11-19T22:37 (+8)
I am going to link post a talk by Rowan Lund (from Family Empowerment Media) here in case people want to learn more.
Davidmanheim @ 2022-11-18T08:52 (+6)
Different types of media and strategies will have very different effects, and different interventions will have very different levels of effectiveness. Not only that, but this class of intervention is very, very easy to do poorly, can have negative impacts, and the impact of a specific media strategy isn't guaranteed to replicate given changing culture. So I think that treating "media interventions" as a single thing might be a mistake - not one that the program implementers make, but one that the EA community might not sufficiently appreciate. I don't think this analysis is wrong in pointing to mass media as a category, but do worry that "fund more mass media interventions, because they work" is too simplistic a takeaway. At the very least, I'd be interested in more detailed case studies of when they succeeded or failed, and what types of messages and approaches work.
Rosie_Bettle @ 2022-11-18T14:08 (+10)
Hi David, thanks making these points. I totally agree that there's likely to be a lot of variation between campaigns, and that examining this is a critical step before making funding decisions- I don't think (for instance) we should just fund mass media campaigns in general.
I did find it helpful to focus upon mass media campaigns (well, global health related mass media campaigns) as a whole to start with. This is because I think that there are methodological reasons to expect that the evidence for mass media will be somewhat weak (even if these interventions work) relative to the general standard of evidence that we tend to expect for global health interventions- namely, RCTs. This is because of problems in randomising, and of achieving sufficiently high power, for an RCT examining a mass media campaign. I think this factor is generally true of mass media campaigns (and perhaps not especially well-known), hence the fairly broad focus at the start of this report.
I agree with you though that ascertaining which programs tend to work is hugely important. I've pointed to a few factors (cultural relevance, media coverage etc), but this section is currently pretty introductory. The examples I've focused upon here are the ones where there is existing RCT evidence in LMICs (e.g. family planning is Glennerster et al., child survival from recognition of symptoms is Sarassat et al., HIV prevention is Banerjee et al.) Some things that stand out to me as being crucial (note that I'm focusing upon global health mass media campaigns in LMIC) include the communities at hand having the resources to successfully change their behavior, there being a current 'information gap' that people are motivated to learn about (e.g. the Sarassat one focuses on getting parents to recognise particular symptoms of diseases that could effect their children, and the Glennerster one provides info about the availability and usage of modern contraceptives), cultural relevance (i.e. through the design of the media) and media coverage.
Rosie_Bettle @ 2023-03-07T19:11 (+1)
Note that I made an edit to this report (March 2023) to highlight that the per person reached cost estimated for DMI includes RCT costs; their per person costs to scale-up a program (aka when an RCT is not being run) are significantly cheaper. I am looking into this in more detail at present.
Brendon @ 2022-11-23T22:20 (+1)
Cleverly crafted, strategic and creative mass media can be very effective and become cultural. It is overlooked in philanthropic circles and is largely captured by big business. The elite skillsets behind great campaigns is also greatly under appreciated outside the agency world where top talent is sort after and paid a premium.
Having produced many mass media campaigns myself a great many things must align to achieve great results.
The other big problem with mass media campaigns is strategy. Too often organisations focus on the negative which can be valid but they lack aspiration or a clever story to connect with. You can show kids in need and show them suffering or you can craft an aspirational story that shows what donors money can do to make them thrive or an impactful story about how a single child saved from malaria went on to do great things. You can show endless chickens in cages suffering or you can show how much chickens love being outside with some humour to make it land well with a general audience. If the focus is coming from the negative then it needs to be creative and clever like Save Ralph.
Mass media is a blank canvas and just paid time and space what really matters is the creative.