Part 2: AI Safety Movement Builders should help the community to optimise three factors: contributors, contributions and coordination
By PeterSlattery @ 2022-12-15T22:48 (+34)
Epistemic status
Written as a non-expert to develop and get feedback on my views, rather than persuade. It will probably be somewhat incomplete and inaccurate, but it should provoke helpful feedback and discussion.
Aim
This is the second part of my series where I attempt to outline a theory of change for Artificial Intelligence (AI) Safety movement building. Part one conceptualises the AI Safety community. Having positioned AI Safety movement building within the broader context of the AI Safety community, I next outline three factors or outcome metrics for this work group to focus on.
I take concerns about the downsides of AI Safety movement building seriously (e.g., 1,2). I am unsure whether we should accelerate recruitment into AI Safety work and, if that is a good idea, how we should do it. I therefore want to understand how different viewpoints within the AI Safety community overlap to help determine what, if any, types of movement building are predominately seen as helpful.
TLDR
- I reiterate that the AI Safety Movement Building group are like a ‘recruitment and operations team’ for the AI Safety community.
- I suggest three factors/outcomes that they should focus on: contributors, contributions and coordination.
- I clarify:
- Why the factors/outcomes suggested are not necessarily proxies for progress on reducing AI risk.
- Why I split out ‘contributions and coordination’ rather than lumping them under productivity
- I request constructive feedback.
Context from Post 1
I argued that AI Safety movement builders are like a ‘recruitment and operations team’ for the AI Safety community. They don’t strategize about how the AI Safety community sets or achieves its goals of mitigating AI risk or how other AI Safety groups (technical, governance or strategy) set or achieve their related sub-goals. Instead, their strategy and action is focused on how to do movement building to best support these groups.
What does success look like for AI Safety movement builders?
The success condition for AI Safety movement builders is the AI Safety community being satisfied that everyone with the relevant comparative advantage is working on AI Safety as efficiently as possible.
Progress towards this success condition can be approximated by progress on three underlying factors: i) contributors, ii) contributions, and iii) coordination.
The first factor (contributors) captures the need to have everyone with relevant comparative advantage working on AI Safety. The second (contributions) and third (coordination) capture the need to be sure that all contributors are working together as effectively as possible.
I’ll start by outlining the key factors/outcomes. I will then offer some quick examples for evaluation metrics, and for desirable and undesirable outcome states. I’ll finish by applying the model in the context of government, as a continuation of the analogy used in the previous post.
Factors/Outcomes defined
Contributors are individuals making positive contributions to AI Safety
Contributions are the contributors’ inputs into AI Safety work.
Coordination is understanding and aligning contributions to ensure maximal efficiency.
Example metrics
Contributors
- The number of workers in specific roles
- The potential number of workers in specific roles
Contributions:
- Hours worked
- Assessments of performance
- Publications/Citations
- Organisations started
- Hires made
Coordination:
- Satisfaction with the AI Safety community's collaboration, cohesion and shared knowledge
- Awareness/understanding of key researchers, agendas, papers and/or concepts
Examples of good and bad outcome states
Contributors
Good outcome state:
- We have evidence that we have effectively reached nearly all individuals who we expect to be able to contribute to AI Safety now or in the future.
- Close to 100% of relevant students/professionals are aware of and amenable to the key arguments and opportunities for working on AI Safety
- We can assess and monitor trends in the number of people doing direct work. We know when things are getting better or worse.
Bad outcome state:
- We are unsure how many contributors we have, could have, or have reached.
- Where we know of a useful cohort, we don’t know the percentage who are aware of the best arguments and related funding opportunities available to them.
- We have collected this data and know that this percentage is relatively small.
- We cannot or do not assess and monitor trends and don’t know if things are getting better or worse.
Contributions
Good outcome state:
- We have evidence that we have effectively maximised the contributions of people working on AI Safety.
- A representative sample of contributors (e.g., technical safety researchers) report 40 hours per week of average output, peak performance, good health, and no needed resources. Their outputs are at, or above, what could reasonably be expected.
- We can assess and monitor trends and know when things are getting better or worse.
Bad outcome state:
- We are unsure what percent of current contributors are being blocked by addressable issues (e.g., bad equipment, mental health or financial need)
- A representative survey of contributors (e.g., technical safety researchers) suggests that the average researcher is producing only 75% of their potential hourly output and 60% of their potential performance output. They attribute this to mental health issues, and a lack of access to computing resources.
- We cannot or do not assess and monitor trends and don’t know if things are getting better or worse.
Coordination
Good outcome state:
- We have evidence that we have effectively maximised the coordination of people working on AI Safety technical work, for instance, due to one or more of the below.
- Organisational leaders report satisfaction with current levels of collaboration, regular contact with other organisations and high awareness of relevant research agendas.
- Researchers demonstrate high awareness and understanding of relevant research, researchers, and organisations.
- Every AI safety researcher / top-tier PhD Student who wants an intern has one.
- We can assess and monitor trends and know when things are getting better or worse.
Bad outcome state:
- We don’t understand the standard of coordination of people working on AI Safety technical work
- Organisational leaders report dissatisfaction with their current levels of collaboration, irregular contact with other organisations and low awareness of relevant research agendas.
- Researchers demonstrate low awareness and understanding of relevant research, researchers, and organisations.
- Many AI safety researchers / top-tier PhD Students who want an intern lack one
- We cannot or do not assess and monitor trends and don’t know if things are getting better or worse.
Summary illustration
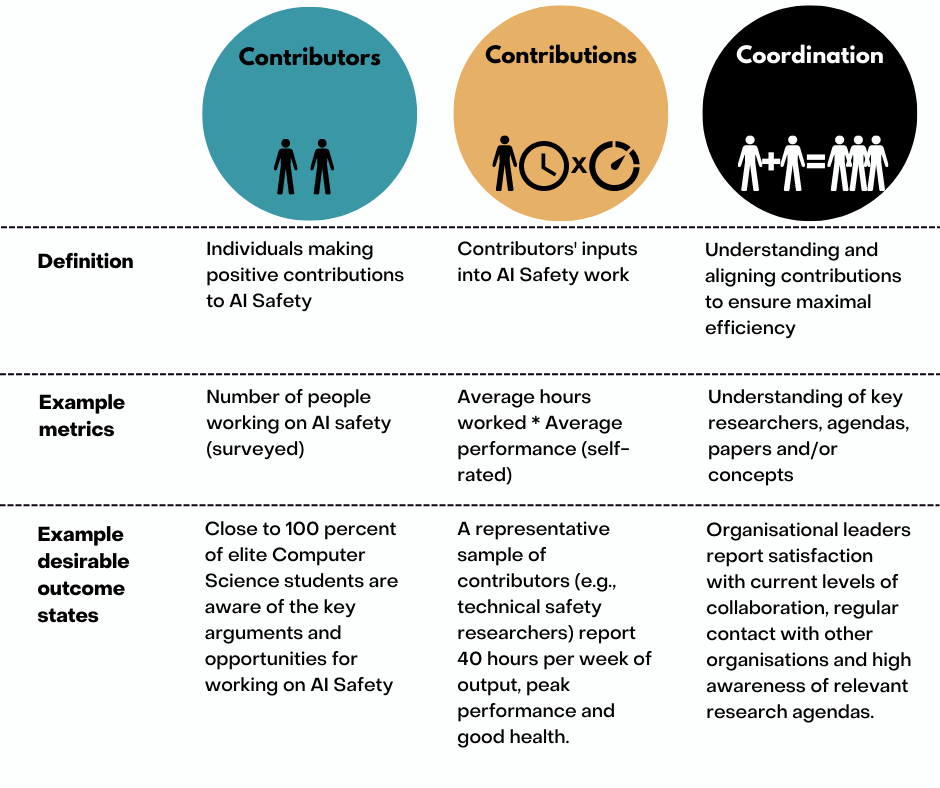
Applying the model in the context of government
To help demonstrate how the model works, I now apply it to a government (continuing the analogy used in my earlier post).
In this case, AI Safety movement builders would be akin to a government ‘recruitment and operations team’ trying to improve i) contributors, ii) contributions, and iii) coordination within government. In this context:
Contributors would be civil servants and supporting staff etc. Progress in optimising the number of contributors might be tracked, for example, by surveying the heads of government departments to track how well different categories of human resources needs were met. Groups of potential recruits could also be surveyed/engaged to see whether they all knew of key opportunities and incentives to work in government.
Contributions are the amount of time (and performance within that time) that civil servants and supporting staff contribute to the government. Progress might be tracked, for example, by surveying a representative sample of relevant civil servants to understand if their work is being hindered by addressable issues (e.g., bad equipment or mental health). Progress might also be measured by tracking metrics such as total employee hours committed to projects, sick leave, and projects completed.
Coordination is the extent to which these individual contributions converge to efficiently achieve the outcomes that the government desires. We might track progress in optimising coordination by (for example) surveying the heads of similar government departments to track how well they understand each other’s goals and projects, and/or a representative sample of relevant civil servants to test awareness and understanding of key concepts, projects or directives.
Clarifications
These factors/outcomes track progress in movement building, not necessarily progress in AI safety
Success in movement building will only reduce the risk of AI related catastrophe if the AI Safety community and its constituent groups have good strategies. We shouldn’t assume that just because we have more people who are contributing more time to AI safety in a more coordinated way, we are getting closer to solving AI safety issues.
Why are ‘contributions’ and ‘coordination’ separate factors?
This framework could have had just two factors: contributors and productivity. However, I decided to divide productivity into ‘contributions’ and ‘coordination’ because a focus on productivity could mislead readers into focussing too much on individual researchers: for example, ‘does Person X have good physical and mental health and the technology they need to do many regular hours of focused work?’. This could lead them to overlook more abstract and systemic issues that impact productivity: for example, ‘Does Person X know/understand the ideal theory of change, and have access to similarly-aligned mentors, networks, and collaborators?
Feedback
Does this all seem useful, correct and/or optimal? Could anything be simplified or improved? What is missing? I would welcome feedback.
What next?
In the next post, I outline specific practices (e.g., marketing/coaching), skills (e.g., Google Ads/CBT) and projects (e.g., talent searching/providing early career support) that are candidates for useful ways to work on each of the factors discussed.
Acknowledgements
The following people helped review and improve this post: Amber Ace, Bradley Tjandra, JJ Hepburn and Greg Sadler, Michael Noetel, Thomas Larsen, Jamie Bernardi, David Nash, Chris Leong, Steven Deng, Alexander Saeri and Emily Grundy. All mistakes are my own.
This work was initially supported by a regrant from FTX to allow me to explore learning about and doing AI safety movement building work. I don’t know if I will use it now, but it got the ball rolling.