Potentially great ways forecasting can improve the longterm future
By Linch @ 2022-03-14T19:21 (+43)
Summary
See companion post here.
In addition to the EA Early Warning Forecasting Center I outlined in my other post, I think there are several ways forecasting may be very useful for longtermism, including:
- Forecasting as a way to amplify EA research
- Prediction-evaluation setups as a way to improve EA grantmaking
- Large-scale broad forecasting as an EA outreach intervention
- Large-scale forecasting tournaments as a talent training and vetting pipeline
- The dream: high-quality, calibrated, long-range forecasting (ideally also at scale and on-demand)
Finally, an entirely different theory-of-change for forecasting is to consider broad, mass-appeal forecasting as a general epistemics intervention, that is, improving the thinking and reasoning quality of society at large. I think this is potentially pretty interesting and promising, and probably net positive, but am generally uncertain of the sign. This is mostly because I worry about squandering the epistemic edge that current broadly altruistic and EA(-adjacent) actors have over the rest of the world.
Further work, from myself and others, would consider and prioritize both among this list and within specific organizational choices in this list (including further research, starter projects or organizations to initiate, grants that are worth making, etc). Further work should also include a red-teaming of this vision, and other refinements.
Most of the points in this post will not seem original to people extremely acquainted with the EA forecasting space. Nonetheless, I thought it may be helpful to bring much of it in one place, as well as include my current opinionated best guesses and judgements.
Forecasting as a way to amplify EA research
There are a number of questions that come up during research for which I think forecasting could be one useful tool. Structural properties of such questions may include them being more amenable to broadly outside-view-style reasoning as opposed to deep internal models, being relatively “clean”, having a semi-objective resolution criteria, being more evaluative than generative (e.g., not questions that require coming up with new policy ideas), being about the future, etc.
Broadly, ways to use forecasting to improve EA research can be decomposed into two categories:
- The researcher personally comes up with forecasting questions and forecasts questions that are relevant to her own work.
- As much as possible, the researcher identifies forecastable questions in her own work and then delegates answering such questions to external forecasters, or forecasting aggregation processes.
- It’s also plausible to me that some of the work in identifying and operationalizing the relevant forecasting questions can be delegatable as well.
There are a number of reasons for why researchers may prefer delegating subsets of their work to external forecasters or forecasting aggregation processes. The two most important to me are: a) for some subset of questions, top forecasters may be much better at getting the correct predictions to them than current EA researchers, and b) speaking loosely, forecaster time is usually less valuable than EA evaluation time (at least / especially after accounting for the fact that it’s probably easier to use money to buy extra forecaster time than to buy extra EA evaluation time).
I’m excited about more work in this general direction because it’s moderately impactful, highly tractable, and very easy to do initial experiments with, compared to other ideas on this list.
Some researchers at Rethink Priorities have been experimenting with a number of different ways to use forecasting to improve our research. For example, we partnered with Metaculus to host a nuclear risk forecasting tournament, we asked a number of different questions on Metaculus related to EA philanthropy, and we also conducted our own survey of elite forecasters about cultured meat timelines (writeup forthcoming). Samotsvety and Sage are also working on this from the supply side, CSET Foretell has some projects, and there appears to be an increasingly high amount of research here.
Prediction-Evaluation setups as a way to improve EA grantmaking
The basic idea is that, as a way to supplement existing grantmaking efforts, we can have amplified prediction efforts to forecast what future evaluators of a project would say about a project (conditional upon further funding and investments in human capital). This allows us to have greater certainty about the quality of ideas, people, and institutions, thus increasing both the quality and quantity of future grantmaking.
For concreteness, you can imagine us having rapid forecasting teams to predict something like “5 years after Project X is funded for $AB millions, what is our best distributional guess for what a team of evaluators of Y caliber spending Z hours would consider to be their best-guess existential risk reduction estimates of Project X,” and use it as one of the (most important) inputs into whether to fund Project X.
Note that I think existing prediction and evaluation setups are currently not ready to do this well. Among others, we need a) better engineering setups to do forecasting at scale, and b) better ontologies for cleaner evaluations at scale, as well as addressing other foreseen and unforeseen (to me) bottlenecks. However, I don’t see strong in-principle reasons why this can’t be done well, and I’m excited for further research and experimental/entrepreneurial progress on this.
Ozzie Gooen and Nuño Sempere at QURI have been doing a number of experiments in this general direction.
Large-scale broad forecasting as an EA outreach intervention
Having legible forecasts by people known to be great at short-range forecasts may be a good EA outreach intervention. For example, Tetlock’s group is working on forecasting existential risks. As long as superforecasters give not-crazy (by longtermist EA lights) estimates of existential risks, then this gives students, policymakers, external researchers, and other groups we may care about a legible reason to care about existential risks, and this is a plausibly good early-pipeline recruiting funnel, in addition to some PR benefits that do not exactly cash out to recruitment.
Large-scale forecasting tournaments as a talent training and vetting pipeline
In addition to recruitment benefits of forecasting, large-scale public forecasting tournaments can be a great way to both quickly vet and train forecasters. This is most directly useful if you think short-range forecasting is very valuable in itself (for example top forecasters in such tournaments can be funneled towards the EA forecasting hedge fund I mentioned above).
It might also be helpful for training and vetting other skills. A hypothesis that many people have and I somewhat share is that great short-range forecasters are more likely to be great at other things, for example long-range forecasting, evaluative research or grantmaking.
The dream: high-quality, calibrated, long-range forecasting
If we want to improve the long-term future, having really high-quality long-range forecasting is clearly useful for making us much better at prioritizing what things to work on, as well as how to do them (this is not trivially true, cf. falling problem, but it would be surprising to me if very good long-range forecasting is not helpful or only minorly helpful for improving the long-term future).
However, it is unclear to me:
- how we can get much better at long-range forecasting,
- how we can efficiently tell if our methods are helping us get much better at long-range forecasting, and
- whether the ways of getting better at long-range forecasting looks anything like existing forecasting methods (as opposed to, e.g., having much deeper inside-view models, or something else entirely).
I also don’t have strong or novel opinions about how to get great at long-range forecasting. So all other sections in my post is mostly agnostic to long-range forecasting while arguing the “short-range forecasting is great for longtermism” thesis.
Forecasting as a general epistemics intervention
Finally, another reason you might be excited about forecasting is wanting to introduce broad, mass-appeal forecasting as a general epistemics intervention, that is, improving the thinking and reasoning quality of society at large. I don’t currently have well-formed opinions about how tractable this or or how important this is (including whether it’s even net-positive).
One possible non-trivial/contentious point I want to make here is that improving general epistemics about the future is not obviously good. If we use the benevolence, intelligence, power framework of assessing actors, then we want to improve the intelligence of the public insofar as the public’s goals are sufficiently benevolent that making them smarter is better than the alternative. In a bit more detail, a specific worry I have follows from noticing that the current altruistic actors (e.g. people concerned about existential risk) seem to also be unusually consequentialist and concerned with how to improve their forecasting skills. To the extent that it’s easier to improve forecasting at the middle and lower ends than peak forecasting abilities, general epistemics interventions may cause altruistic actors to lose some of our epistemic edge over the rest of the world, and this is likely bad. Concretely, I’m maybe [1]~60% that general epistemics interventions are net positive[2], whereas the tone of many people I talk to seems intuitively closer to ~80-90%.
The list of potentially great uses of forecasting to improve longtermist efforts is overlapping and non-exhaustive. You can help by expanding it.
Next Steps
Further work here (from myself and others) should elucidate and prioritize specific next steps that will be valuable to take, including recommending grants, directions for further research, useful skills and learning value, and (especially) identifying and incubating starter projects to move towards this vision.
There are a number of other groups in this space that I’m excited about, including new projects, many of whom I expect to have launch posts before the middle of this year.
I am also excited about further work by other people in this space and adjacent spaces, including from entrepreneurs, forecasters, forecasting researchers, and grantmakers interested in setting up something like one of the models I proposed above, or being an early employee of them. There are also a number of other exciting ways to contribute. The one I’d like to highlight is for decisionmakers and researchers to consider how expert forecasting can be most helpful for their own work.
Acknowledgements
Many of the ideas in this document have floated around the nascent EA forecasting community for some time, and are not original to me. Unfortunately I have not tracked well which ideas are original, so maybe it’s safe to assume that none of the ideas are. At any rate, I consider the primary value of the current work as a distillation. Thanks to Peter Wildeford, Michael Aird, Ben Snodin, Max Raüker, Misha Yagudin, and Eli Lifland for feedback on earlier drafts. Most mistakes are my own.
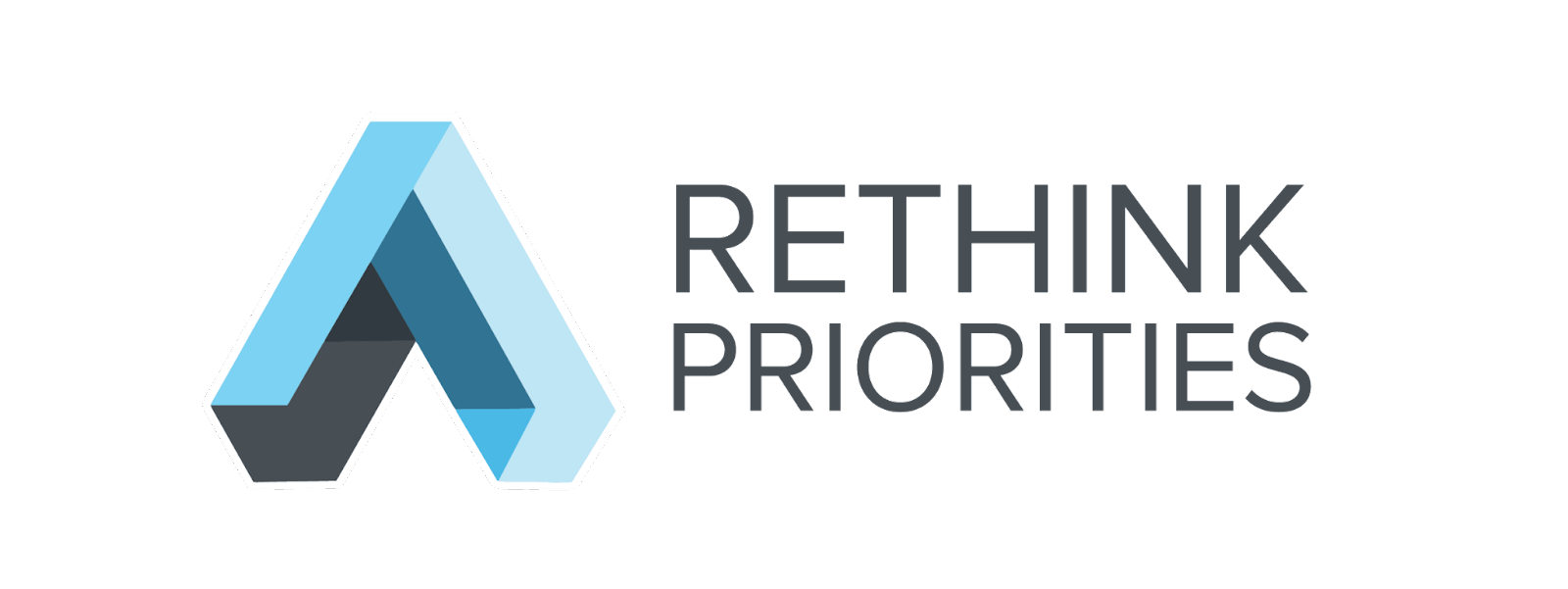
This research is a project of Rethink Priorities. It was written by Linch Zhang. If you like our work, please consider subscribing to our newsletter. You can explore our completed public work here.
- ^
Probability defined in the sense that I’d land on this position upon ~1000 hours of thought, without learning additional empirical facts about the world that could not be easily accessible for someone with unlimited internet access in early 2022.
- ^
As a reference point, I’m only ~35% that marginal scientific acceleration is net positive, and probably a bit lower for bio specifically and a bit higher for AI.
Linch @ 2022-03-18T20:36 (+6)
In the interest of dogfooding my own ideas, I think it'd be cool to amplify this research via forecasting. To that end, I'm paying an external forecasting consultancy, Sage, to come up with a list of operationalized forecasting questions for important cruxes within this research post.
MaxRa @ 2022-03-14T20:44 (+4)
general epistemics interventions may cause altruistic actors to lose some of our epistemic edge over the rest of the world, and this is likely bad
Interesting. Besides the broad altruism of the public it probably also depends on
- how much one expects to having to coordinate with other actors/institutions to reduce x-risks
- how much you actually need especially altruistic values to be aligned on issues like reducing x-risk from AI & pandemics
- how much "epistemic conscientiousness" / the desire to improve forecasting skills correlates with trustworthiness
I'm probably more like 80-90% that this generally is net positive.
Linch @ 2022-03-14T21:13 (+4)
Some quick thoughts:
- The suits who hear forecasts that AGI (or other stuff) is powerful and doom-inducing might just hear that it's POWERFUL and doom-inducing whereas the message we really want to get across (to the extent we want to get messages across at all) is that it's powerful and DOOM-INDUCING.
- Altruistic actors may be more inclined to steer the world towards some plausible conceptions of utopia. In contrast, even if we avert doom, less altruistic actors might still overall be inclined to preserve existing hierarchies and stuff, which could be many orders of magnitude away from optimality.
Also happy to chat further in person.
dilhanperera @ 2022-04-26T13:03 (+1)
Exciting stuff, thanks for the post!
If possible, could you expand on this bit from idea 2? "Note that I think existing prediction and evaluation setups are currently not ready to do this well. Among others, we need a) better engineering setups to do forecasting at scale, and b) better ontologies for cleaner evaluations at scale"
In particular, what do you see as the scale-limiting characteristics of platforms like Metaculus? Lack of incentives, or something else?
And what do you mean by "better ontologies for cleaner evaluations"? (E.g. describing an existing ontology and its limitations would be helpful)
Thanks!
David Johnston @ 2022-03-15T01:32 (+1)
Are you at ~65% that marginal scientific acceleration is net negative, or is most of your weight on costs = benefits?
Linch @ 2022-03-15T01:38 (+3)
~65% that marginal scientific acceleration is net negative