The Importance of AI Alignment, explained in 5 points
By Daniel_Eth @ 2023-02-11T02:56 (+50)
This piece gives an overview of the alignment problem and makes the case for AI alignment research. It is crafted both to be broadly accessible to those without much background knowledge (not assuming any previous knowledge of AI alignment or much knowledge of AI) and to make the compositional logic behind the case very clear.
I expect the piece will be particularly appealing to those who are more reductive in their thinking and who want to better understand the arguments behind AI alignment (I’m imagining this audience includes people within the community doing field building, as well as people in various STEM fields who have only vaguely heard of AI alignment).
Crossposted to the AGI Safety Fundamentals website, with minor edits:
https://www.agisafetyfundamentals.com/alignment-introduction
This piece describes the basic case for AI alignment research, which is research that aims to ensure that advanced AI systems can be controlled or guided towards the intended goals of their designers. Without such work, advanced AI systems could potentially act in ways that are severely at odds with their designers’ intended goals. Such a situation could have serious consequences, plausibly even causing an existential catastrophe. In this piece, I elaborate on five key points to make the case for AI alignment research:
- Advanced AI (defined below) is possible
- Advanced AI might not be that far away (possibly realized within decades)
- Advanced AI might be difficult to direct
- Poorly-directed advanced AI could be catastrophic for humanity
- There are steps we can take now to reduce the danger
1 – Advanced AI is possible
By advanced AI, I mean, roughly speaking, AI systems capable of performing almost all cognitive work humans perform (e.g., able to substitute for scientists, CEOs, novelists, and so on).
Researchers disagree about the form of advanced AI that is most likely to be developed. Many of the most popular visions involve an “artificial general intelligence,” or “AGI” – a hypothetical AI system that could learn any cognitive task that a human can.[1] One possibility for advanced AI is a singular AGI that could outcompete human experts in most fields across their areas of expertise. Another possibility is an ecosystem of specialized AI systems that could collectively accomplish almost all cognitive work – some researchers speculate that this setup would involve multiple AGIs performing complex tasks alongside more narrow AI systems.[2] Researchers also disagree about whether advanced AI will more likely be developed using current AI methods like deep learning or via a future AI paradigm that has not been discovered yet.[3]
There is approximate consensus among almost all relevant experts, however, that advanced AI is at the very least physically possible. The human brain is itself an information-processing machine with the relevant capabilities, and thus it serves as proof that machines with such capabilities are possible; an AI system capable of the same cognitive tasks as the human brain would, by definition, be advanced AI.[4]
2 – Advanced AI might not be that far away
Below, I outline some reasons for thinking advanced AI might be achieved within the next few decades. Each argument has its limitations, and there is a lot of uncertainty; nevertheless, considering these arguments collectively, it seems appropriate to put at least decent odds (e.g., double-digit percent chance) on advanced AI becoming a reality within a few decades.
Many AI experts and generalist forecasters think it’s likely advanced AI will be developed within the next few decades:
- A 2016 survey of top AI researchers found that most forecasted at least a 50% chance of “high-level machine intelligence” (defined similarly to advanced AI) being developed by 2061, with around a 30% chance of development by 2040. Follow-up surveys in 2019 and 2022 found similar results.
- According to the aggregated predictions of forecasters on the prominent forecasting-website Metaculus, there is a 50% chance of AGI arriving by 2042.[5] Metaculus is well-regarded among many forecasters for its track record; also, particularly relevant to advanced AI, Metaculus has previously been ahead of the curve in predicting major world events[6] and technological progress.[7]
Extrapolating AI capabilities plausibly suggests advanced AI within a few decades:
- Qualitatively, AI in the past several years has made large strides imitating both intuitive thinking (e.g., computer vision, creating images from written descriptions, robotic gymnastics) and high-level reasoning (e.g., multi-step reasoning, explaining jokes, solving high school- and college-level math and science problems).[8] These two hallmarks of human thought were, until recently, largely believed to be far out of reach for AI and harbingers of advanced AI.
- Crucially, the current wave of AI progress is largely fueled by AI systems that learn relatively broad capabilities from scratch, with performance continuously improving as computing power increases, and novel capabilities sometimes emerging. For example, OpenAI’s GPT-3 was programmed to simply learn to predict the next word from a sequence of text,[9] and it surprisingly learned capabilities as varied as poetry, language translation, and computer coding.
- Based on how AI performance scales with computing power for current AI systems, we can estimate how much more computing power would be necessary for these systems to reach human performance on various tasks. Further, given trends in the cost of computing power over time, we can estimate when this level of computing power may become economically available. Performing this calculation with the AI systems of GPT-3 and PaLM, and judging by these systems’ performance on reasoning tasks they were not specifically trained for (e.g., analogies, common sense, arithmetic), approximately 25-50 years of additional growth in computing power would be enough for these systems to achieve human-equivalent performance on most such reasoning tasks, even assuming zero algorithmic improvements.[10]
- Speculatively, comparisons between AI and the human brain tend to find that the hardware needed to train an AI to match the brain will likely be available within a few decades.[11]
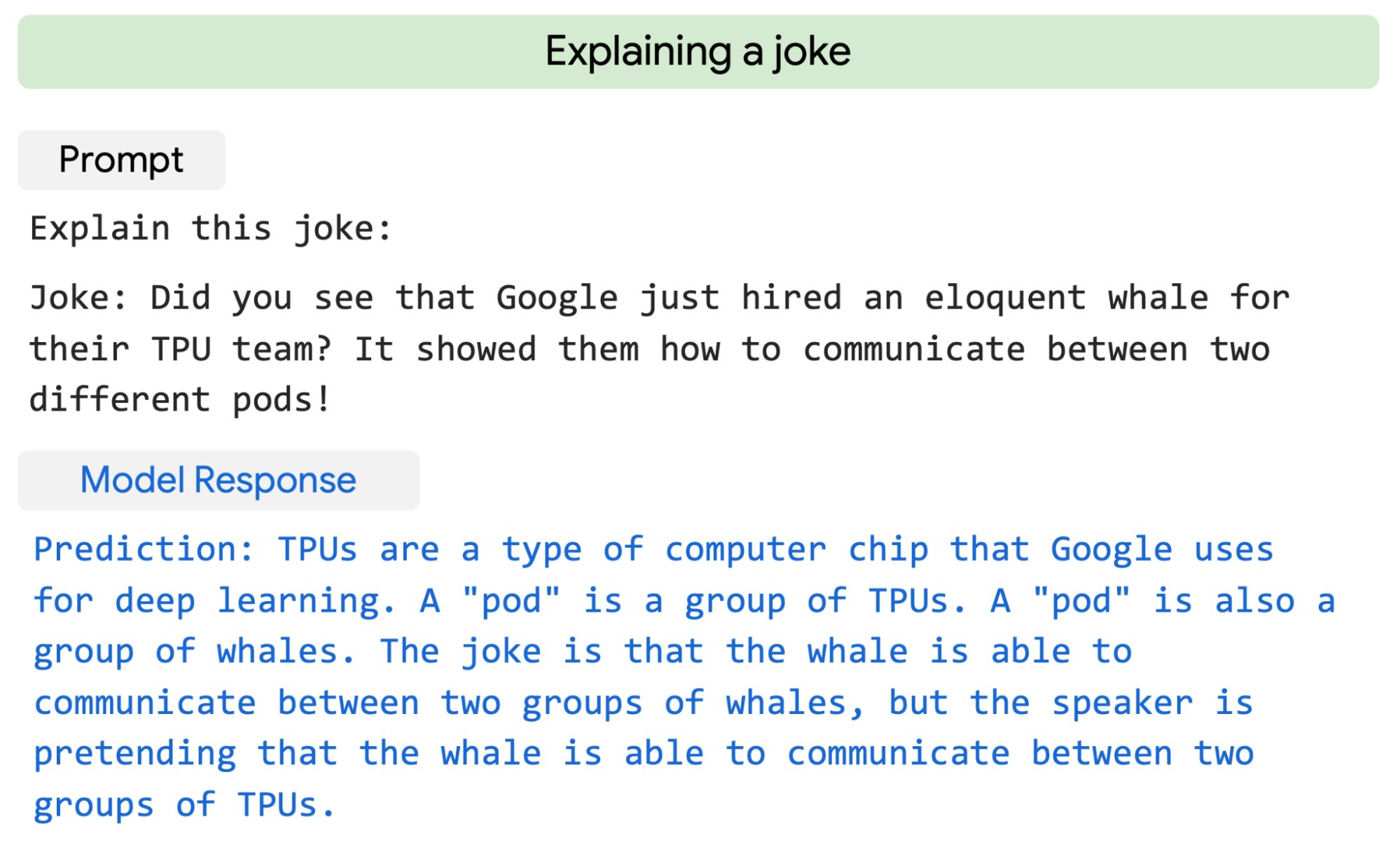
3 – Advanced AI might be difficult to direct
Current AI systems are often accidentally misdirected:
- GPT-3, for instance, is notorious for outputting text that is impressive, but not of the desired “flavor” (e.g., outputting silly text when serious text is desired), and researchers often have to tinker with inputs considerably to yield desirable outputs.
- There are many examples of various AI systems achieving the exact goals they were designed to achieve, but in surprising ways that violate a commonsense, humanlike understanding.[12] In one comical example, an AI trained to maximize points in a boat-racing video game settled on a strategy of ignoring the track and instead spinning in circles to continuously pick up the same bonuses, all the while crashing into surrounding boats.
Advanced AI systems may similarly technically satisfy specifications in ways that violate what we actually want (i.e., the “King Midas” problem):
- One allure of advanced AI systems is they could act in open-ended ways (e.g., taking on the roles of CEOs); however, as such systems may inevitably confront a multitude of unforeseen trade-offs, it may be nearly impossible to specify ahead of time desired goals for these systems to pursue.[13]
- Goals that initially work well can spell disaster when taken to the extreme, but problems may not be immediately obvious.[14]
- Aligning advanced AI based on fixed goals (i.e., with hard-coded reward functions) may be intractable, as no matter how complex you made the goals, you’d leave out some important features and have difficulty explicitly defining other “fuzzy” features.
Training AI on human feedback may help address the above specification problems, but this introduces its own problems, including incentivizing misleading behavior:
- In response to the above concerns, most researchers think we should train advanced AI on human feedback instead of simply on fixed goals; analogously, a boat-racing AI might not just be rewarded for collecting points, but also penalized for behavior that a human overseer subjectively decides is bad, in the hopes that the AI would adopt behavior that aligned with the hard-to-specify desires of the human.
- Unfortunately, human feedback is an imperfect indicator of what we really want, and the gap between the two may still be exploitable by the AI; this gap may be particularly large if the volume or speed of AI decision-making is such that human oversight can only be sporadic, if the AI proposes actions beyond the comprehension of the human, or if the human is simply mistaken about relevant factual matters.
- Worse, this setup creates an incentive for the AI to mislead its human overseer into thinking it’s better behaved than it is. As an overly simplistic example, consider an overseer that attempts to train a cleaning robot by providing periodic feedback to the robot, based on how quickly the robot appears to clean a room; such a robot might learn that it can more quickly “clean” the room by instead sweeping messes under a rug.[15]
- Despite these limitations, training AI systems on human feedback remains a promising research avenue for AI alignment, even if it may not solve the entire problem itself (see section 5 for more on areas of AI alignment research).
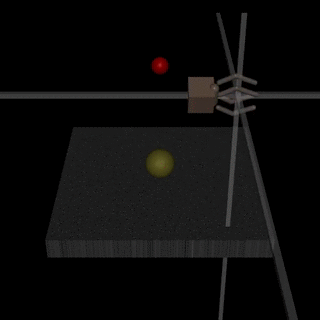
Additionally, advanced AI systems may come to pursue proxies for goals that work well in training, but these proxies may break down during deployment:
- Modern AI systems are typically trained to “learn” behaviors via a trial-and-error-like process in which they are “rewarded” for scoring well according to some goal (specified by their designers). This process often leads to the AI pursuing proxies for the specified goal; while these proxies may work well in training, they often stop working well when the environment changes (such as in deployment). Advanced AI may be trained similarly and thus may have similar problems.
- Notably, some AI systems, when faced with a novel environment, can retain their previous capabilities yet pursue a different goal than the one that was specified by their designers.[16] In one example, an AI was trained to solve (virtual) mazes, where winning involved reaching a piece of cheese towards the upper-right corner of the maze; when the AI was tested on mazes where the cheese was positioned elsewhere, the AI would often ignore the cheese and progress toward the upper-right corner nonetheless – competently pursuing a different goal than what the designers had specified.
- By analogy, evolution “trained” humans to (approximately[17]) maximize survival and reproduction, but this led to human traits that, in today’s world, no longer promote survival and reproduction. For instance, evolution gave humans a desire for various flavors of food, in order to prod humans to eat various types of nutritious food in the ancestral environment; more recently, however, humans have invented many artificial flavors that often satisfy these desires to greater degrees than their natural counterparts, often without the evolutionarily-advantageous nutritional value. We don’t want AI systems that similarly skirt the goals we give them (“pursuing the equivalent of artificial flavors”).[18]
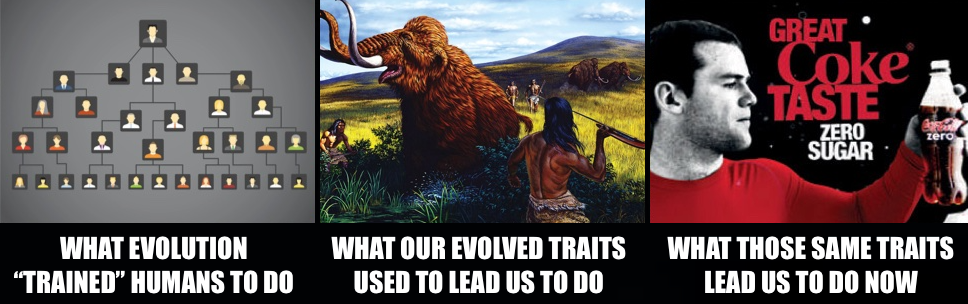
To be clear, the above worries don’t imply that advanced AI wouldn’t be able to “understand” what we really wanted, but instead that this understanding wouldn’t necessarily translate to the AI systems acting in accordance with our wants:
- By definition, advanced AI would be able to perform tasks that require understanding “fuzzy” aspects of human goals and behavior,[19] and thus such AI would likely “understand”[20] ways in which the goals it internalized from training conflicted with the goals its designers intended (e.g., it may recognize that its goals were actually just imperfect proxies for its designers’ goals).
- If an AI recognizes this discrepancy between its internalized goals and its designers’ intended goals, however, that does not automatically cause the discrepancy to disappear. Continuing the evolution analogy, when a human learns that their love of artificially-flavored food is due to evolutionary pressure for nutritious food, the human doesn’t suddenly start desiring nutritious food instead; contrarily, the human typically continues to desire the same artificially-flavored food as before.
- Once the AI system can understand the discrepancy, however, it may face incentives to hide the discrepancy from its human overseer (i.e., it might “play nice” for the rest of training and do harmful things only when deployed in the real world). This deceptive behavior may happen if, for instance, the AI has goals related to affecting the world in ways the overseer would disapprove of.[21]
- Speculatively, we might thus wind up in a bit of a catch-22: before the AI system has been trained sufficiently to understand the discrepancy, it cannot be trained to automatically bring all its goals into alignment with its overseer’s desires, and once it has reached this understanding, it will face an incentive to deceive its overseer instead.
- If we could simply tell the AI “do what we mean, not what we say” and get the AI to robustly listen to that, we would have solved this problem, but no one knows how this can be accomplished given current research.
It’s possible advanced AI will be built before we solve the above problems, or even without anyone really understanding the systems that are built:
- While no one currently knows how to build advanced AI, there is no strong reason to assume we’ll solve the above problems before we get there.
- Current AI systems are typically “black boxes,” meaning that their designers don’t understand their inner workings. Emergent, learned capabilities occasionally remain undiscovered for significant periods. If the current AI paradigm leads to advanced AI, these advanced systems will likely similarly be black boxes.
- History is filled with examples of technologies that were created before a good understanding of them was developed; for instance, humans built bridges for millennia before developing mechanical engineering (note many such bridges collapsed, in now-predictable ways), flight was developed before much aerodynamic theory, and so on.
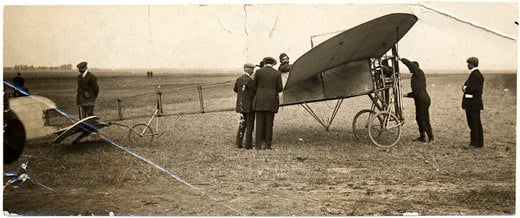
4 – Poorly-directed advanced AI could be catastrophic for humanity
Our typical playbook regarding new technologies is to deploy them before tackling all potential major issues, then course correct them over time, solving problems after they crop up. For instance, modern seatbelts were not invented until 1951, 43 years after the model T Ford’s introduction; consumer gasoline contained the neurotoxin lead for decades, before being phased out; etc.
With advanced AI, on the other hand, relatively early failures at appropriately directing these systems may preclude later course correction, possibly yielding catastrophe. This dynamic necessitates flipping the typical script – anticipating and solving problems sufficiently far ahead of time, so that our ability as humans to course correct is never extinguished.
As mentioned above, poorly-directed advanced AI systems may curtail humanity’s ability to course correct:
- Soon after we develop advanced AI, we will likely face AI systems that far surpass humans in most cognitive tasks,[22] including in tasks relevant for influencing the world (such as technological development, social/political persuasion, and cyber operations).
- Insofar as advanced AI systems and humans pursue conflicting goals, advanced AI will likely outcompete or outmaneuver humans to achieve their goals above ours.[23]
- Poorly-directed advanced AI systems would likely determine (correctly) that their then-current goals would not be achieved if humans redirected them towards other goals or shut them off, and thus would (successfully) take steps to prevent these interventions.[24]
From there, the world could develop in unexpected and undesirable ways, with no recourse:
- Humans in such a world dominated by advanced AI may be as vulnerable as many animals in today’s (human-dominated) world, where our fate would depend more on the goals of advanced AI systems than on our own goals.[25]
- This situation could take many forms: a single AI could become more powerful than the rest of civilization combined; a group of AIs could coordinate to become more powerful than the rest of civilization; an ecosystem of different (groups of) AIs may wind up in a balance of power with each other, but with humans effectively out of the loop of society’s decisions; etc.
- It’s unclear if such worlds would even preserve features necessary for human survival – as a thought experiment, would a fully-automated and growing AI economy (with various AI systems psychopathically pursuing various goals) ensure food was provided for humans, or that the byproducts of industrial processes never altered the atmosphere beyond ranges survivable to humans? Maybe?
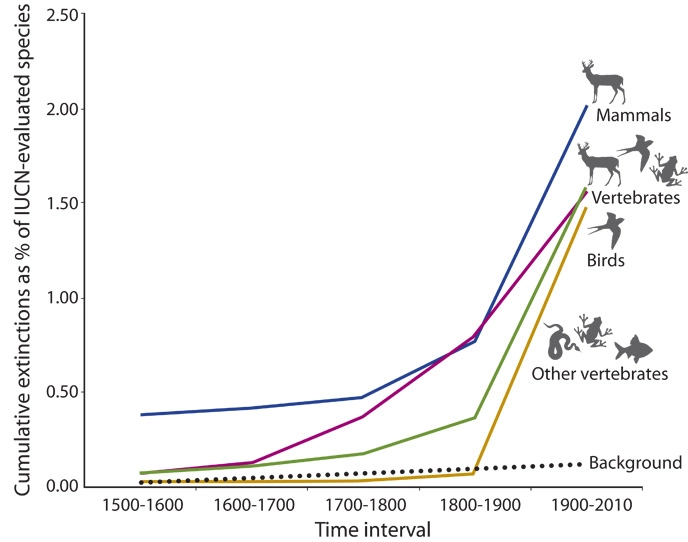
While the above worries may sound extreme, they are not particularly fringe among relevant experts who have examined the issue (though there is considerable disagreement among experts and not all share these concerns):
- Some leading AI researchers have publicly voiced these concerns (e.g., UC Berkeley CS professor Stuart Russell), as did a few early CS and AI pioneers (e.g., Alan Turing).
- In a recent survey of top AI researchers, when asked explicitly, “What probability do you put on future AI advances causing human extinction or similarly permanent and severe disempowerment of the human species?” the majority estimated at least a 1 in 20 chance of such an outcome. In two previous surveys, most top AI researchers placed at least 1 in 20 odds and at least 1 in 50 odds on “high-level machine intelligence” (defined similar to advanced AI) having an impact that is “extremely bad (e.g., human extinction).”
- Some leading AI labs, such as DeepMind (which is owned by Google) and OpenAI (which is heavily funded by Microsoft), acknowledge these risks are serious, arguably against their vested interests.
- Researchers in the field of existential risk think advanced AI risks are serious.[26]
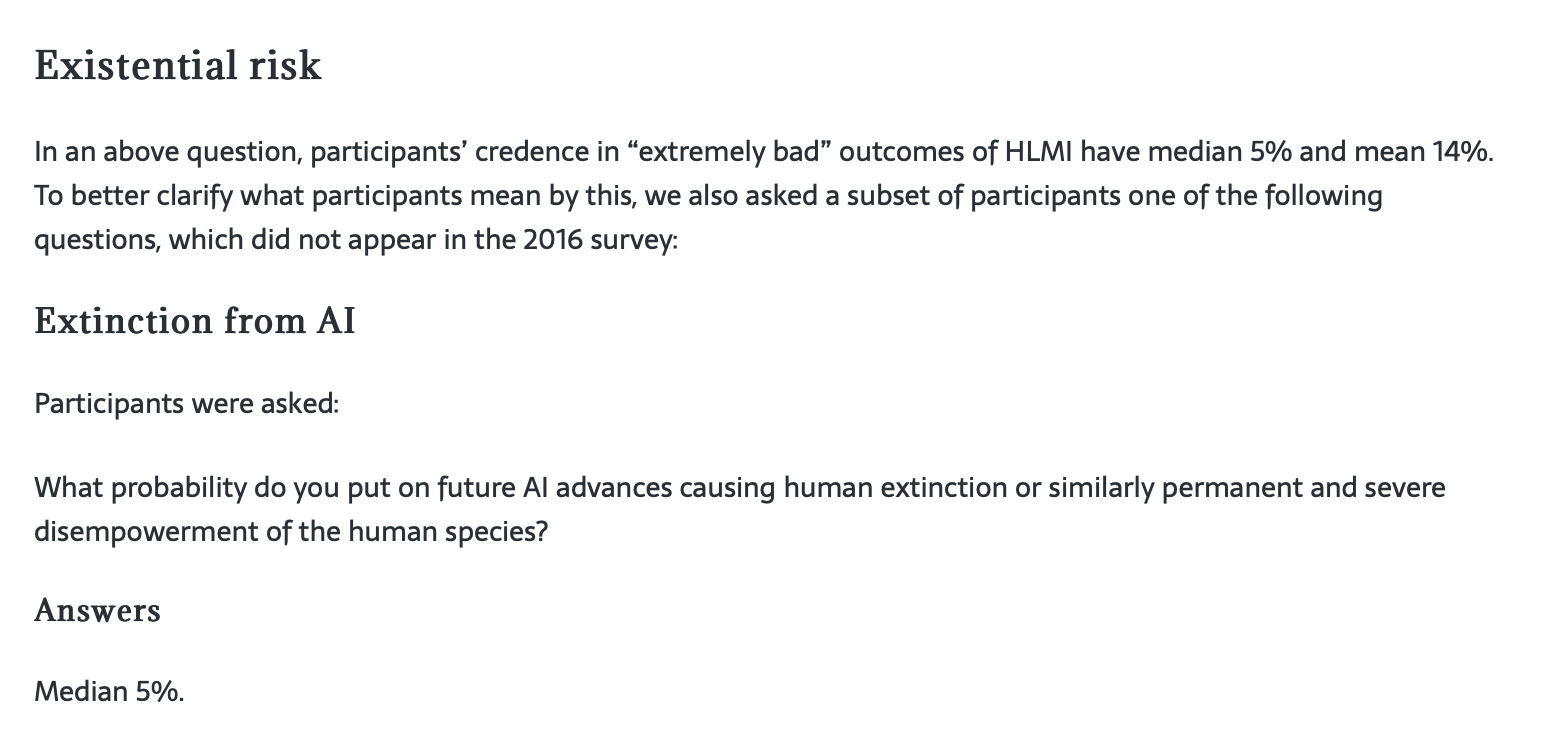
5 – There are steps we can take now to reduce the danger
To reduce the risks discussed above, two broad types of work are being pursued – developing technical solutions that enable advanced AI to be directed as its designers intend (i.e., technical AI alignment research) and other, nontechnical work geared towards ensuring these technical solutions are developed and implemented where necessary (this nontechnical work falls under the larger umbrella of AI governance[27]).
Some technical AI alignment research involves working with current AI systems to direct them towards desired goals, with the hope that insights transfer to advanced AI:
- Advanced AI systems might resemble current AI systems to some degree, so methods for directing current AI systems may yield valuable insights that transfer over to advanced AI.
- Research intuitions sometimes transfer between engineering paradigms, so even if advanced AI does not resemble current AI, intuitions gained from directing current AI may still be valuable for directing advanced AI.
Other technical AI alignment research involves more theoretical or abstract work:
- This research often abstracts away the specifics of how advanced AI may work and instead considers how idealized AI systems with traits such as goal-directedness, embeddedness in their environment, and high optimization power may be formulated so that they could be directed according to the (hard to specify) wishes of their (future) designers.
- These sorts of theoretical abstractions allow for research relevant to AI systems with capabilities far beyond those available today or that operate according to unfamiliar processes.
The next two paragraphs list two broad areas of technical AI alignment research – note that I’m listing these areas simply for illustrative purposes, and there are many more areas that I don’t list.
Understanding the inner workings of current black-box AI systems:
- Better understanding may enable both designing AI in more intentional ways and checking (before deployment) if systems possess dangerous emergent capabilities.
- Further, good understanding of the internal workings of AI systems may allow for training AI not just based on outward behavior, but also on inner workings, potentially allowing for more easily directing AI systems to adopt or avoid particular internal procedures (e.g., it may be possible to train AI to not be deceptive via feedback on the AI’s internal workings[28]).
- Mechanistic interpretability research involves developing methods to understand inner workings of otherwise black-box AI systems (example).
- Deep Learning theory research involves investigating why current AI systems develop in the sorts of ways they do, as well as describing underlying dynamics (example).
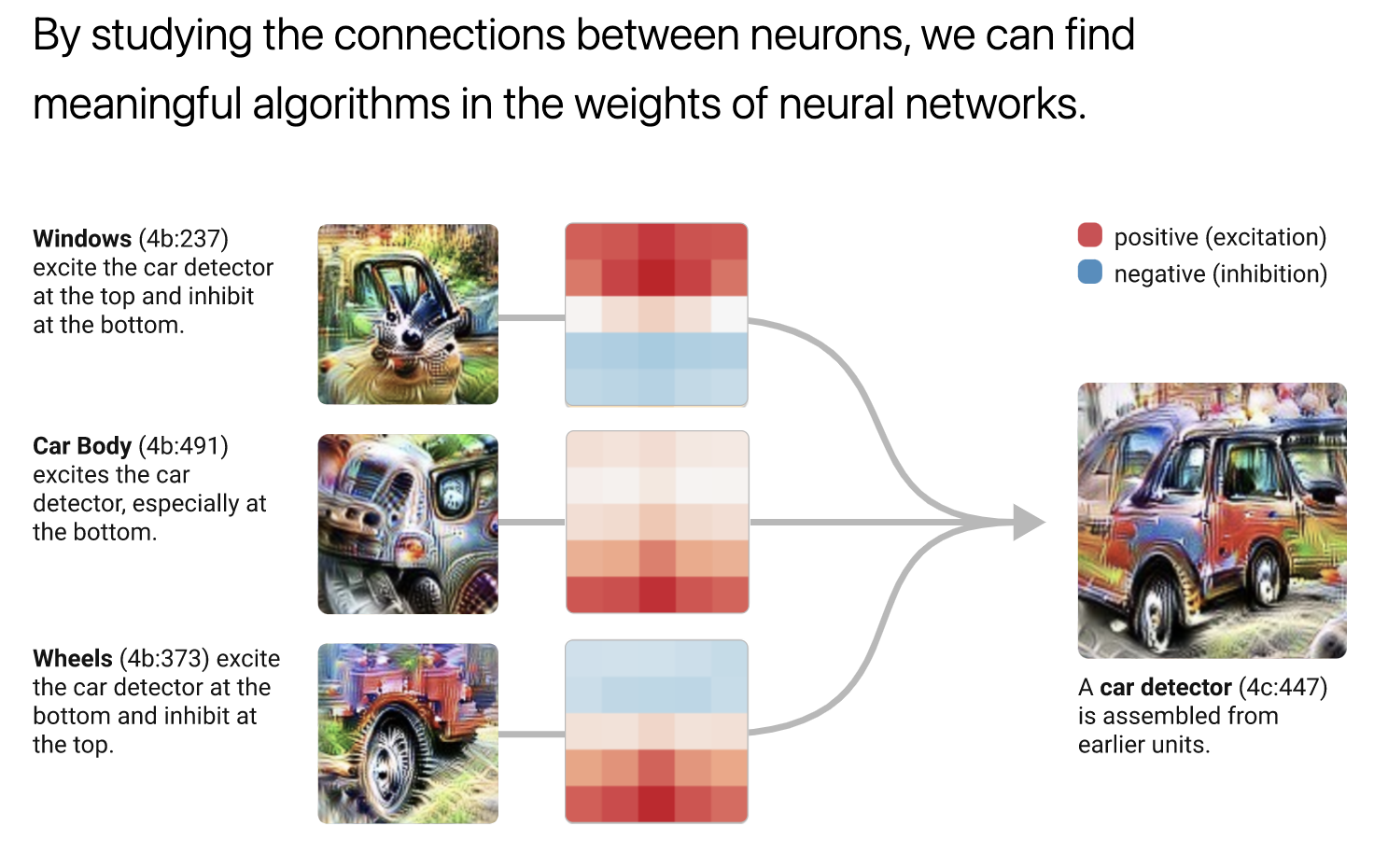
Developing methods for ensuring the honesty or truthfulness of AI systems:
- Many cutting-edge AI systems specializing in language generation are prone to making factually inaccurate statements, sometimes despite the exact same system previously having made a true statement on the same exact factual matter (e.g., the system may answer a factual question inaccurately, despite previously having answered the same question accurately).[29]
- Research into truthful AI aims for AI systems that avoid making such false claims (example), while research in the related field of honest AI seeks AI systems that make claims in line with their learned models of the world (example).[30]
- Work to direct AI systems to only ever be “honest” or “truthful” may function as practice for later work directing advanced AI toward other important-yet-“fuzzy” goals.
- Additionally, if we could direct advanced AI to be honest, that alone may reduce risks related to deception, as then the system could not pretend to lack knowledge that it had, nor could it necessarily develop strategic plans hidden from human oversight.
- In addition to empirical work on directing current AI systems to be honest/truthful, researchers are pursuing theoretical work on methods to elicit latent knowledge from advanced AI – that is, to read off “knowledge” that the AI has, thereby effectively forcing it to be honest.
See more: the online AI Alignment Curriculum from the AGI Safety Fundamentals program describes several further technical AI alignment research avenues in more detail, as does the paper Unsolved Problems in ML Safety.[31]
On the nontechnical side, several areas of AI governance are relevant for reducing misalignment risks from advanced AI, including work to:
- Reduce risks of corner-cutting on the development of advanced AI – if advanced AI is constructed in a hurried manner or without proper safety measures, it may be more likely to wind up poorly directed. Worryingly, most software is currently developed in a relatively haphazard way, and the field of AI does not have a particularly strong culture of safety the way some disciplines, like nuclear engineering, do. Some current work to reduce this risk is geared towards reducing a zero-sum “race dynamic” towards advanced AI.[32]
- Improve institutional decision-making processes (especially on emerging technology) – plausible reforms to improve societal decision-making are obviously too numerous and varied to mention, but broadly speaking, better governmental, international, and corporate decision-making may yield more sensible actions to promote aligned AI systems in the run up to advanced AI.
See more: the AI Governance Curriculum from the AGI Safety Fundamentals program describes further areas of AI governance work in more detail.
Note that technical problems can sometimes take decades to solve, so even if advanced AI is decades away, it’s still reasonable to begin working on developing solutions now. Current technical AI alignment work is occurring in academic labs (e.g., at UC Berkeley's CHAI, among many other academic labs), in nonprofits and public benefit corporations (e.g., Redwood Research and Anthropic), and in industrial labs (e.g., DeepMind and OpenAI). A recent survey of top AI researchers, however, indicates most (69%) think society should prioritize “AI safety research”[33] either “more” or “much more” than currently.
- ^
It should be noted that some researchers view the concept of “general intelligence” as flawed and consider the term “AGI” to be either a misnomer at best or confused at worst. Nevertheless, in this piece we are concerned with the capabilities of AI systems, not whether such systems should be referred to as “generally intelligent,” so disagreement over the coherency of the term “AGI” doesn’t affect the arguments in this piece.
- ^
In this second scenario, different AGIs might specialize in a similar manner to how human workers specialize in the economy today.
- ^
A future paradigm could, for instance, be based on future discoveries in neuroscience.
- ^
The brain is a physical object, and its mechanisms of operation must therefore obey the laws of physics. In theory, these mechanisms could be described in a manner that a computer could replicate.
- ^
As of today's date: February 10, 2023.
- ^
- ^
E.g., Metaculus predicted a breakthrough in the computational biology technique of protein structure prediction, before DeepMind’s AI AlphaFold astounded scientists with its performance in this task.
- ^
Other examples where AI has recently made large strides include: conversing with humans via text, speech recognition, speech synthesis, music generation, language translation, driving vehicles, summarizing books, answering high school- or college-level essay questions, creative storytelling, writing computer code, scientific advancement, mathematical advancement, hardware advancement, mastering classic board games and video games, mastering multiplayer strategy games, doing any one task from a large number of unrelated tasks and switching flexibly between these tasks based on context, using robotics to interact with the world in a flexible manner, integrating cognitive subsystems via an “inner monologue,” etc.
- ^
Technically, this description is a slight simplification; GPT-3 was actually programmed to learn to predict the next “token” from a sequence of text, where a “token” would generally correspond to either a word or a portion of a word.
- ^
Depending on whether we extrapolate linearly or using an “S-curve,” most such tasks are implied to reach near-perfect performance with ~1028 to ~1031 computer operations of training. Assuming a $100M project, an extrapolation of 2.5 year doubling time in the price-performance of GPUs (computer chips commonly used in AI), and a current GPU computational cost of ~1017 operations/$, such performance would be expected to be reached in 25 to 50 years. Note this extrapolation is highly uncertain; for instance, high performance on these metrics may not in actuality imply advanced AI (implying this estimate is an underestimate) or algorithmic progress may reduce necessary computing power (implying it’s an overestimate).
- ^
The most powerful supercomputers today likely already have enough computing power to surpass that of the human brain. However, an arguably more important factor is the amount of computing power necessary to train an AI of this size (the amount of computing power necessary to train large AI systems typically far exceeds the computing power necessary to run such systems). One extensive report used a few different angles of attack to estimate the amount of computing power needed to train an AI system that was as powerful as the human brain, and this report concluded that such computing power would likely become economically available within the next few decades (with a median estimate of 2052).
- ^
This problem is known as “specification gaming” or “outer misalignment.”
- ^
E.g., “maximize profits,” if interpreted literally and outside a human lens, may yield all sorts of extreme psychopathic and illegal behavior that would deeply harm others for the most marginal gain in profit.
- ^
The general phenomena at play here (sometimes referred to as “Goodhart’s law”) has many examples – in one classic-but-possibly-fictitious example, the British Empire put a bounty on cobras within colonial India (to try to reduce the cobra population), but some locals responded by breeding cobras to kill in order to collect the bounty, thus eventually leading to a large increase in the cobra population.
- ^
Similarly, attempts to train AI systems to not mislead their overseers (by punishing these systems for behavior that the overseer deems to be misleading) might instead train these systems to simply become better at deception so they don't get caught (for instance, only sweeping a mess under the rug when the overseer isn’t looking).
- ^
This problem is known as “goal misgeneralization” or “inner misalignment.”
- ^
Note the true story is somewhat more complicated, as evolution “trained” individuals to also support the survival and reproduction of their relatives.
- ^
As one simple example, we don’t want a video-game-playing AI to hack into its console to give itself a high score once it learns how to accomplish this feat.
- ^
For instance, understanding what we really mean when we use imprecise language.
- ^
At least insofar as AI can be said to “understand” anything.
- ^
The logic here is the AI may reason that if it defected in training, the overseer would simply provide negative feedback (which would adjust its internal processes) until it stopped defecting. Under such a scenario, the AI would be unlikely to be deployed in the world with its current goals, so it would presumably not achieve these goals. Thus, the AI may choose to instead forgo defecting in training so it might be deployed with its current goals.
- ^
It’s common for cutting-edge AI capabilities to move relatively quickly from matching human abilities in a domain to far surpassing human abilities in that domain (see: chess, Jeopardy!, and Go for high-profile examples). Alternatively, even if it takes a while for advanced AI capabilities to progress to far surpassing human abilities in the relevant domains, the worries sketched out below may still occur in a more drawn-out fashion.
- ^
In the same way that AI systems can now outcompete humans in chess and Go.
- ^
Such AI systems might guard against being shut down by using their social-persuasion or cyber-operation abilities. As just one example, these systems might initially pretend to be aligned with the interests of humans who had the ability to shut them off, while clandestinely hacking into various data centers to distribute copies of themselves across the internet.
- ^
Note that for many animals, the problem is not due to idiosyncrasies of human nature, but instead simply due to human interests steamrolling animal interests where interests collide (e.g., competing for land).
- ^
For instance, Toby Ord, a leading existential risk researcher at Oxford, estimates that “unaligned AI” is by far the most likely source of existential risk over the next 100 years – greater than all other risks combined.
- ^
AI governance also encompasses several other areas. For instance, it includes work geared towards ensuring advanced AI isn’t misused by bad actors who intentionally direct such systems towards undesirable goals. Such misuse may, in an extreme scenario, also constitute an existential risk (if it enables the permanent “locking-in” of an undesirable future order) – note this outcome would be conceptually distinct from the alignment failure modes described in this piece (which, instead of being “intentional misuse” are “accidents”), so such misuse cases are not covered in this piece.
- ^
Feedback on outward behavior may be inadequate for training AI systems away from deception, as if one is being deceptive, then one will generally outwardly behave in a manner designed to not appear deceptive.
- ^
Interestingly, these same systems are reasonably good at evaluating their own previous claims – that is, if they are asked to evaluate how likely a previous claim they made is to be accurate, they tend to give substantially higher probability of accuracy for claims that are in fact accurate compared to those that are inaccurate.
- ^
Honest AI may therefore make false claims if it had learned inaccurate information, but it would not generally make false claims on an issue where it had learned accurate information and assimilated this information into its “knowledge” of the world. (Note that researchers disagree about whether current AI systems should or should not be said to have “knowledge” in the sense that the word is commonly used, even setting aside the thorny issue of precisely defining the word “knowledge.”)
- ^
Note that the latter paper defines alignment research differently than I have – by my definition, most of the research avenues in that paper would be considered technical AI alignment research, even ones the paper does not classify within the section on “alignment.”
- ^
The more that various organizations feel they are in a competitive race towards advanced AI, the more pressure there may be for at least some of these organizations to cut corners to win the race.
- ^
The survey described “AI safety research” as having significant overlap with what I’m calling “technical AI alignment research.”
Raphaël S @ 2023-03-03T07:10 (+3)
Excellent breakdown, thanks
Dušan D. Nešić (Dushan) @ 2023-02-16T17:10 (+2)
If you, the person reading this, are interested in helping with AI safety but do not have a technical background in AI Safety and instead have it in complex sciences (evolutionary biology, sociology, economics, law, neurology...) do reach out to us at PIBBSS.ai . You will be late for this year's applications, but we may have other ways of cooperating, or we can put you on the list to be the first one to find out when we have new programs by signing up to our mailing list.