[Cause Exploration Prizes] Measuring Happiness
By Open Philanthropy @ 2022-08-24T10:35 (+5)
This anonymous essay was submitted to Open Philanthropy's Cause Exploration Prizes contest and published with the author's permission.
If you're seeing this in summer 2022, we'll be posting many submissions in a short period. If you want to stop seeing them so often, apply a filter for the appropriate tag!
“If you cannot measure it, you cannot improve it” - Lord Kelvin
TL; DR: at the moment, we lack measures of hedonic happiness—yet many people are hugely interested in improving the happiness of sentient beings as a way to do good in the world. However, recently developed biopsychological approaches—that will be further improved via advances in AI—can provide us with the techniques to do this, in both humans and non-human animals. This seems to be a neglected scientific area; to the best of my understanding, most scientific funding bodies are not especially interested in measuring happiness. Yet investing in these techniques could enable new breakthroughs in happiness research.
Summary
Effective altruists aim to do the most good, and most people’s conception of ‘good’ encompasses increasing the happiness of sentient beings. Measurement is key towards improving a given outcome; it facilitates the ability to recognise the causes of that outcome, and a way to track that outcome following particular interventions. For example, economists use measures such as consumption and GDP (at different levels of analysis), while global health utilises measures such as DALYs. Yet happiness—sometimes argued to be the most important underlying characteristic—is reliant upon self report measures of wellbeing.
There is a significant need for measures of the affective component of happiness. Collecting affective data (rather than relying solely upon self-report scores of life satisfaction)[1] is important because (1) non-human animals (and young children) cannot provide self-report data; (2) life satisfaction scores are not measuring ‘happiness’ per se—and many effective altruists are primarily concerned with the hedonic component of happiness; and (3) self-report has its limitations. In particular, it takes a lot of effort to collect, cannot be collected automatically, is somewhat prone to bias, and cannot provide continuous longitudinal data. Richer datasets (that would become possible through the development of affective measures) are key to advancing our understanding of happiness.
However, biopsychological approaches are not far off being able to measure affective happiness. OP could invest into research that aims to further improve these techniques, and I believe that this area is now especially tractable due to advances in AI. In particular, I highlight research that tracks the affective states of other animals by using AI to measure their facial expressions. Other measures (more aimed at humans) use approaches such as smart-watch based measurement of bodily states, which are used in conjunction with machine-learning algorithms to predict emotional states. Further into the future, it is likely that it will be possible to measure the affective component of happiness via its neural correlates. Happiness measurement is ‘off the radar’ for many non-philanthropic funders, but this data (and the ability to run experiments to test happiness interventions) could provide key insights into improving global wellbeing.
Why is this important?
Animals cannot report their own wellbeing
Accurate recognition and assessment of wellbeing are critical for animal welfare,[2] but animals are obviously unable to provide self-reports concerning their wellbeing. Farm and zoo animal welfare is sometimes assessed via behavioural methods (e.g. a zookeeper would note hair-pulling as a behavioural indicator of poor welfare) but these methods are not especially well-used, due to the workload demand to the observer.[3] In addition, note that these methods are liable to miss individuals suffering from poor wellbeing—many animals (such as the domestic cat) appear to have evolved methods of avoiding the overt display of cues that they are in pain, in order to avoid predation.[4] Other methods focus upon health indicators, such as the presence of open sores. Clearly, these health-based measures of welfare are important—but many animals that are physically well may nonetheless have poor wellbeing.
Tracking the affective states of animals would likely uncover surprising new cause areas for improving animal wellbeing. For example, global health metrics such as DALYs guide the decisions of funders and policymakers, by making various illnesses comparable across geographic regions. Building up data about animal wellbeing would analogously allow us to better identify the largest (and most neglected) causes of animal suffering, which may be counter-intuitive. Note that while we have begun to uncover some of the causes of human happiness and wellbeing, we have barely scratched the surface of this in other animals; and indeed there is additional reason to expect these causes to be counter-intuitive, bearing in mind that the inner mental lives of other species may be very different to our own.
Furthermore, this is a speculative point, but I think that using measures which track affective states could be highly effective in shifting policy and behavior towards initiatives that foster animal wellbeing. As a policymaker, imagine being presented with data which strongly suggests that ‘animals kept in condition [x] are far happier than animals kept in condition [y]’; this would seem to be persuasive evidence to pick condition [x]. One could also imagine this kind of data being used to guide consumer behavior.
Affective measures would make it possible to collect new types of data
Measuring the affective state of happiness entails using psychometric measurements: that is, measuring bodily states (such as facial expressions, heart rate variability, activity in brain areas) to get insight into that individual’s hedonic state. An example would be using cameras to track the facial expressions and posture of a group of chickens, then using AI (trained on a set of photos) to assess whether they were in pain. A more direct approach (that is not currently practical, but might be in the future) would be to directly measure the neural correlates of happiness. Unlike survey-based measures, these psychometric measures are (1) able to capture ‘moment-by-moment’ happiness, (2) automated, and (3) arguably less prone to bias.
With regards to the first point, note that survey questions usually ask people to make a judgment over their life (or a substantial period of time) as a whole.[5] For example, Gallup World Poll surveys use the ‘Cantrill Ladder’ approach, where participants are asked to think of a ladder, with the best possible life for them being a 10 and the worst being a 0: they must then rate their life on this scale. In comparison, measuring the hedonistic affect of happiness would provide continuous data. This is important because happiness is not necessarily a ‘set point’ phenomena, but rather is a volatile emotion that changes over time and is sensitive to environmental conditions.[6] Continuous data would provide insight into the day-by-day determinants of happiness, an area which is very underexplored.
Second, these psychometric measures can be automated: the person would not need to be prompted to think about their own wellbeing, or to be surveyed by another person—making it logistically feasible to collect far larger amounts of data. For example, we could track the happiness of a group of individuals/ animals at one time.
Third, there is reason to think that psychometric measures of affective happiness may show less bias (and sensitivity to cultural context, e.g. how one interprets the self-report questions) than self-report. Note that I consider self-reports of subjective wellbeing to be valid measure, but there are a number of circumstances where we might expect someone to either lie, or to be genuinely mistaken about their own wellbeing. While most people think they know when they are happy, sad or stressed, people’s own emotional awareness can be surprisingly poor: imagine someone who thinks that they are fine only to realise that they are chronically burnt-out, or on the edge of a depressive episode. People’s memories are also famously fallible;[7] when we try to consider our overall levels of happiness, it is likely that we are falling victim to the same biases. At present, we have little way to test this since our primary methods are self-report.
Overall, this data could also help us to ‘unravel’ current cruxes of happiness research. For example, take the Easterlin Paradox, or the (debated)[8] finding that ‘at a point in time happiness varies directly with income, both among and within nations, but over time the long-term growth rates of happiness and income are not significantly related’.[9] One of the leading explanations for this paradox is the idea of social comparison; that at any given moment in time, wealthier people are happy since they compare their wealth to poorer people. However when the population as a whole increases their wealth, this does not increase happiness in the same way since there is no equivalent comparison to be made. Richer datasets could help us to get at this question, because we could specifically examine how social comparison affects happiness within individuals—that is, testing at this level of analysis may provide the insights that we need to understand the macroeconomic data. For example, we could test what happens immediately after someone makes a social comparison to others (and finds that they are at an advantage with regards to metric [x]), and compare this to data looking at how happy people are when they discover that the community as a whole has benefited in metric [x]. Affective data would allow us to look at the data over time, and may be less vulnerable to social desirability biases.
At a more ‘meta’ level, I think that a larger amount of happiness data (that would be facilitated via the collection of affective data) could help us better understand the causes of wellbeing. If we look at health, the collection of huge quantities of health data has allowed us to pinpoint causes of poor health—many of which were unintuitive at the time (such as smoking!). This is a little speculative, but it seems very plausible that similar data for happiness might uncover other unexpected causes of poor/ good wellbeing.
We should measure hedonic happiness, alongside life satisfaction
Happiness consists of hedonic experience: the balance of pleasant over unpleasant experiences. In contrast, subjective wellbeing additionally gets at factors beyond happiness.[10] Imagine being asked to think of a ladder, with the best possible life being a 10 and the worst being a 0, and then being asked to rank your life on this scale. You might include some evaluation of the balance of positive and negative affect over your life (happiness), but you would likely also include other aspects—such as evaluation of whether you are reaching your life’s goals.
It is not my aim here to get into a debate about which metric(s) effective altruists should aim to maximise, as I’m sure that others have done this far better than I could. However, I think it’s clear that many people do place moral weight upon increasing the affective happiness of other beings—perhaps not to the exclusion of other metrics,[11] but with the understanding that this is a critically important metric. Yet we have been forced to rely upon life satisfaction measures, perhaps primarily for practicality reasons.[12] Recent scientific advances—aided by AI breakthroughs—mean that we can more directly measure happiness.
Does it matter, whether we use subjective wellbeing scores versus measures of the affective experience of happiness? From a philosophical perspective, the version that you prefer depends upon which strand of utilitarianism (if any) you favour;[13] hedonists would presumably value affective data more highly, while people who value aspects of wellbeing (such as having one’s goals met) might weight subjective wellbeing self-report data more highly.
From a more practical standpoint, a key point is that we really need a measure of affective experience to examine animal wellbeing, since we cannot ask them how their life is going. Looking at humans specifically, there does appear to be a correlation between measures of emotional wellbeing (which I take to be a closer proxy of the affective experience of happiness) and life satisfaction—but there are also key differences. For example, the hedonic experience of happiness appears to be uncorrelated with education, varies over days of the week, improves with age, and responds to income only up to a threshold. In contrast, evaluative measures remain correlated with income even at high levels of income, are strongly correlated with educations, are often U-shaped in age and do not vary over days of the week.[14] To understand happiness, I think it is necessary to account for affective happiness as well as wellbeing.
Is it possible to measure affective happiness?
My purpose in this section is not to necessarily provide a thorough recommendation of which technique to focus upon, but rather to establish that there are existing techniques (as well as new techniques in the pipeline) that are promising candidates to measure and track happiness.
I think it is helpful to provide a quick overview of what happiness is, from an evolutionary and neuroscientific standpoint. Neural mechanisms that generate affective reactions are present and similar in many animals (particularly mammalian brains), and presumably have been selected for.[15] Importantly, within the neurosciences the study of happiness has focused upon its hedonic component (as opposed to eudaimonia, or a sense of meaningfulness and engagement in life—which I take to be roughly analogous to the ‘evaluative’ component of wellbeing stressed in social sciences research) since it is easier to evoke hedonic responses in laboratory animals. Studies with rodents have found a ‘pleasure network’ in which larger activation results in larger pleasure reactions—these networks include regions of the brain such as the nucleus accumbens, limbic regions of the prefrontal cortex, anterior cingulate cortex and midinsular cortex.[16] One possibility is that the hedonic brain circuits that are implicated in pleasure and reward (i.e. that have evolved to encourage animals to seek out resources such as food and mates) interact with other brain systems relevant to self-understanding, at least in humans—creating eudaimonia.[17]
Given that happiness is a neurobiological phenomenon, this suggests it is possible to measure. At present, some of the best ways to measure the affective state of happiness rely on proxies that are very responsive to the behavior of the nervous system—such as facial expression and heart rate variability. In the future, I think it is likely that we will be able to more directly measure the neural correlates of happiness.
AI and facial expression
Facial expressions (alongside posture) can be used to measure track affective states, at least in non-human animals. That is, it is possible to train up an AI to recognise and measure different emotional expressions, allowing the measurement of wellbeing. To the best of my knowledge, this has been most extensively examined in animals—there is a rich literature looking at the emotional expressions of mammals in particular, and research looking at the emotional expression of pain in taxa beyond mammals. Consequently, I think that a key use of such technology could be to improve welfare standards in factory farming, and to quantify and recognise wild animal suffering.
Facial expressions in mammals are widespread, and many facial movements are evolutionarily conserved across species.[18] There are a number of papers examining the specifics of emotional responses in other animals—for example, generalised facial relaxation has been linked to positive affect in humans, and the play face in many primate species (involving a relaxed expression with an open mouth) is strongly linked to a positive affect. In horses, the relaxation of the upper eyelids and ears is thought to indicate a well state.
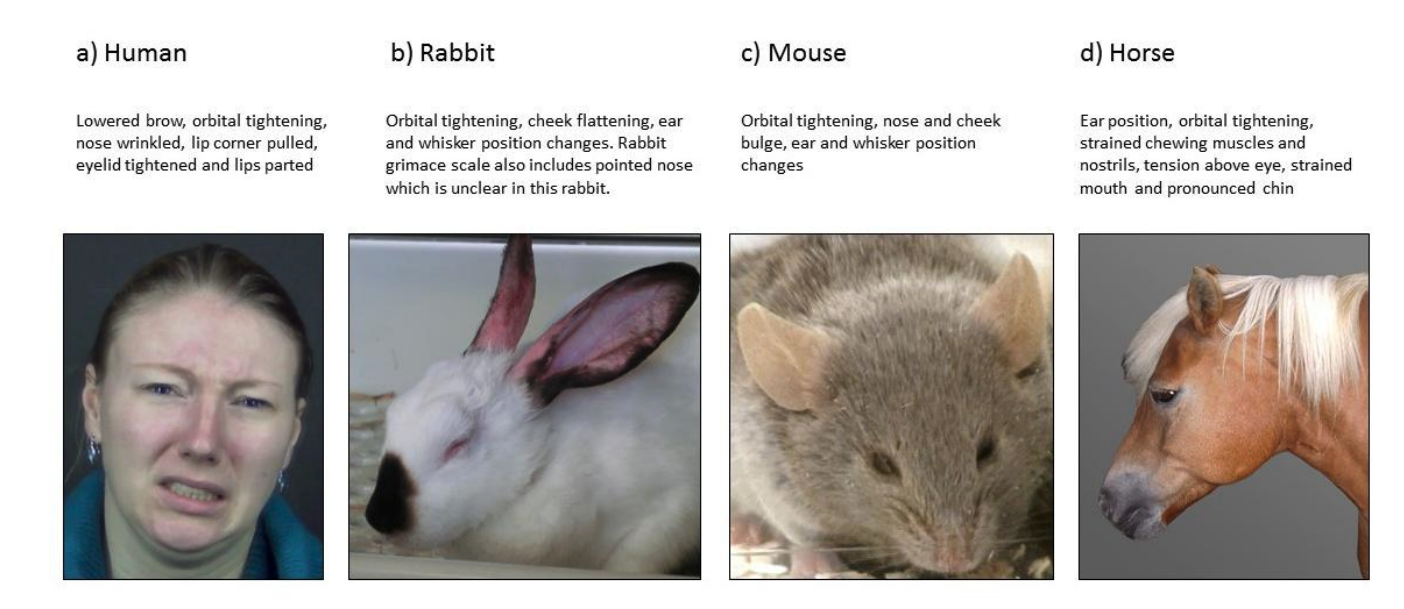
Emotional expressions are consistent markers of wellbeing within species. A recent paper successfully developed tools (one using machine learning from a dataset of cat facial expressions, and the other neural networks) to automatically recognise whether cats are in pain—they tested the tools with photographs, and apparently found an accuracy of over 72%.[19] Another platform has already been developed to automatically measure the emotional/ mental states of cows and pigs.[20] The technology uses facial recognition technology, alongside Python-based algorithms, in order to (1) recognise which animal is which, and (2) correlate changes in facial expression to mental and emotional states. I’m still not quite sure just how ‘ready’ this technology is to be used, and I am a little skeptical if these accuracy measures are to be believed—nonetheless, this area seems promising.
With regards to humans, I could not find the same volume of work. However, I did find work creating real-time automated systems to recognise human facial expressions.[21] On the one hand, it could be the case that emotional expressions are more specific to the individual (and/ or culturally specific) in humans compared to other animals—making the development of AI algorithms to track these expressions more challenging. Along similar lines, humans may be more skilled at hiding their emotional expressions compared to other animals. At the same time, I’m not convinced that either of these barriers will prove impenetrable towards AI that aims to measure our affective states—but I am unsure about privacy concerns for this kind of data.
While I don’t think that these platforms are necessarily perfect already, I think that the animal platforms in particular are already very promising. In general, AI offers a huge potential towards improving animal welfare by giving us the ability to track the affective states of other animals (who obviously lack the language to otherwise tell us how they are doing). I have focused upon facial expressions here, but this kind of method could potentially gain additional accuracy via the combination of multiple sources of information: such as thermal imaging, body posture information, vocalisation data, etc. Consequently, I think that investing in this technology—both in terms of its development, and scaling it up–could be a hugely impactful way to increase animal welfare. Ultimately, it could also be possible to film wild animals (e.g. with a drone) then use these same techniques to gain insight into their wellbeing.
Sensor-based approaches (aka on smartwatches)
This method is more predominantly used for humans, although note that the general principles (of using sensor-based information to track affective states) also apply to non-human animals. One potential benefit to using such methods (rather than observing the face by use of a camera, as in the method outlined above) is that sensor-based information does not require facial images.
Wearable sensors, such as those concealed within smartwatches, can provide emotional information via cues from heart rate, posture, noise and acceleration.[22] Some of this work used an ‘emotive couch’ (yes, really)—a couch set up with a bunch of concealed electrodes, designed to measure the person’s positions in order to get insight into their emotional state (via the use of machine learning techniques). They did not manage to achieve great accuracy, although it was better than chance (62.2% accuracy, when deciding between the three emotions of Relaxation, Interest and Anxiety). However, I think it’s an interesting proof of principle.
Subsequent work has started to improve these measures. For example, a more recent smartwatch based method (which had a stage where individuals manually ranked their own happiness levels, allowing the smartwatch app to learn that individual’s psychometric profile for happiness) tested a variety of algorithms, finding that one had an accuracy of 88%.[23] This is using data from a variety of sensors on the smartwatch; an accelerometer, a heart rate sensor, a microphone, a position sensor (analysing where the person was), and information about the weather. I would still take this finding with a grain of salt—for example, I’m unclear of the importance of the psychometric measures versus location in facilitating this level of accuracy—however, this nonetheless suggests that such an approach is feasible. With increasing advances in machine learning techniques, it seems likely that this will work.
Neural correlates of happiness
The methods that I have described above use proxies for happiness—but happiness is a neurobiological phenomenon. Ideally, it would be preferable to measure happiness more directly. To the best of my understanding, this technology is significantly further afield than using AI and facial expression, or sensor-based methods. Nonetheless, I think it is very possible that this technology will emerge in the future.
An ideal measure of happiness would quantify the activity of neurons that activate reward circuits in the brain. At present, one evolving method is to use neuroimaging approaches—these approaches work by estimating the energy consumption changes in the brain. Participants who are inducted to feel happy (i.e. by recalling happy memories) are put into a scanner, and their brain activation responses are compared to a control person (i.e. who is asked to recall a neutral memory) to try and work out which brain regions are specifically active during periods of happiness. At present, a number of brain regions have been highlighted via this general method—including the anterior cingulate cortex, prefrontal cotex and orbitofrontal cortex.[24] Indeed, there is evidence for clear anatomical signals of at least five basic affective emotions; happiness, sadness, fear and disgust.[25] It is possible that further developments might make it possible to achieve a greater level of granularity–at present, it is not possible to tell ‘how happy’ someone is via these kinds of scans (and clearly, it would be impractical to give someone a PET/ fMRI scan whenever you wanted to measure their happiness). For these reasons, I think that EEG (below) has more potential.
Another idea would be to support research that uses EEG methods:[26] using electrodes to measure electrical brain activity via the scalp. It is theoretically plausible that EEG could capture affective information, since (very small) electrical signatures are caused by brain networks that are active during emotion. The problem is that EEG most clearly captures electrical information from the outer layers of the brain, but many regions that are theorised to be involved with emotions are deep in the brain, for instance in the limbic structures and subcortical regions.
Nonetheless, recent breakthroughs in how the electrical signals are processed appears to be surpassing this problem; a recent paper using this method claimed an accuracy rate (of classifying 9 emotional states) of 89%.[27] To the best of my understanding, the current limitations are to do with the machine learning algorithms which process the electrical signals—I therefore expect that this area is quite tractable. We might expect EEG devices to become smaller, alongside the advancement of other brain-computer interfaces. Overall, I view this approach as ‘further off’ than sensor-based methods—but I do expect this technology to be developed.
Neglectedness
I think that this is a very neglected area. Effective altruism is concerned with increasing the happiness of sentient beings, and takes a scientific approach to improving good—yet we lack a way to actually measure happiness. I am not aware of any grants to similar initiatives from EA aligned organisations.
To the best of my understanding, this is a research area that does not attract much scientific funding. My intuition is that it is too speculative and high risk, and many funding organisations (across both human and animal funding groups) are more concerned with health metrics than wellbeing metrics. While it is difficult to find specific numbers to test my intuitions, most of the research grants I could find tended to focus upon the philosophy of wellbeing/ positive psychology, rather than the measurement of happiness per se. For instance, see recent happiness and wellbeing grants.[28]
It is quite striking that some techniques (e.g. on using AI to measure affective states in farm animals) seems to be fairly close to being ready to be put into the real-world.
Some of the many reasons I could be wrong:
1. We already know what animals need for their welfare, it’s better to go ahead and do that than focus on a measure of wellbeing
This is something I am still wondering about. However, I think that we need more wellbeing data to convince people to place animal wellbeing measures into policy, and that it will be a lot easier to collect large amounts of data via these measures that can be collected automatically (rather than asking someone to take behavioral measures via ethogram). In addition, I think we are liable to uncover unexpected ways of improving animal wellbeing via affective state monitoring—we don’t really know what’s going on in animal minds, and it’s very possible that we are suffering from anthropomorphic biases and getting it wrong/ missing non-intuitive ways to improve their wellbeing.
2. Maybe measuring the affective state of happiness would lead to an overwhelming focus on it, to the detriment of other morally-relevant qualities
I was somewhat concerned about this (I am not a hardcore utilitarian). However, we collect health data without viewing it as the most important metric of all time— I don’t think that collecting this data would actually lead to an overwhelming focus upon it.
3. Maybe it will turn out that these psychometric measures aren’t really measuring the affective state of happiness
In humans, it’s pretty easy to validate this data (by asking people ‘what are you feeling at this moment?’ and seeing if the measures agree). In animals, it is trickier— but contextual cues can be used to validate (i.e. a more intensive version of ‘that lamb is pulling the same face as when it was playing, so this is likely a pleasant emotional state’).
4. We should be worried about privacy. Say that we measured people’s happiness regularly, at the doctor. Could this data be used against us?
I am also concerned about this. I think that affective happiness data would need the same protections as health data.
5. Maybe measuring happiness won’t actually lead to new insights
It’s possible, but I think that few people would historically have predicted the kinds of insights that extensive health data has produced (for example, that smoking is bad).
6. Happiness data and subjective wellbeing data will lead to the same conclusions anyway
I think that a lot of the time, they will do. But the affective data still seems likely to diverge from wellbeing with regards to numerous important aspects (e.g. wealth). I also think that the affective data may help to pull apart existing cruxes in happiness research, and to create substantial happiness datasets more generally. Plus of course, we don’t have subjective wellbeing data for animals.
Open questions
With more time, I would have gone deeper into testing my assumption that ‘new data will create new insights into happiness, which will allow us to better promote global wellbeing’. I suspect that this is true, but I think it would have been better to test this further, for example by looking at historical data—how did measuring health metrics improve the effectiveness of global health bodies? Are there any reasons that wellbeing data might not follow the pattern laid out by health data?
Conclusions
We do not yet have a way to measure the affective component of happiness, despite its importance towards understanding how to do the most good in the world. At the moment, we rely upon self-report measures of wellbeing. However, technologies that will allow us to measure affective happiness are not far off—although they are under-resourced. We should invest in these technologies, and scale their use up in areas where we expect they would be of the most use (for example, a potential use that is not far off would be to monitor farmed animal wellbeing). As a longer term aim, we could begin to build up larger happiness datasets that are more equivalent to the kinds of data that we use within global health today. Richer data has the potential to shift our understanding of the determinants of happiness, and to enable researchers to make theoretical breakthroughs in happiness research.
- ^
Note that I think self-report of wellbeing is a valid and hugely useful measure—but I still think it’s important to be able to measure actual happiness.
- ^
- ^
- ^
- ^
One exception to this comes from experience sampling methods, where participants are prompted to record their feelings at least once per day; however this method is expensive to implement, and risks being intrusive.
- ^
- ^
- ^
- ^
- ^
- ^
- ^
- ^
- ^
- ^
- ^
- ^
- ^
- ^
- ^
- ^
- ^
- ^
Roessler and Gloor. They measured the accuracy via an experience-sampling method (so a notification would pop up, asking the person about their current emotions).
- ^
- ^
- ^
- ^
- ^